On Strong-Scaling and Open-Source Tools for High-Throughput Quantification of Material Point Cloud Data: Composition Gradients, Microstructural Object Reconstruction, and Spatial Correlations
arXiv (Cornell University)(2022)
摘要
Characterizing microstructure-material-property relations calls for software tools which extract point-cloud- and continuum-scale-based representations of microstructural objects. Application examples include atom probe, electron, and computational microscopy experiments. Mapping between atomic- and continuum-scale representations of microstructural objects results often in representations which are sensitive to parameterization; however assessing this sensitivity is a tedious task in practice. Here, we show how combining methods from computational geometry, collision analyses, and graph analytics yield software tools for automated analyses of point cloud data for reconstruction of three-dimensional objects, characterization of composition profiles, and extraction of multi-parameter correlations via evaluating graph-based relations between sets of meshed objects. Implemented for point clouds with mark data, we discuss use cases in atom probe microscopy that focus on interfaces, precipitates, and coprecipitation phenomena observed in different alloys. The methods are expandable for spatio-temporal analyses of grain fragmentation, crystal growth, or precipitation.
更多查看译文
关键词
material point cloud data,microstructural object reconstruction,composition gradients
AI 理解论文
溯源树
样例
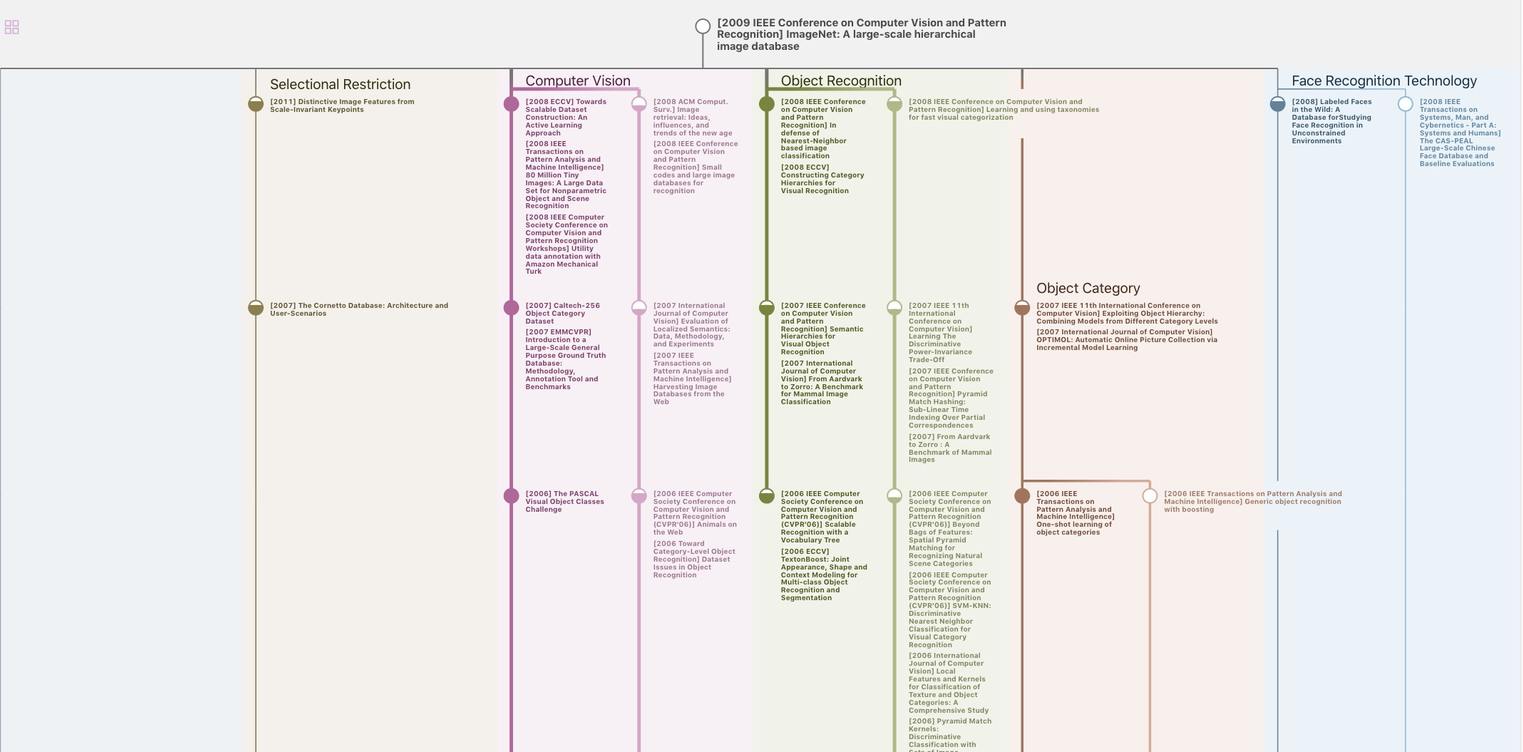
生成溯源树,研究论文发展脉络
Chat Paper
正在生成论文摘要