Linear Algorithms for Robust and Scalable Nonparametric Multiclass Probability Estimation
Journal of data science(2022)
摘要
Multiclass probability estimation is the problem of estimating conditional probabilities of a data point belonging to a class given its covariate information. It has broad applications in statistical analysis and data science. Recently a class of weighted Support Vector Machines (wSVMs) has been developed to estimate class probabilities through ensemble learning for K-class problems (Wu, Zhang and Liu, 2010; Wang, Zhang and Wu, 2019), where K is the number of classes. The estimators are robust and achieve high accuracy for probability estimation, but their learning is implemented through pairwise coupling, which demands polynomial time in K. In this paper, we propose two new learning schemes, the baseline learning and the One-vs-All (OVA) learning, to further improve wSVMs in terms of computational efficiency and estimation accuracy. In particular, the baseline learning has optimal computational complexity in the sense that it is linear in K. Though not being most efficient in computation, the OVA offers the best estimation accuracy among all the procedures under comparison. The resulting estimators are distribution-free and shown to be consistent. We further conduct extensive numerical experiments to demonstrate finite sample performance.
更多查看译文
关键词
Semi-Supervised Learning,Cost-Sensitive Learning,Active Learning,Deep Learning
AI 理解论文
溯源树
样例
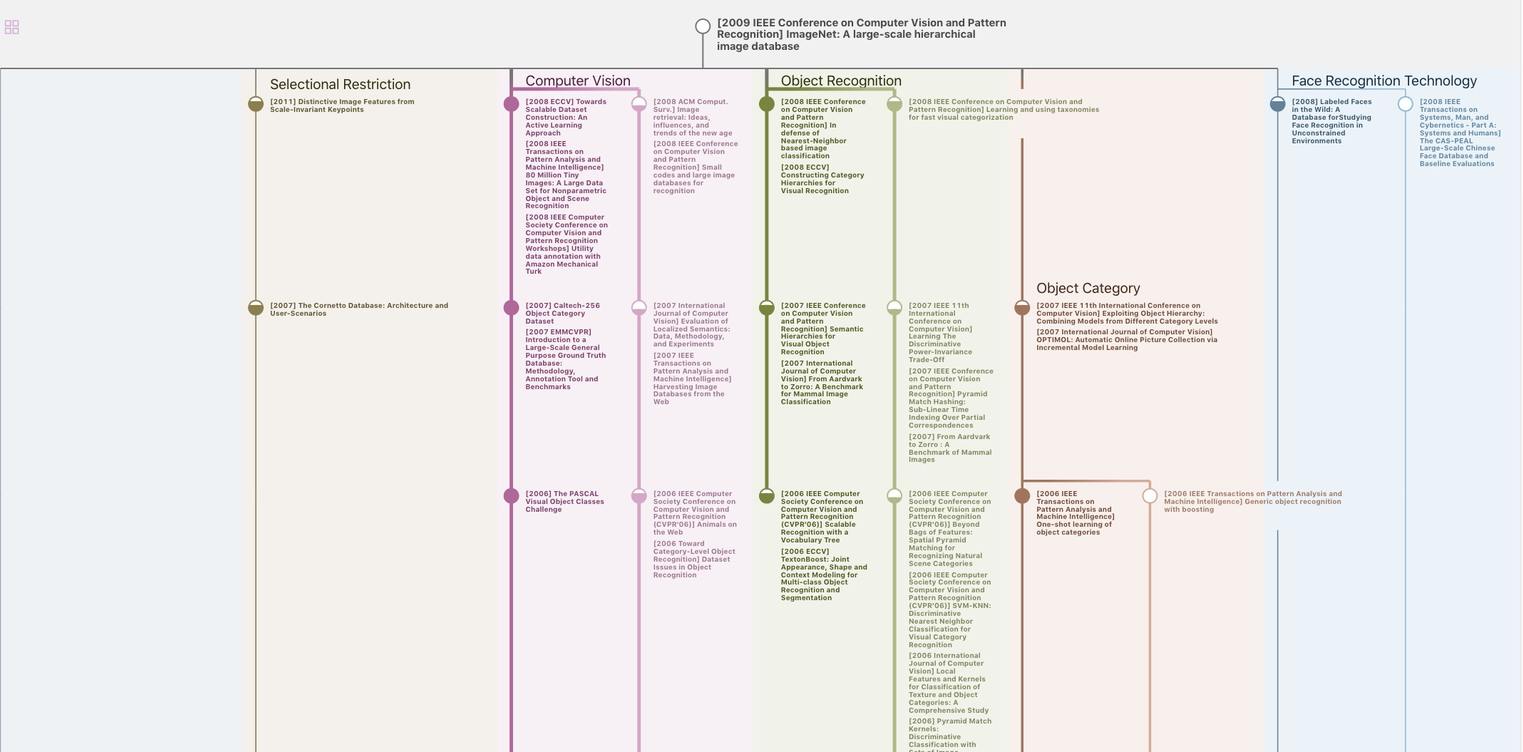
生成溯源树,研究论文发展脉络
Chat Paper
正在生成论文摘要