Integrated framework for EMD-Boruta-LDA feature extraction and SVM classification in coal and gas outbursts
JOURNAL OF EXPERIMENTAL & THEORETICAL ARTIFICIAL INTELLIGENCE(2023)
摘要
Coal and gas outbursts classification has become more important than before due to the serious threat to the safety of coal production, in this paper, we proposed a novel combination model consists of feature decomposition and reconstruction, feature selection and feature extraction for classification of coal and gas outbursts. First, EMD is used to decompose the coal and gas outbursts index features into a number of different IMFS; Second, in order to find out the relevance of IMFS with regard to the features, a wrapper algorithm Boruta with the RF classifier is employed, and the IMFS which has high relevance with the feature are selected to form a new index feature, then the new obtained features construct new influencing factors that affect coal and gas outbursts; Furtherly, in order to eliminate the redundancy between the new generated features and the uncorrelation between the index features and outbursts, the LDA is used to extract the features with class differentiation. Finally, the SVM classifiers based on the optimal parameters by Bayesian optimisation algorithm is employed to evaluate the proposed feature extraction scheme. Experimental results show that the proposed comprehensive model can achieve significant performance in terms of classification accuracy and feature size compared to existing methods for coal and gas outbursts classification.
更多查看译文
关键词
Coal and gas outbursts, Empirical mode decomposition(EMD), Boruta, linear discriminant analysis(LDA), support vector machine(SVM)
AI 理解论文
溯源树
样例
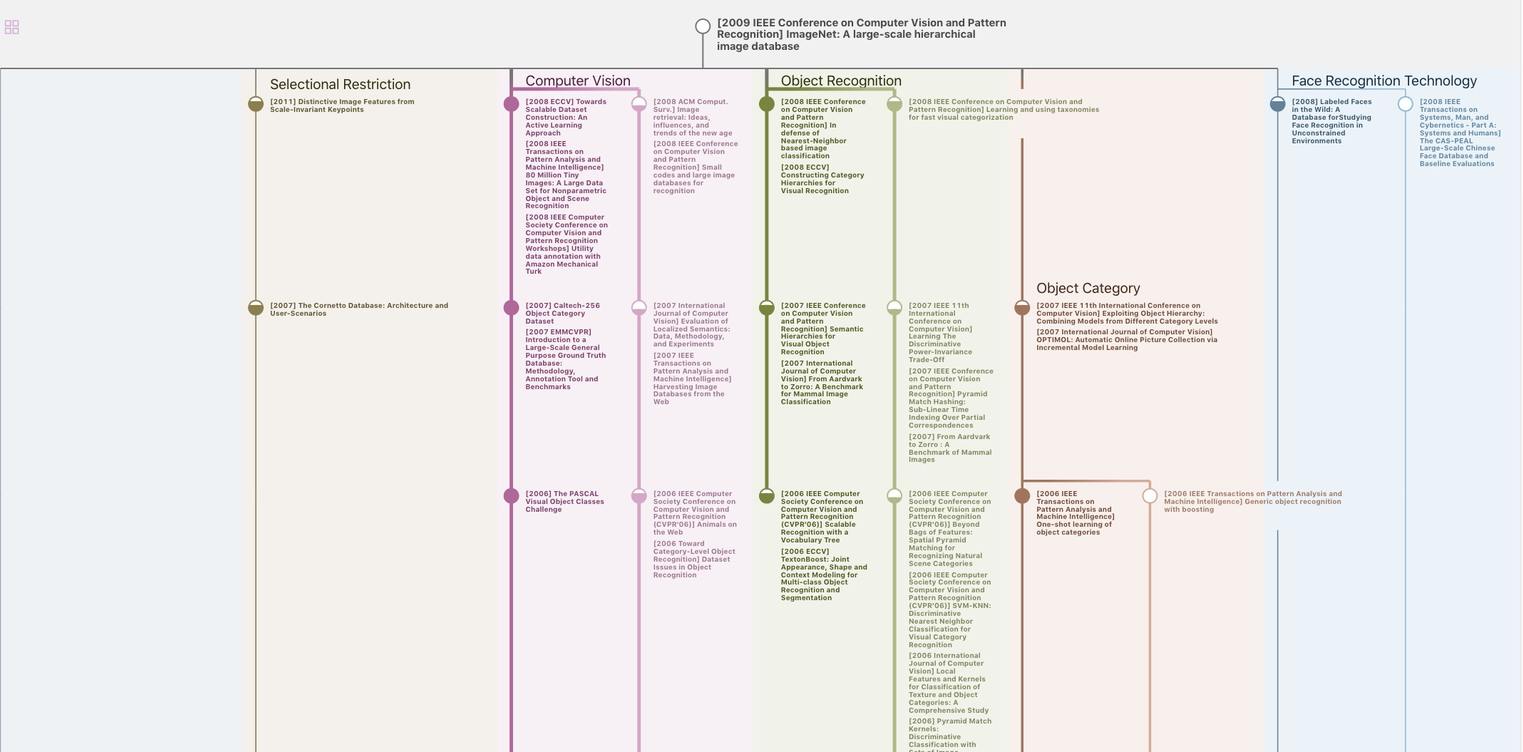
生成溯源树,研究论文发展脉络
Chat Paper
正在生成论文摘要