Boosting subjective quality of Arabic text-to-speech (TTS) using end-to-end deep architecture
International Journal of Speech Technology(2022)
摘要
End-to-end speech synthesis methods managed to achieve nearly natural and human-like speech. They are prone to some synthesis errors such as missing or repeating words, or incomplete synthesis. We may argue this is mainly due to the local information preference between text input and the learned acoustic features of a conditional autoregressive (CAR) model. The local information preference prevents the model from depending on text input when predicting acoustic features. It contributes to synthesis errors during inference time. In this work, we are comparing two modified architectures based on Tacotron2 to generate Arabic speech. The first architecture replaces the WaveNet vocoder with a flow-based implementation of WaveGlow. The second architecture, influenced by InfoGan, maximizes the mutual information between text input and predicted acoustic features (mel-spectrogram) to eliminate the local information preference. The training objective has been also changed by adding a CTC loss term. The training objective could be considered as a metric of local information preference between text input and predicted acoustic features. We carried the experiments on Nawar Halabi’s dataset ( http://en.arabicspeechcorpus.com/ ) which contains about 2.41 h of Arabic speech. Our experiments show that maximizing mutual information between predicted acoustic features and conditional text input as well as changing the training objective can enhance the subjective quality of generated speech and reduce the utterance error rate.
更多查看译文
关键词
Tacotron 2, WaveGlow, InfoGan, Arabic text-to-speech, Speech synthesis, Deep learning, Neural networks
AI 理解论文
溯源树
样例
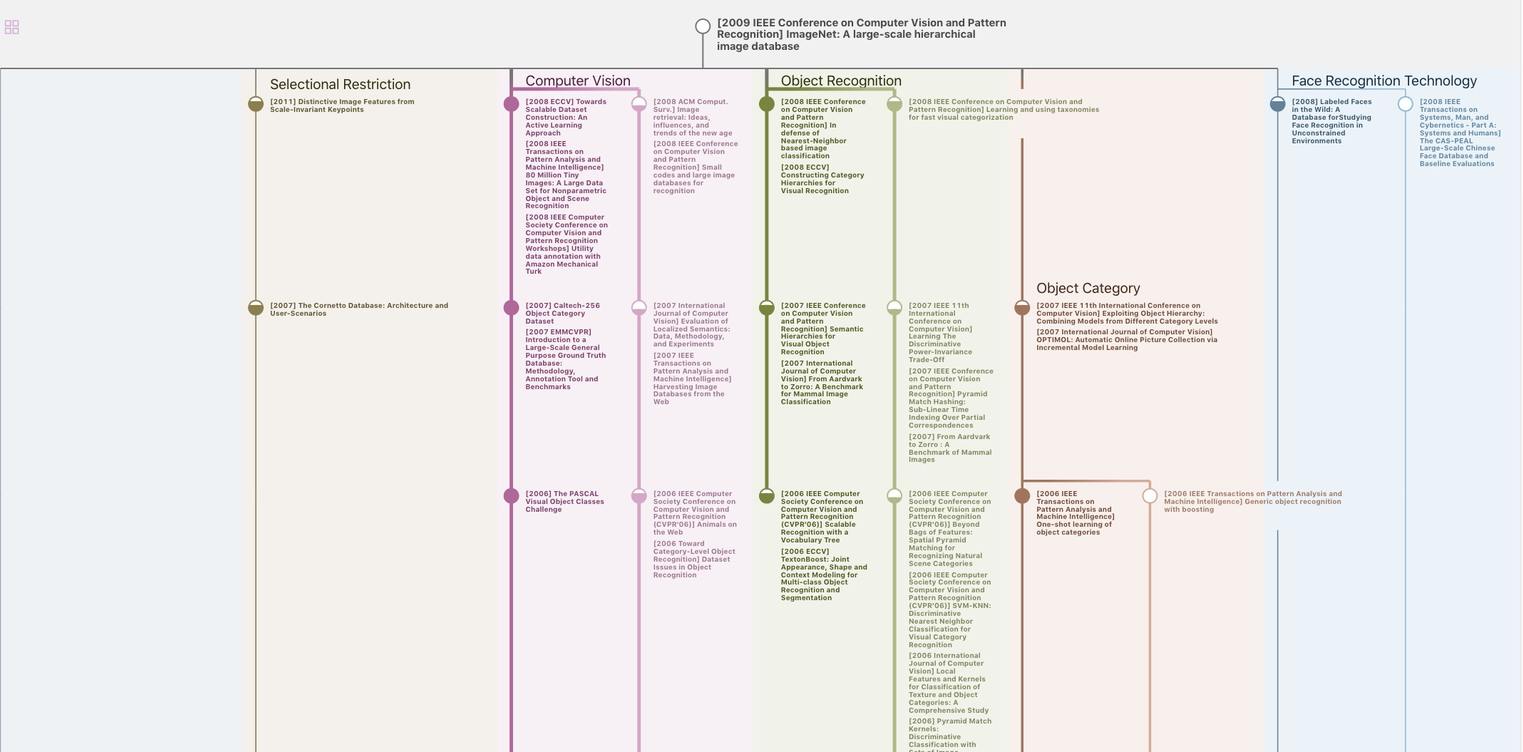
生成溯源树,研究论文发展脉络
Chat Paper
正在生成论文摘要