Automated visual surveying of vehicle heights to help measure the risk of overheight collisions using deep learning and view geometry
COMPUTER-AIDED CIVIL AND INFRASTRUCTURE ENGINEERING(2023)
摘要
Overheight vehicle collisions continuously pose a serious threat to transportation infrastructure and public safety. This study proposed a vision-based method for automatic vehicle height measurement using deep learning and view geometry. In this method, vehicle instances are first segmented from traffic surveillance video frames by exploiting mask region-based convolutional neural network (Mask R-CNN). Then, 3D bounding box on each vehicle instance is constructed using the obtained vehicle silhouette and three orthogonal vanishing points in the surveilled traffic scene. By doing so, the vertical edges of the constructed 3D bounding box are directly associated with the vehicle image height. Last, the vehicle's physical height is computed by referencing an object with a known height in the traffic scene using single view metrology. A field experiment was performed to evaluate the performance of the proposed method, leading to the mean and maximum errors of 3.6 and 6.6, 5.8 and 12.9, 4.4 and 8.1, and 9.2 and 18.5 cm for cars, buses, vans, and trucks, respectively. The experiment also demonstrated the ability of the method to overcome vehicle occlusion, shadow, and irregular appearance interferences in height estimation suffered by existing image-based methods. The results signified the potential of the proposed method for overheight vehicle detection and collision warning in real traffic settings.
更多查看译文
AI 理解论文
溯源树
样例
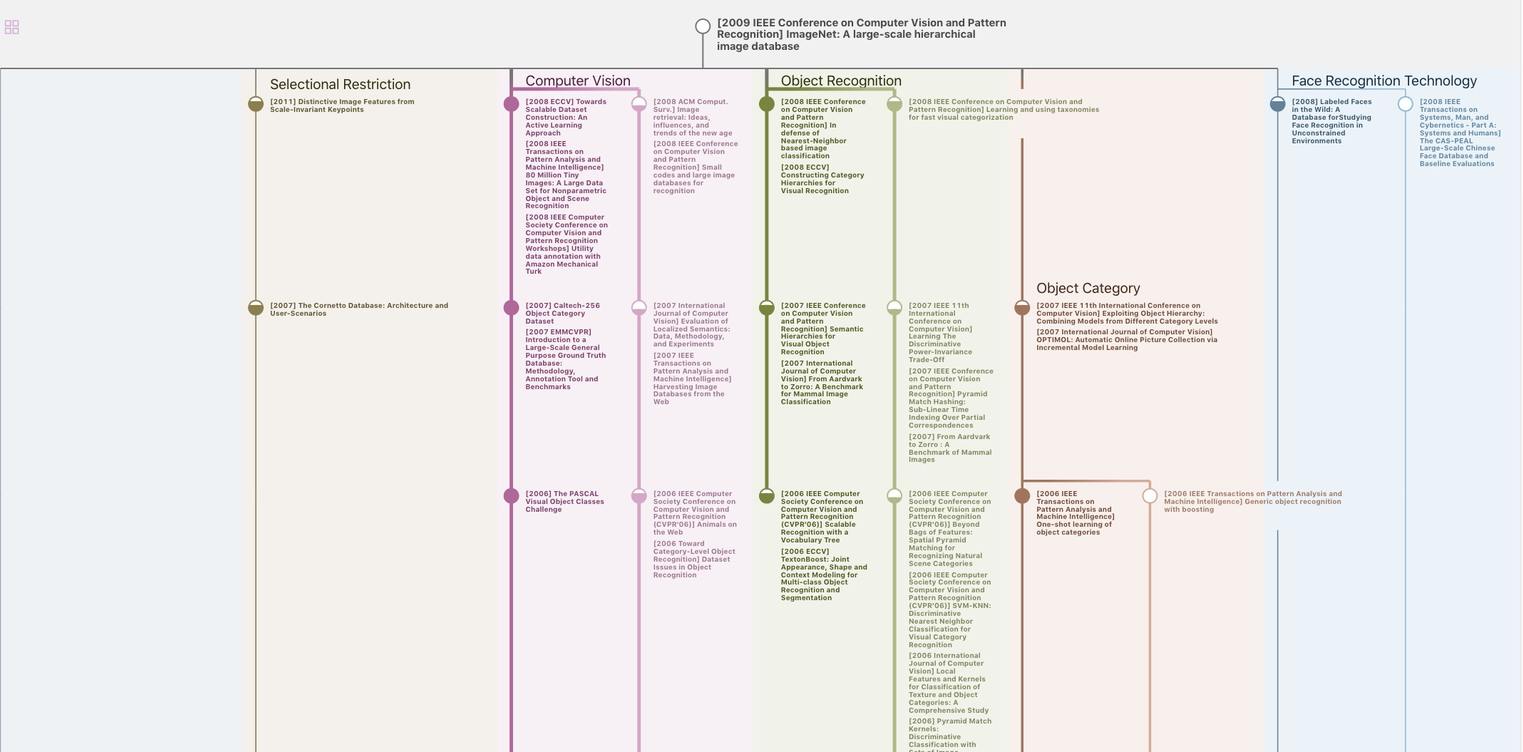
生成溯源树,研究论文发展脉络
Chat Paper
正在生成论文摘要