Imitation Learning with Graph Neural Networks for Improving Swarm Robustness under Restricted Communications
APPLIED SCIENCES-BASEL(2021)
摘要
This paper focuses on generating distributed flocking strategies via imitation learning. The primary motivation is to improve the swarm robustness and achieve better consistency while respecting the communication constraints. This paper first proposes a quantitative metric of swarm robustness based on entropy evaluation. Then, the graph importance consistency is also proposed, which is one of the critical goals of the flocking task. Moreover, the importance-correlated directed graph convolutional networks (IDGCNs) are constructed for multidimensional feature extraction and structure-related aggregation of graph data. Next, by employing IDGCNs-based imitation learning, a distributed and scalable flocking strategy is obtained, and its performance is very close to the centralized strategy template while considering communication constraints. To speed up and simplify the training process, we train the flocking strategy with a small number of agents and set restrictions on communication. Finally, various simulation experiments are executed to verify the advantages of the obtained strategy in terms of realizing the swarm consistency and improving the swarm robustness. The results also show that the performance is well maintained while the scale of agents expands (tested with 20, 30, 40 robots).
更多查看译文
关键词
swarm robustness, graph importance entropy, graph convolutional networks, imitation learning
AI 理解论文
溯源树
样例
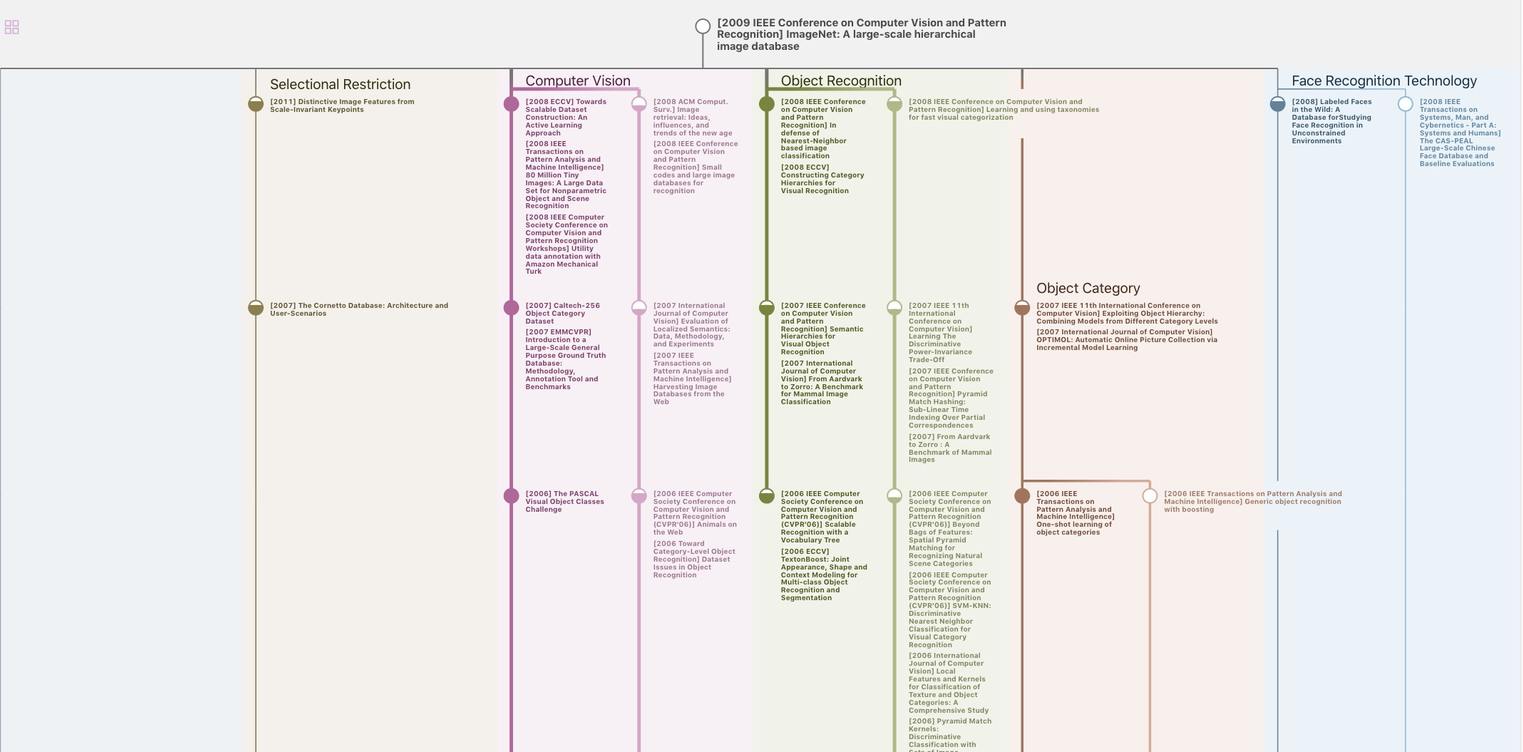
生成溯源树,研究论文发展脉络
Chat Paper
正在生成论文摘要