Bayesian Approach in a Learning-Based Hyperspectral Image Denoising Framework
IEEE ACCESS(2021)
摘要
Hyperspectral images (HSI) are corrupted by a combination of Gaussian and impulse noise. Successful denoising of HSI data increases the accuracy of high-level vision operations like classification, target tracking and land-cover problem. On the one hand, the traditional approach of handling the denoising problem using maximum a posteriori (MAP) criterion is often restricted by the time-consuming iterative optimization process and design of hand-crafted priors to obtain an optimal result. On the other hand, the discriminative learning-based approaches offer fast inference speed over a trained model; but are highly sensitive to the noise level used for training. A discriminative model trained with a loss function which does not accord with the Bayesian degradation process often leads to sub-optimal results. In this paper, we design the training paradigm emphasizing the role of loss functions in neural network; similar to as observed in model-based optimization methods. Further, Bayesian motivated loss functions also act as priors to constrain the solution space to the types of noise observed in hyperspectral image acquisition process. As a result, loss functions derived in Bayesian setting and employed in neural network training boosts the denoising performance. Extensive analysis and experimental results on synthetically corrupted and real hyperspectral datasets suggest the potential applicability of the proposed technique under a wide range of homogeneous and heterogeneous noisy settings. Classification results over the real dataset and associated metrics like kappa coefficient and overall accuracy used as the task-based evaluators further support our hypothesis made in the proposed methodology.
更多查看译文
关键词
Noise measurement, Training, Noise reduction, Estimation, Convolutional neural networks, Bayes methods, Neural networks, Bayesian estimation, discriminative learning, Gaussian-impulse noise, hyperspectral imaging, residual learning
AI 理解论文
溯源树
样例
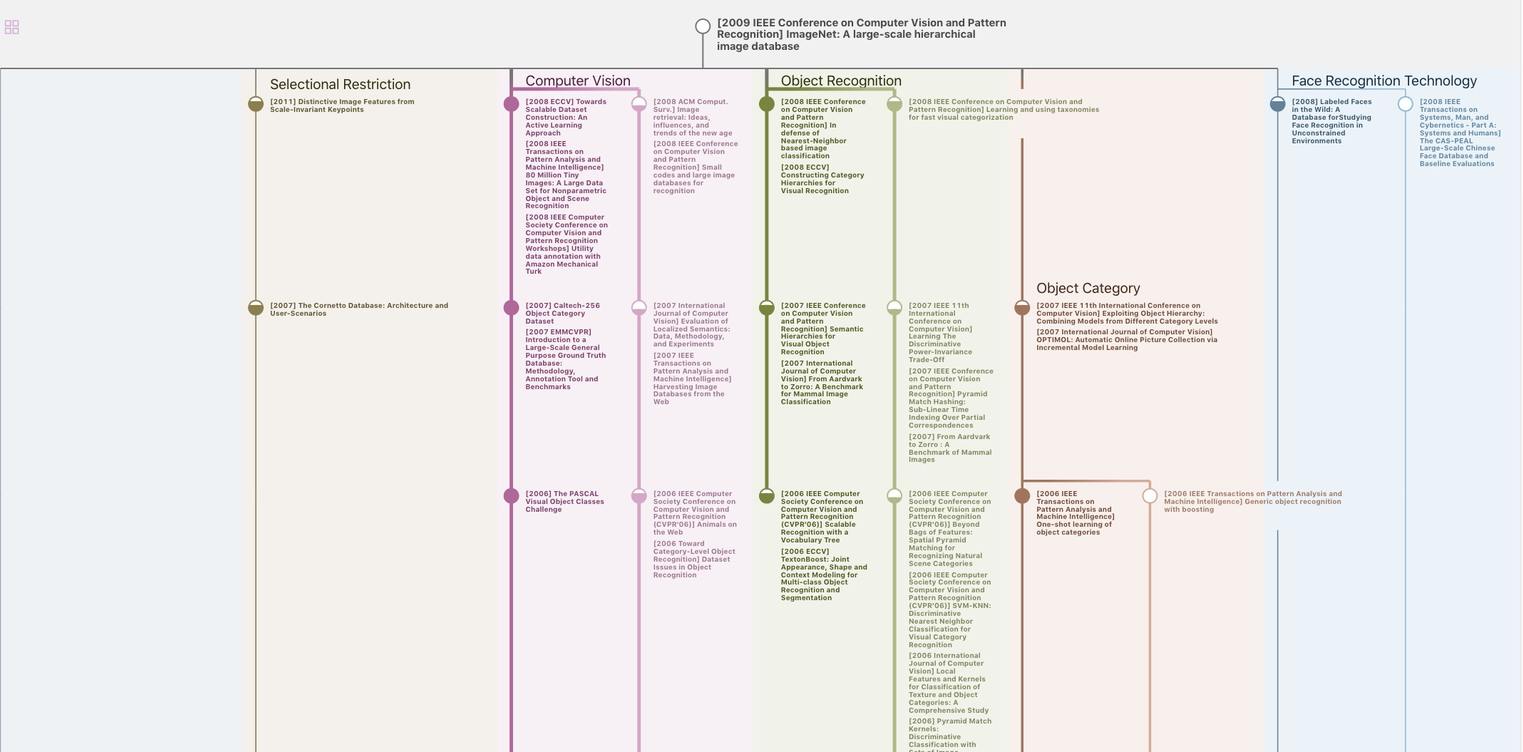
生成溯源树,研究论文发展脉络
Chat Paper
正在生成论文摘要