Learning to Query Internet Text for Informing Reinforcement Learning Agents
arxiv(2022)
摘要
Generalization to out of distribution tasks in reinforcement learning is a challenging problem. One successful approach improves generalization by conditioning policies on task or environment descriptions that provide information about the current transition or reward functions. Previously, these descriptions were often expressed as generated or crowd sourced text. In this work, we begin to tackle the problem of extracting useful information from natural language found in the wild (e.g. internet forums, documentation, and wikis). These natural, pre-existing sources are especially challenging, noisy, and large and present novel challenges compared to previous approaches. We propose to address these challenges by training reinforcement learning agents to learn to query these sources as a human would, and we experiment with how and when an agent should query. To address the \textit{how}, we demonstrate that pretrained QA models perform well at executing zero-shot queries in our target domain. Using information retrieved by a QA model, we train an agent to learn \textit{when} it should execute queries. We show that our method correctly learns to execute queries to maximize reward in a reinforcement learning setting.
更多查看译文
关键词
informing reinforcement learning,query internet text,reinforcement learning
AI 理解论文
溯源树
样例
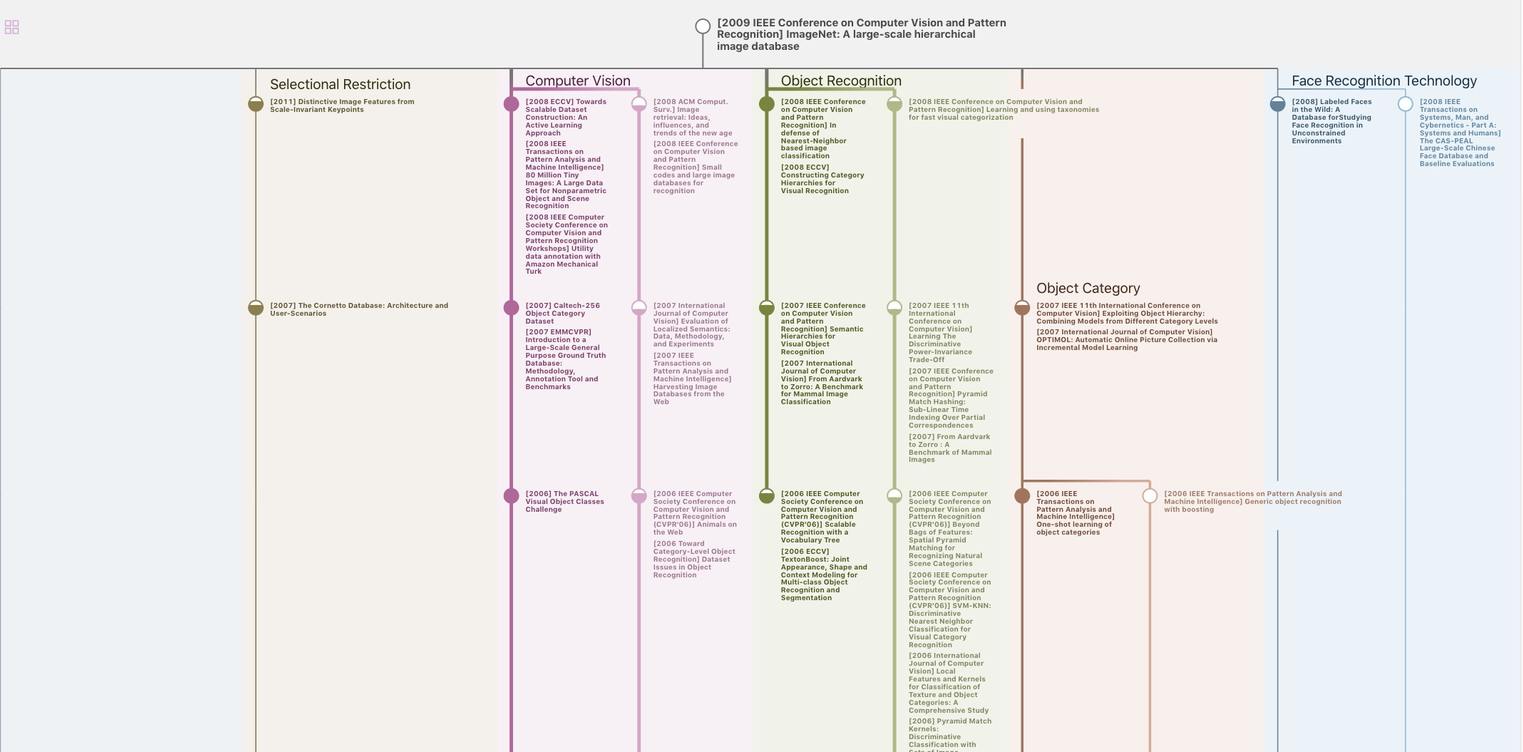
生成溯源树,研究论文发展脉络
Chat Paper
正在生成论文摘要