Orthogonal stochastic configuration networks with adaptive construction parameter for data analytics
Industrial Artificial Intelligence(2023)
摘要
As a randomized learner model, SCNs are remarkable that the random weights and biases are assigned employing a supervisory mechanism to ensure universal approximation and fast learning. However, the randomness makes SCNs more likely to generate approximate linear correlative nodes that are redundant and low quality, thereby resulting in non-compact network structure. In light of a fundamental principle in machine learning, that is, a model with fewer parameters holds improved generalization. This paper proposes orthogonal SCN, termed OSCN, to filtrate out the low-quality hidden nodes for network structure reduction by incorporating Gram–Schmidt orthogonalization technology. The universal approximation property of OSCN and an adaptive setting for the key construction parameters have been presented in details. In addition, an incremental updating scheme is developed to dynamically determine the output weights, contributing to improved computational efficiency. Finally, experimental results on two numerical examples and several real-world regression and classification datasets substantiate the effectiveness and feasibility of the proposed approach.
更多查看译文
关键词
Gram–Schmidt orthogonalization technology,Incremental updating scheme,Stochastic configuration networks,Universal approximation property
AI 理解论文
溯源树
样例
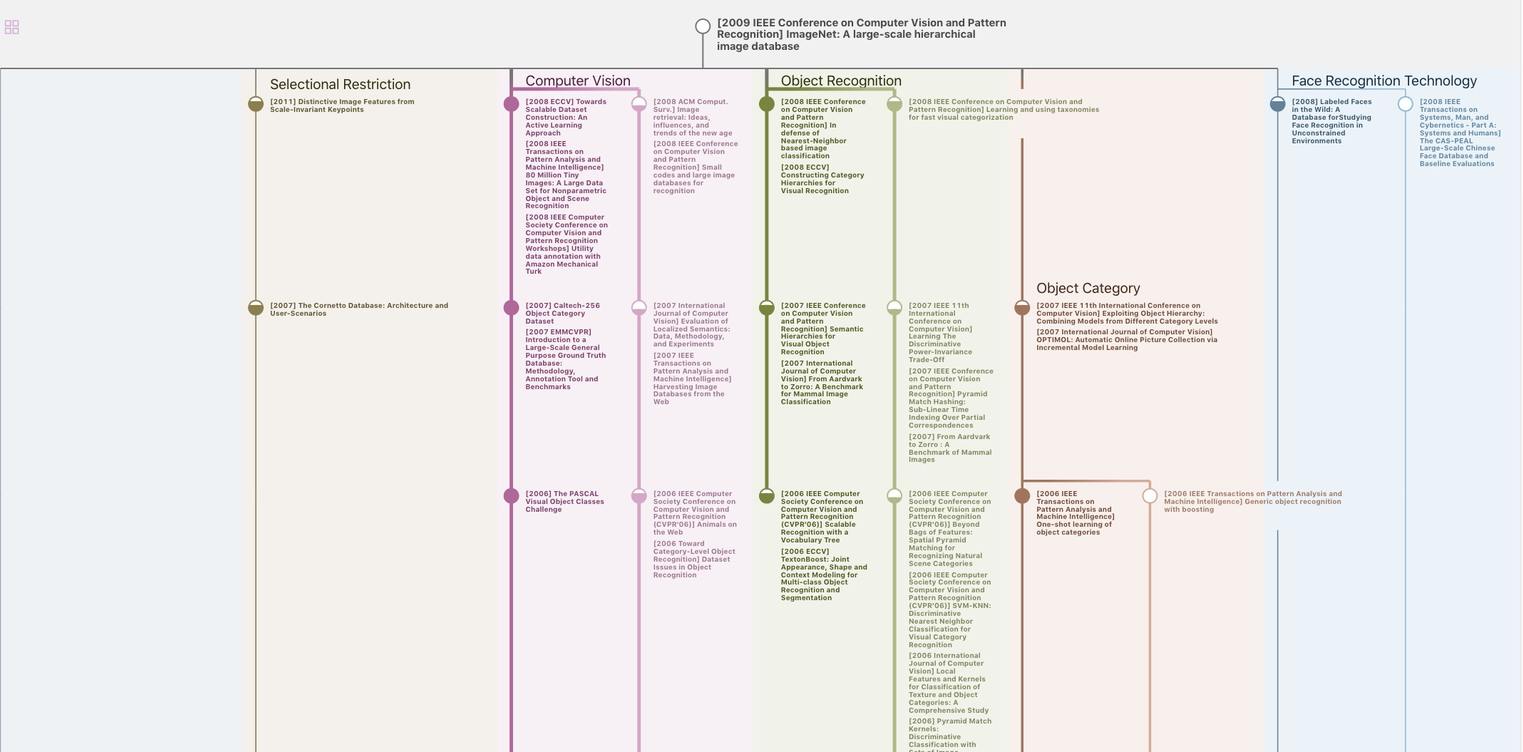
生成溯源树,研究论文发展脉络
Chat Paper
正在生成论文摘要