Searching for memory-lighter architectures for OCR-augmented image captioning
JOURNAL OF INTELLIGENT & FUZZY SYSTEMS(2022)
摘要
Current State-of-the-Art image captioning systems that can read and integrate read text into the generated descriptions need high processing power and memory usage, which limits the sustainability and usability of the models (as they require expensive and very specialized hardware). The present work introduces two alternative versions (L-M4C and L-CNMT) of top architectures (on the TextCaps challenge), which were mainly adapted to achieve near-State-of-The-Art performance while being memory-lighter when compared to the original architectures, this is mainly achieved by using distilled or smaller pre-trained models on the text-and-OCR embedding modules. On the one hand, a distilled version of BERT was used in order to reduce the size of the text-embedding module (the distilled model has 59% fewer parameters), on the other hand, the OCR context processor on both architectures was replaced by Global Vectors (GloVe), instead of using FastText pre-trained vectors, this can reduce the memory used by the OCR-embedding module up to a 94%. Two of the three models presented in this work surpassed the baseline (M4C-Captioner) of the challenge on the evaluation and test sets, also, our best lighter architecture reached a CIDEr score of 88.24 on the test set, which is 7.25 points above the baseline model.
更多查看译文
关键词
M4C-Captioner, MMF, multimodal transformers, reading comprehension, TextCaps challenge
AI 理解论文
溯源树
样例
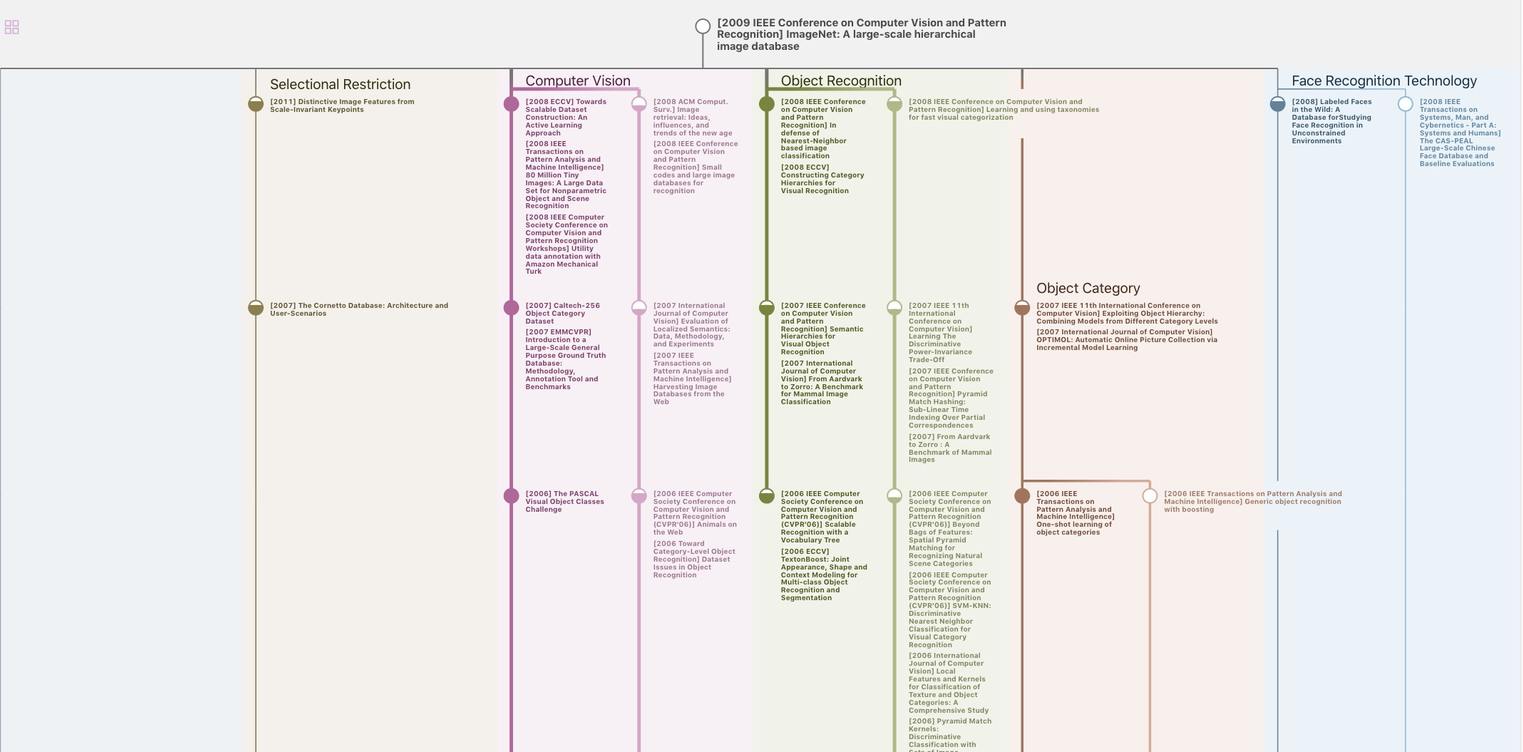
生成溯源树,研究论文发展脉络
Chat Paper
正在生成论文摘要