Double Fourier Integral Analysis Based Convolutional Neural Network Regression for High-Frequency Energy Disaggregation
IEEE Transactions on Emerging Topics in Computational Intelligence(2022)
摘要
Non-Intrusive Load Monitoring aims to extract the energy consumption of individual electrical appliances through disaggregation of the total power load measured by a single smart-meter. In this article we introduce Double Fourier Integral Analysis in the Non-Intrusive Load Monitoring task in order to provide more distinct feature descriptions compared to current or voltage spectrograms. Specifically, the high-frequency aggregated current and voltage signals are transformed into two-dimensional unit cells as calculated by Double Fourier Integral Analysis and used as input to a Convolutional Neural Network for regression. The performance of the proposed methodology was evaluated in the publicly available U.K.-DALE dataset. The proposed approach improves the estimation accuracy by 7.2% when compared to the baseline energy disaggregation setup using current and voltage spectrograms.
更多查看译文
关键词
Energy disaggregation,non-intrusive load monitoring (NILM),spectrogram,double fourier integral analysis (DFIA)
AI 理解论文
溯源树
样例
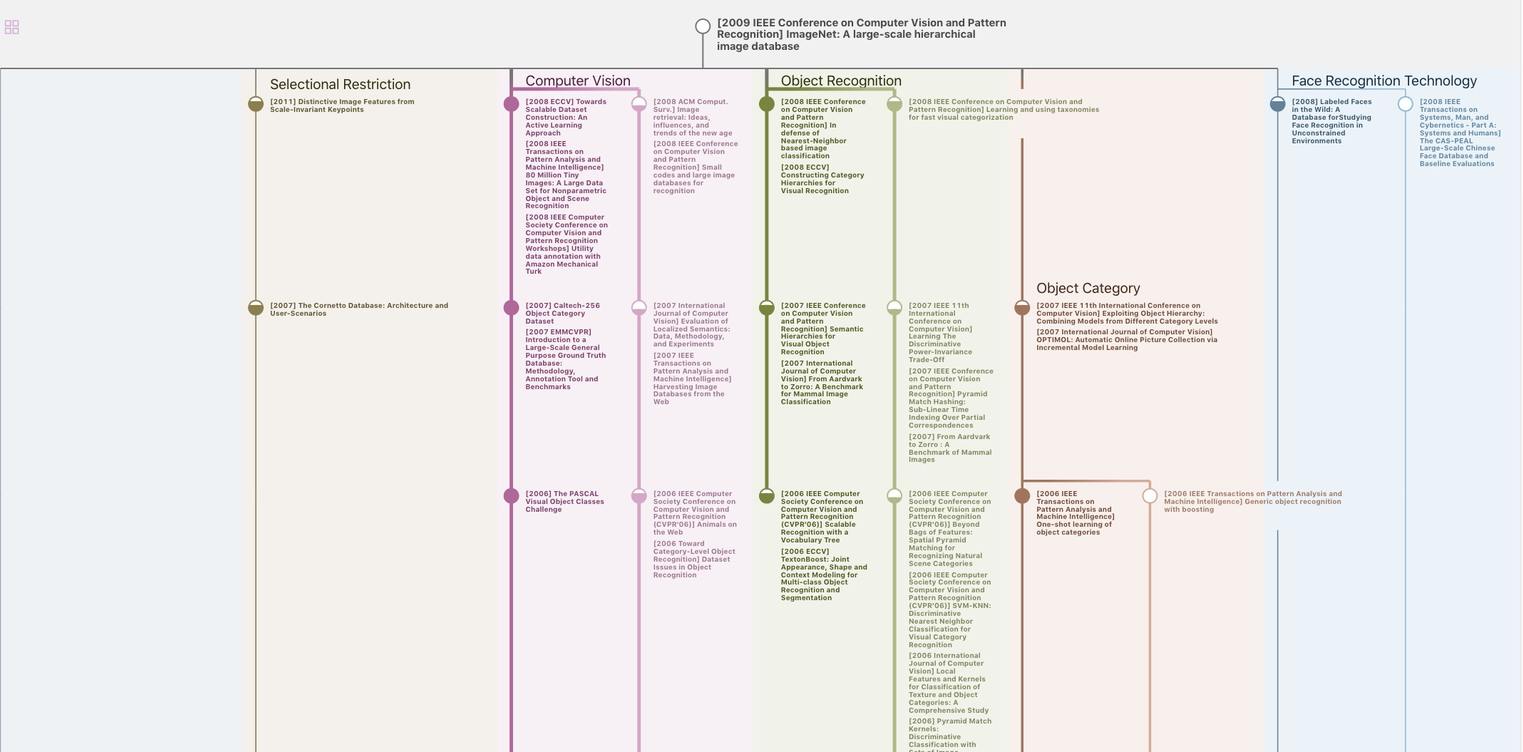
生成溯源树,研究论文发展脉络
Chat Paper
正在生成论文摘要