Energy Procurement and Retail Pricing for Electricity Retailers via Deep Reinforcement Learning with Long Short-term Memory
CSEE Journal of Power and Energy Systems(2022)
摘要
The joint optimization problem of energy procurement and retail pricing for an electricity retailer is converted into separately determining the optimal procurement strategy and optimal pricing strategy, under the “price-taker” assumption. The aggregate energy consumption of end use customers (EUCs) is predicted to solve for the optimal procurement strategy vis a long short-term memory (LSTM)-based supervised learning method. The optimal retail pricing problem is formulated as a Markov decision process (MDP), which can be solved by using deep reinforcement learning (DRL) algorithms. However, the performance of existing DRL approaches may deteriorate due to their insufficient ability to extract discriminative features from the time-series vectors in the environmental states. We propose a novel deep deterministic policy gradient (DDPG) network structure with a shared LSTM-based representation network that fully exploits the Actor's and Critic's losses. The designed shared representation network and the joint loss function can enhance the environment perception capability of the proposed approach and further improve the optimization performance, resulting in a more profitable pricing strategy. Numerical simulations demonstrate the effectiveness of the proposed approach.
更多查看译文
关键词
Deep reinforcement learning,electricity market,energy procurement,long short-term memory,retail pricing
AI 理解论文
溯源树
样例
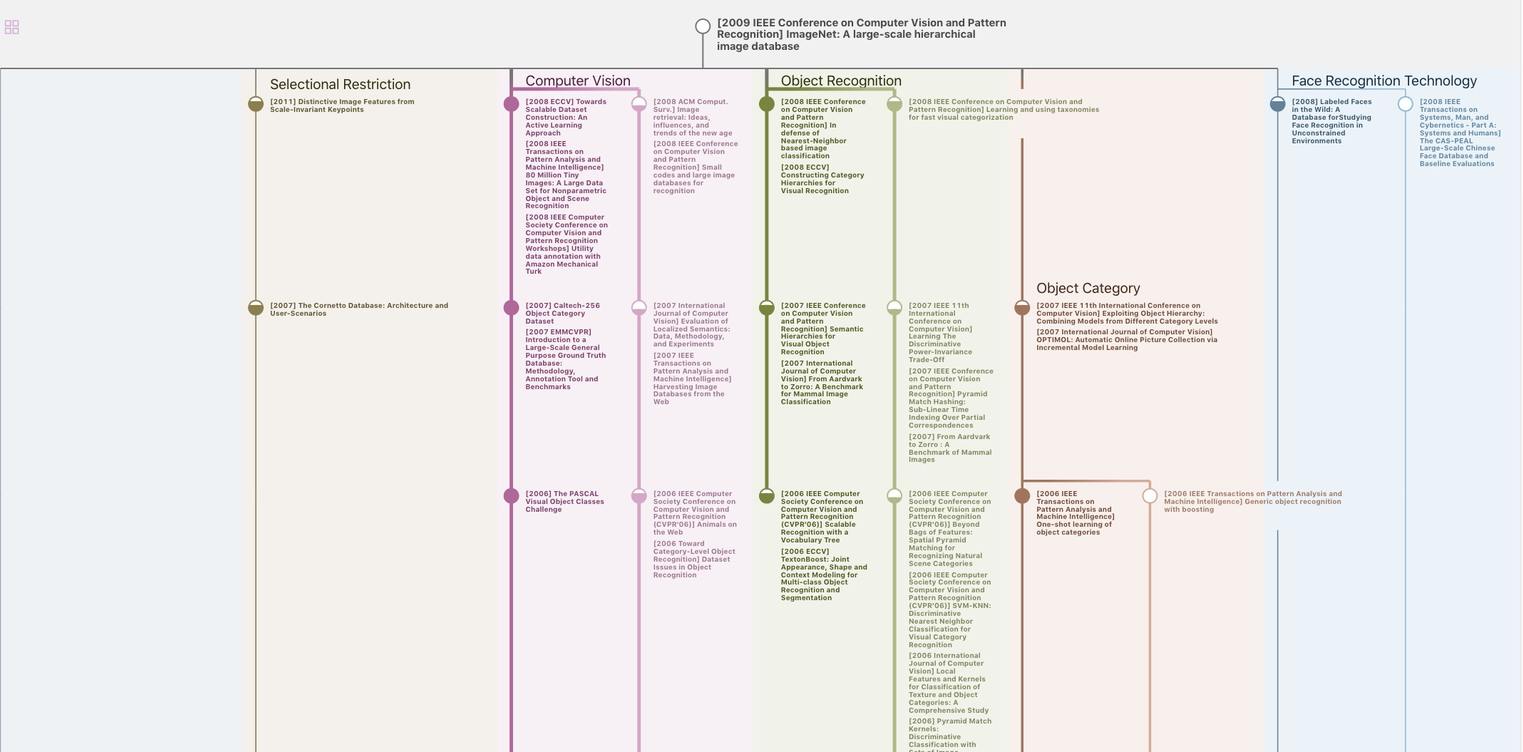
生成溯源树,研究论文发展脉络
Chat Paper
正在生成论文摘要