Modeling Semiarid River-Aquifer Systems with Bayesian Networks and Artificial Neural Networks
MATHEMATICS(2022)
摘要
In semiarid areas, precipitations usually appear in the form of big and brief floods, which affect the aquifer through water infiltration, causing groundwater temperature changes. These changes may have an impact on the physical, chemical and biological processes of the aquifer and, thus, modeling the groundwater temperature variations associated with stormy precipitation episodes is essential, especially since this kind of precipitation is becoming increasingly frequent in semiarid regions. In this paper, we compare the predictive performance of two popular tools in statistics and machine learning, namely Bayesian networks (BNs) and artificial neural networks (ANNs), in modeling groundwater temperature variation associated with precipitation events. More specifically, we trained a total of 2145 ANNs with different node configurations, from one to five layers. On the other hand, we trained three different BNs using different structure learning algorithms. We conclude that, while both tools are equivalent in terms of accuracy for predicting groundwater temperature drops, the computational cost associated with the estimation of Bayesian networks is significantly lower, and the resulting BN models are more versatile and allow a more detailed analysis.
更多查看译文
关键词
Bayesian networks, artificial neural networks, groundwater temperature, classification, semiarid areas
AI 理解论文
溯源树
样例
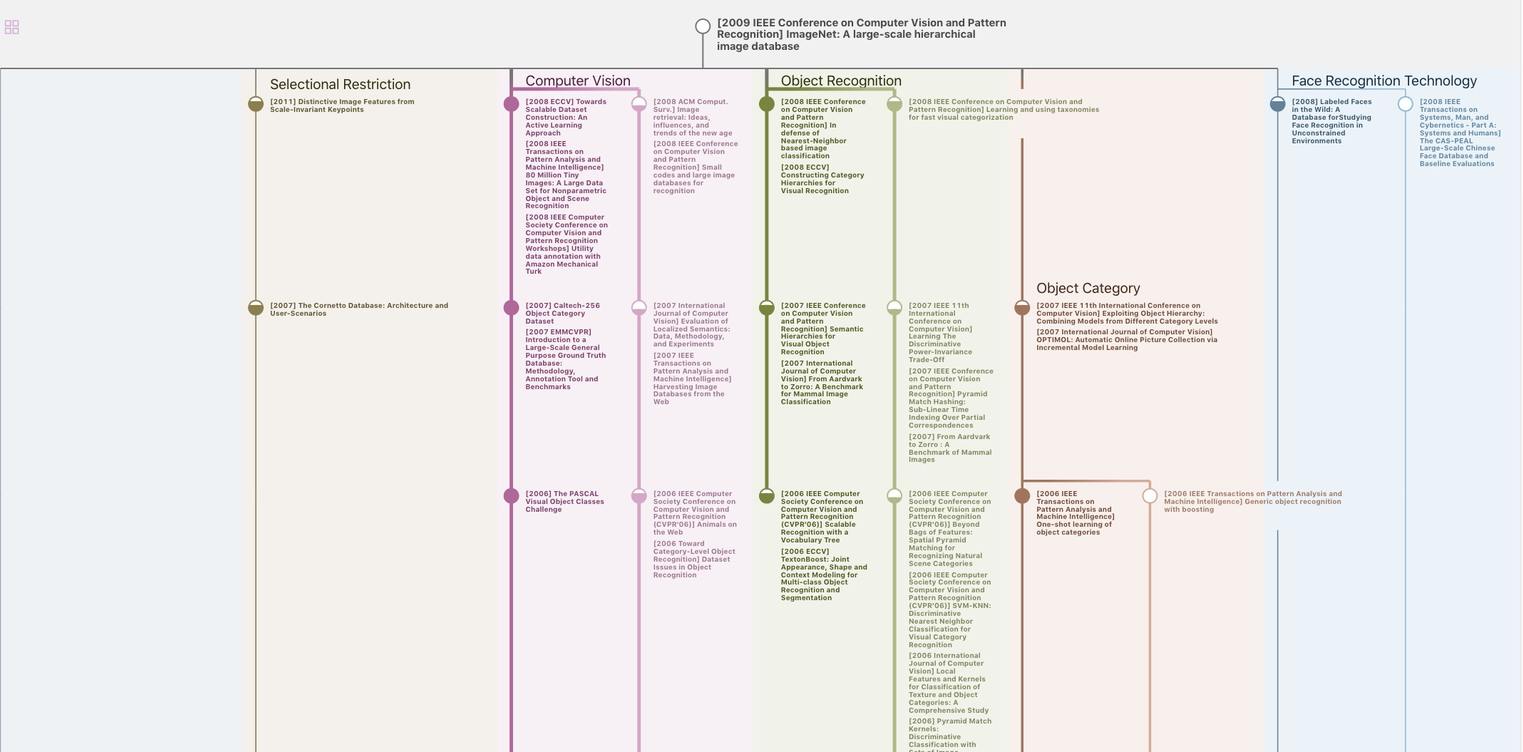
生成溯源树,研究论文发展脉络
Chat Paper
正在生成论文摘要