Quantification of Parkinsonian Kinematic Patterns in Body-Segment Regions During Locomotion
Journal of Medical and Biological Engineering(2022)
摘要
Purpose Diagnosis and treatment of Parkinson’s Disease (PD) are typically supported by a kinematic gait analysis. Nonetheless, the main drawbacks of the classical analysis, based on a reduced set of markers, are the loss of small dynamical changes, the invasive methodology, and the sparse representation from few points, restricting the disease analysis. This work aims to perform a robust regional kinematic characterization, which may result in a potential digital biomarker of the disease to complement personalized analysis, treatment and monitoring of PD. Methods This work introduces a markerless computational framework based on a full body-segment kinematic characterization related with PD motor alterations. Firstly, a set of dense motion trajectories are computed to represent locomotion. Such trajectories are grouped using a deep learning based body segmentation, that partitions the human silhouette into regions corresponding to the head, trunk and limbs. Each resultant region is described using dartboard -like kinematic histograms computed along the trajectories. Results The proposed approach was validated using different pretrained classification models. The proposed method was evaluated on a set of 11 control subjects and 11 PD patients, achieving an average accuracy of 99.62% for lower-limbs and head regions. Conclusion This work proved to be effective to classify Parkinsonian patterns w.r.t control gaits. A major contribution of the proposed strategy is the capability to recover kinematic patterns in different body segments, particularly, for head and trunk regions, which turned out to be a decisive PD biomarker.
更多查看译文
关键词
Gait analysis,Kinematic analysis,Dense trajectories,Parkinson’s disease
AI 理解论文
溯源树
样例
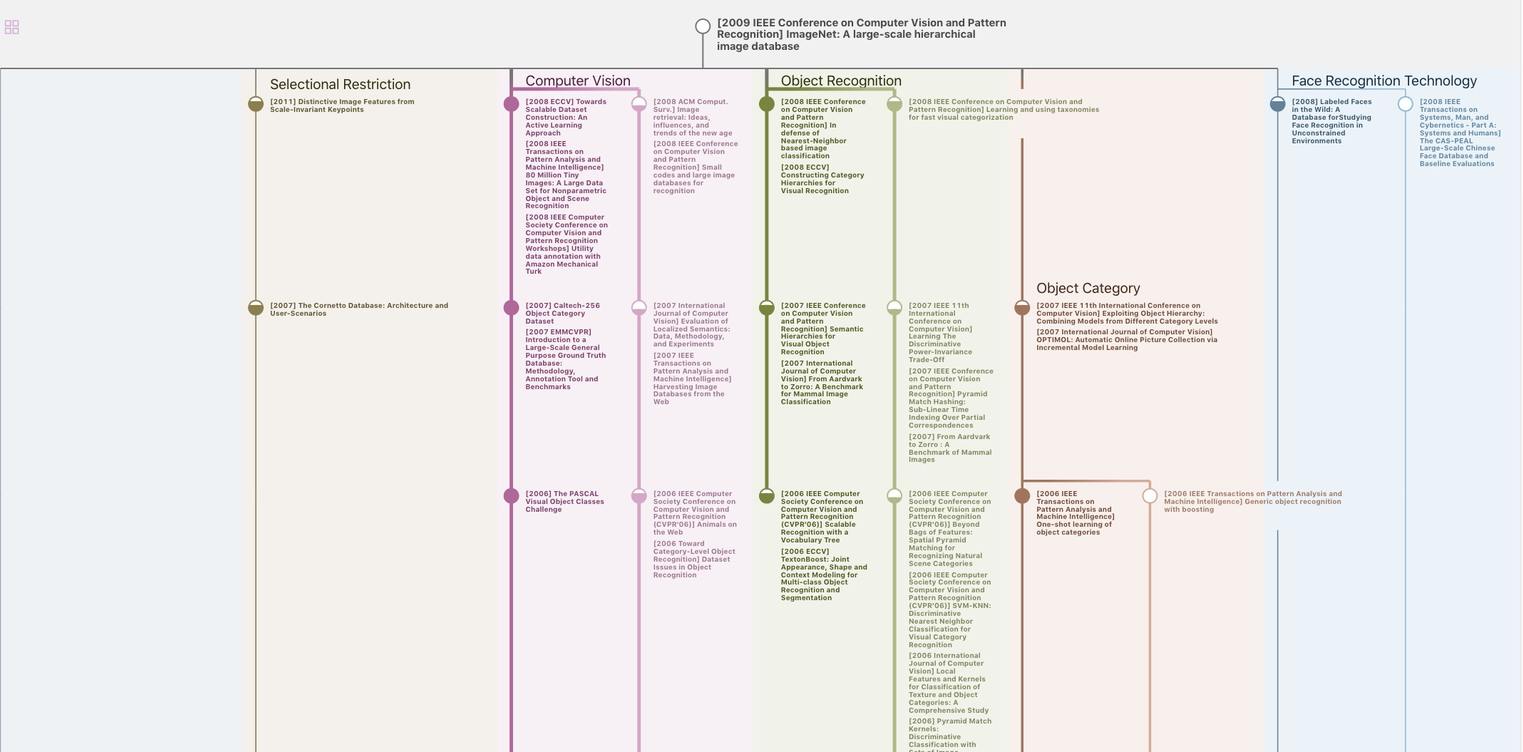
生成溯源树,研究论文发展脉络
Chat Paper
正在生成论文摘要