Optimal Management for EV Charging Stations: A Win–Win Strategy for Different Stakeholders Using Constrained Deep Q-Learning
Energies(2022)
摘要
Given the additional awareness of the increasing energy demand and gas emissions’ effects, the decarbonization of the transportation sector is of great significance. In particular, the adoption of electric vehicles (EVs) seems a promising option, under the condition that public charging infrastructure is available. However, devising a pricing and scheduling strategy for public EV charging stations is a non-trivial albeit important task. The reason is that a sub-optimal decision could lead to high waiting times or extreme changes to the power load profile. In addition, in the context of the problem of optimal pricing and scheduling for EV charging stations, the interests of different stakeholders ought to be taken into account (such as those of the station owner and the EV owners). This work proposes a deep reinforcement learning-based (DRL) agent that can optimize pricing and charging control in a public EV charging station under a real-time varying electricity price. The primary goal is to maximize the station’s profits while simultaneously ensuring that the customers’ charging demands are also satisfied. Moreover, the DRL approach is data-driven; it can operate under uncertainties without requiring explicit models of the environment. Variants of scheduling and DRL training algorithms from the literature are also proposed to ensure that both the conflicting objectives are achieved. Experimental results validate the effectiveness of the proposed approach.
更多查看译文
关键词
dynamic pricing,EV charging station,pricing and scheduling,reinforcement learning,deep Q-learning,demand response
AI 理解论文
溯源树
样例
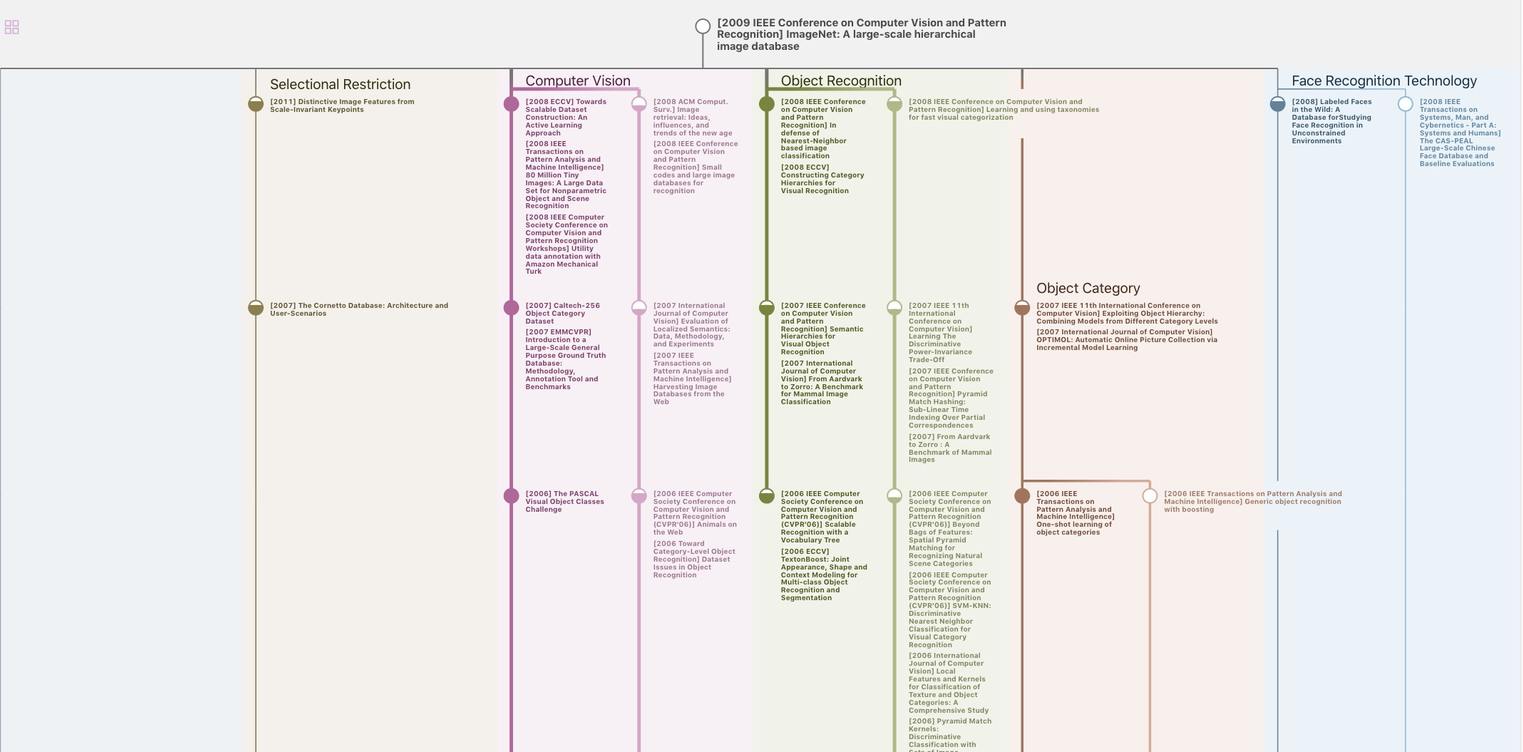
生成溯源树,研究论文发展脉络
Chat Paper
正在生成论文摘要