Information-Directed Selection for Top-Two Algorithms
arxiv(2023)
摘要
We consider the best-k-arm identification problem for multi-armed bandits, where the objective is to select the exact set of k arms with the highest mean rewards by sequentially allocating measurement effort. We characterize the necessary and sufficient conditions for the optimal allocation using dual variables. Remarkably these optimality conditions lead to the extension of top-two algorithm design principle (Russo, 2020), initially proposed for best-arm identification. Furthermore, our optimality conditions induce a simple and effective selection rule dubbed information-directed selection (IDS) that selects one of the top-two candidates based on a measure of information gain. As a theoretical guarantee, we prove that integrated with IDS, top-two Thompson sampling is (asymptotically) optimal for Gaussian best-arm identification, solving a glaring open problem in the pure exploration literature (Russo, 2020). As a by-product, we show that for k > 1, top-two algorithms cannot achieve optimality even with an oracle tuning parameter. Numerical experiments show the superior performance of the proposed top-two algorithms with IDS and considerable improvement compared with algorithms without adaptive selection.
更多查看译文
关键词
algorithms,selection,information-directed,top-two
AI 理解论文
溯源树
样例
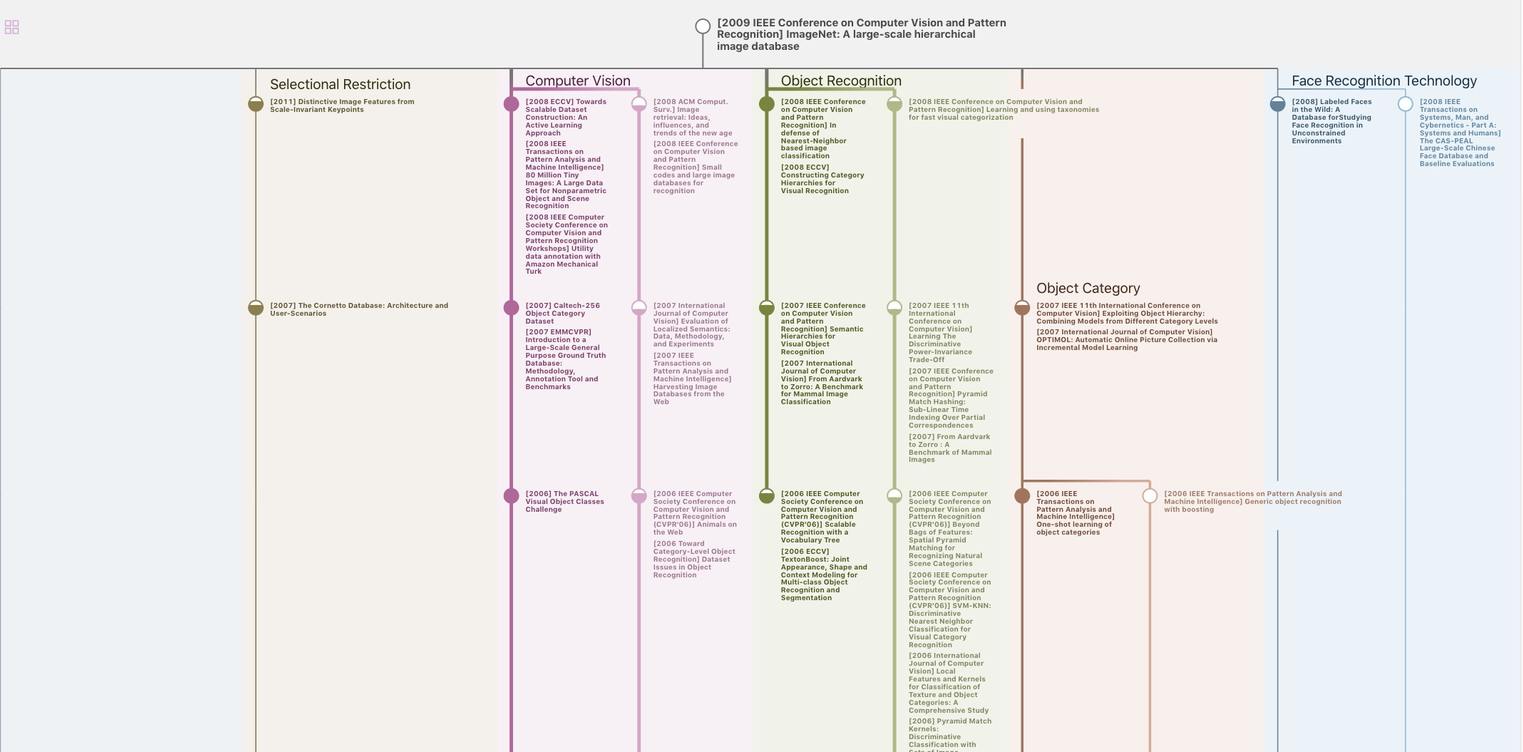
生成溯源树,研究论文发展脉络
Chat Paper
正在生成论文摘要