Selective Classification Via Neural Network Training Dynamics
arxiv(2022)
摘要
Selective classification is the task of rejecting inputs a model would predict incorrectly on through a trade-off between input space coverage and model accuracy. Current methods for selective classification impose constraints on either the model architecture or the loss function; this inhibits their usage in practice. In contrast to prior work, we show that state-of-the-art selective classification performance can be attained solely from studying the (discretized) training dynamics of a model. We propose a general framework that, for a given test input, monitors metrics capturing the disagreement with the final predicted label over intermediate models obtained during training; we then reject data points exhibiting too much disagreement at late stages in training. In particular, we instantiate a method that tracks when the label predicted during training stops disagreeing with the final predicted label. Our experimental evaluation shows that our method achieves state-of-the-art accuracy/coverage trade-offs on typical selective classification benchmarks.
更多查看译文
关键词
classification,selective,neural network,dynamics,training
AI 理解论文
溯源树
样例
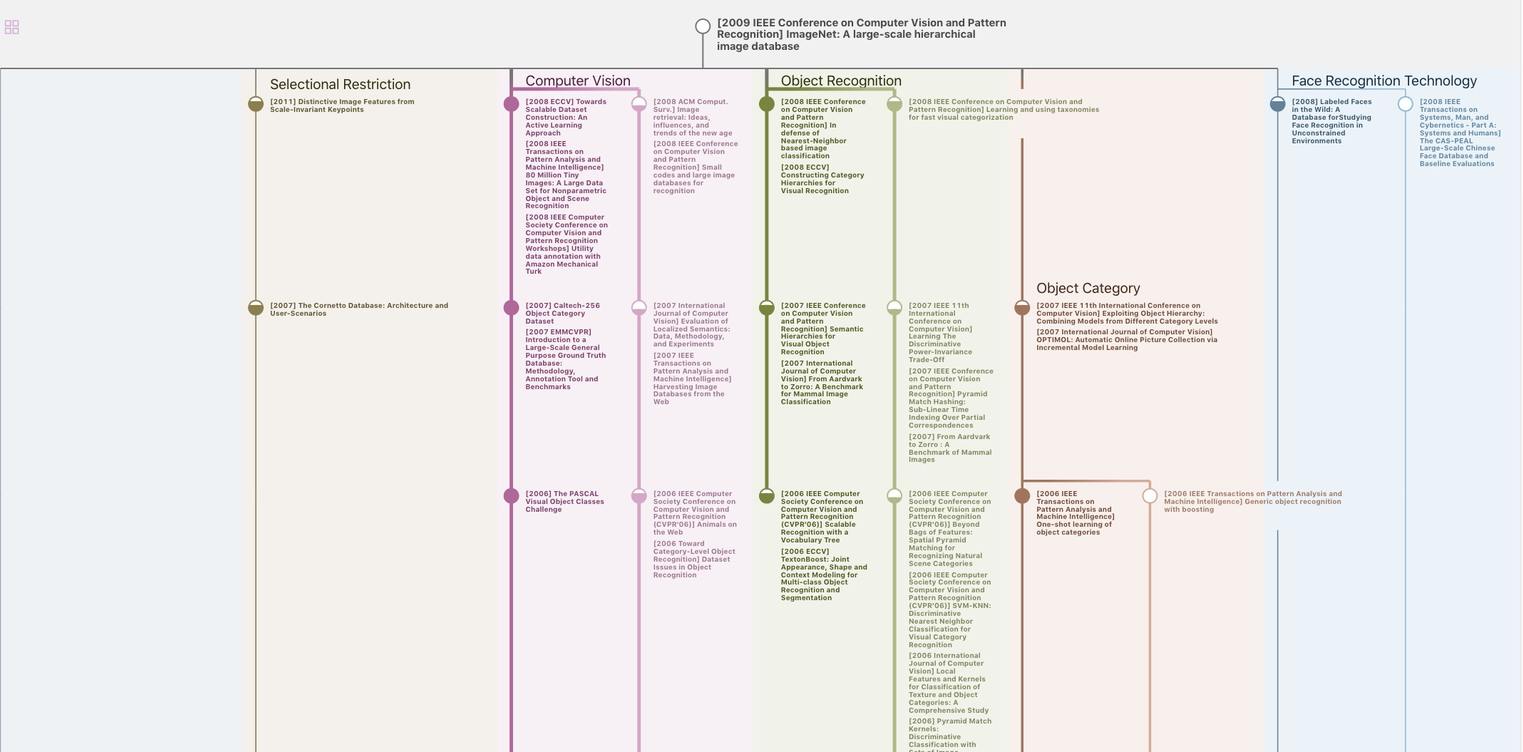
生成溯源树,研究论文发展脉络
Chat Paper
正在生成论文摘要