Improving Human Image Synthesis with Residual Fast Fourier Transformation and Wasserstein Distance
IEEE International Joint Conference on Neural Network (IJCNN)(2022)
摘要
With the rapid development of the Metaverse, virtual humans have emerged, and human image synthesis and editing techniques, such as pose transfer, have recently become popular. Most of the existing techniques rely on GANs, which can generate good human images even with large variants and occlusions. But from our best knowledge, the existing state-of-the-art method still has the following problems: the first is that the rendering effect of the synthetic image is not realistic, such as poor rendering of some regions. And the second is that the training of GAN is unstable and slow to converge, such as model collapse. Based on the above two problems, we propose several methods to solve them. To improve the rendering effect, we use the Residual Fast Fourier Transform Block to replace the traditional Residual Block. Then, spectral normalization and Wasserstein distance are used to improve the speed and stability of GAN training. Experiments demonstrate that the methods we offer are effective at solving the problems listed above, and we get state-of-the-art scores in LPIPS and PSNR.
更多查看译文
关键词
human image synthesis,GAN,residual fourier transform,Metaverse
AI 理解论文
溯源树
样例
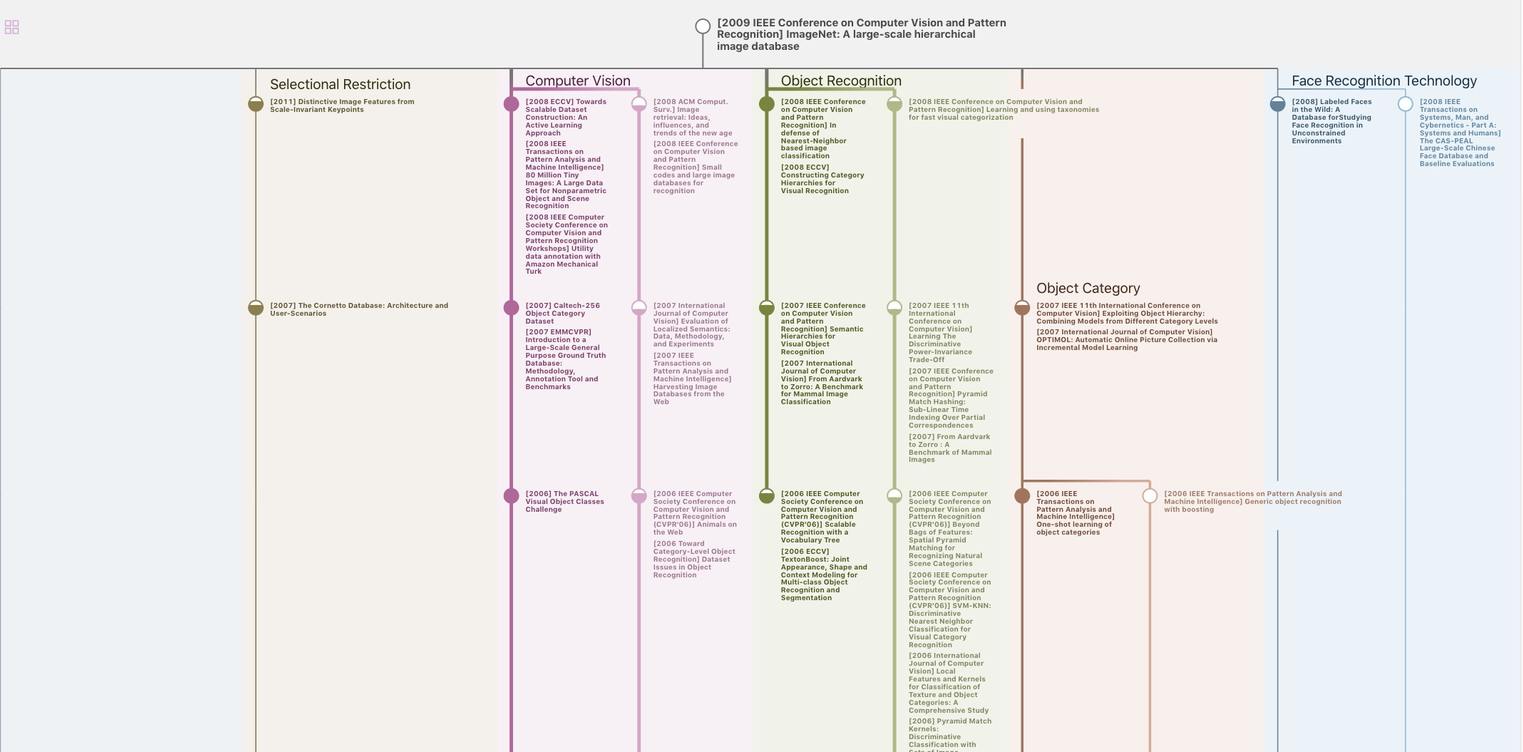
生成溯源树,研究论文发展脉络
Chat Paper
正在生成论文摘要