Imposing equilibrium restrictions in the estimation of dynamic discrete games
QUANTITATIVE ECONOMICS(2021)
摘要
Imposing equilibrium restrictions provides substantial gains in the estimation of dynamic discrete games. Estimation algorithms imposing these restrictions have different merits and limitations. Algorithms that guarantee local convergence typically require the approximation of high-dimensional Jacobians. Alternatively, the Nested Pseudo-Likelihood (NPL) algorithm is a fixed-point iterative procedure, which avoids the computation of these matrices, but-in games-may fail to converge to the consistent NPL estimator. In order to better capture the effect of iterating the NPL algorithm in finite samples, we study the asymptotic properties of this algorithm for data generating processes that are in a neighborhood of the NPL fixed-point stability threshold. We find that there are always samples for which the algorithm fails to converge, and this introduces a selection bias. We also propose a spectral algorithm to compute the NPL estimator. This algorithm satisfies local convergence and avoids the approximation of Jacobian matrices. We present simulation evidence and an empirical application illustrating our theoretical results and the good properties of the spectral algorithm.
更多查看译文
关键词
Dynamic discrete games, nested pseudo-likelihood, fixed-point algorithms, spectral algorithms, convergence, convergence selection bias, C13, C57, C61, C73
AI 理解论文
溯源树
样例
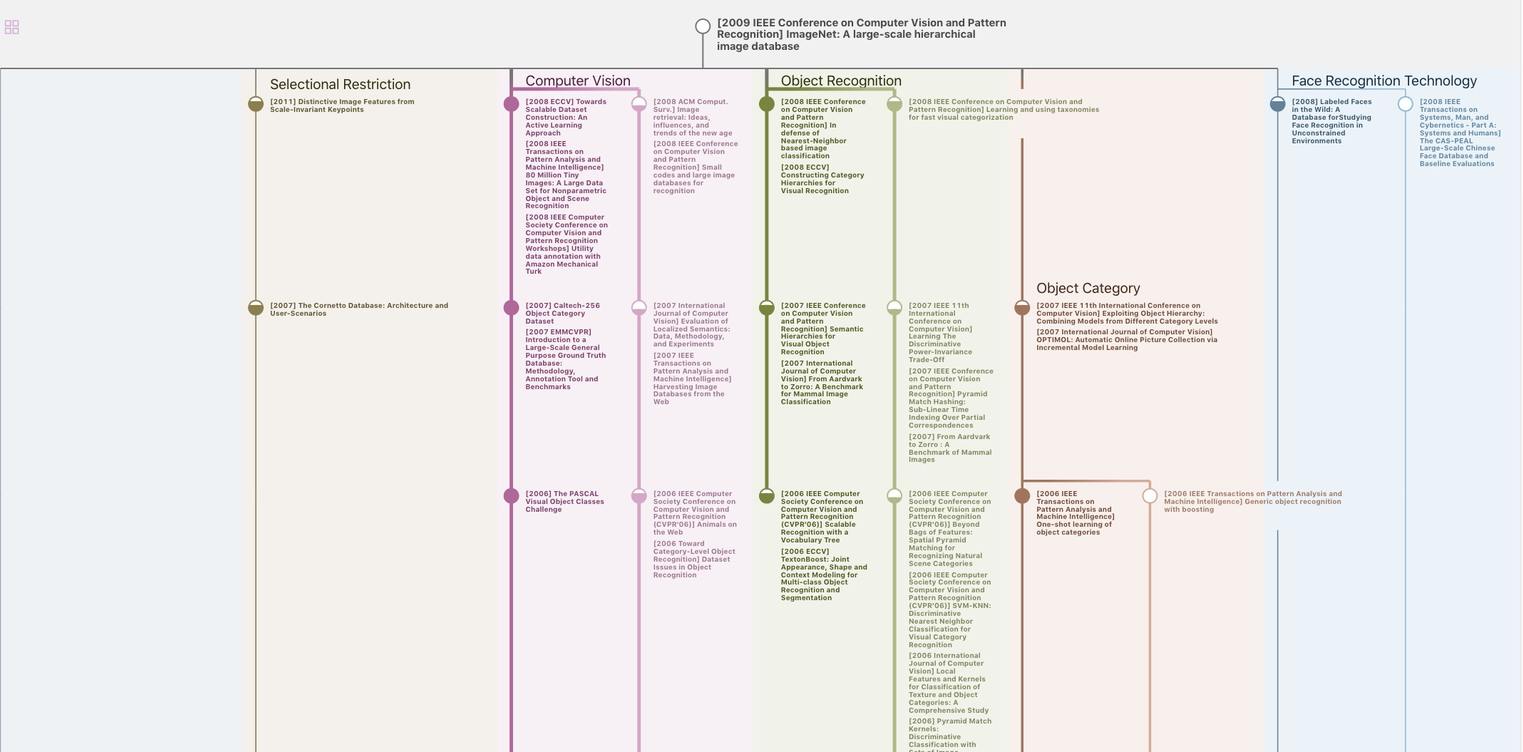
生成溯源树,研究论文发展脉络
Chat Paper
正在生成论文摘要