Comparison of Machine Learning Methods for Predicting Winter Wheat Yield in Germany.
CoRR(2021)
摘要
This study analyzed the performance of different machine learning methods for winter wheat yield prediction using extensive datasets of weather, soil, and crop phenology. To address the seasonality, weekly features were used that explicitly take soil moisture conditions and meteorological events into account. Our results indicated that nonlinear models such as deep neural networks (DNN) and XGboost are more effective in finding the functional relationship between the crop yield and input data compared to linear models. The results also revealed that the deep neural networks often had a higher prediction accuracy than XGboost. One of the main limitations of machine learning models is their black box property. As a result, we moved beyond prediction and performed feature selection, as it provides key results towards explaining yield prediction (variable importance by time). The feature selection method estimated the individual effect of weather components, soil conditions, and phenology variables as well as the time that these variables become important. As such, our study indicates which variables have the most significant effect on winter wheat yield.
更多查看译文
AI 理解论文
溯源树
样例
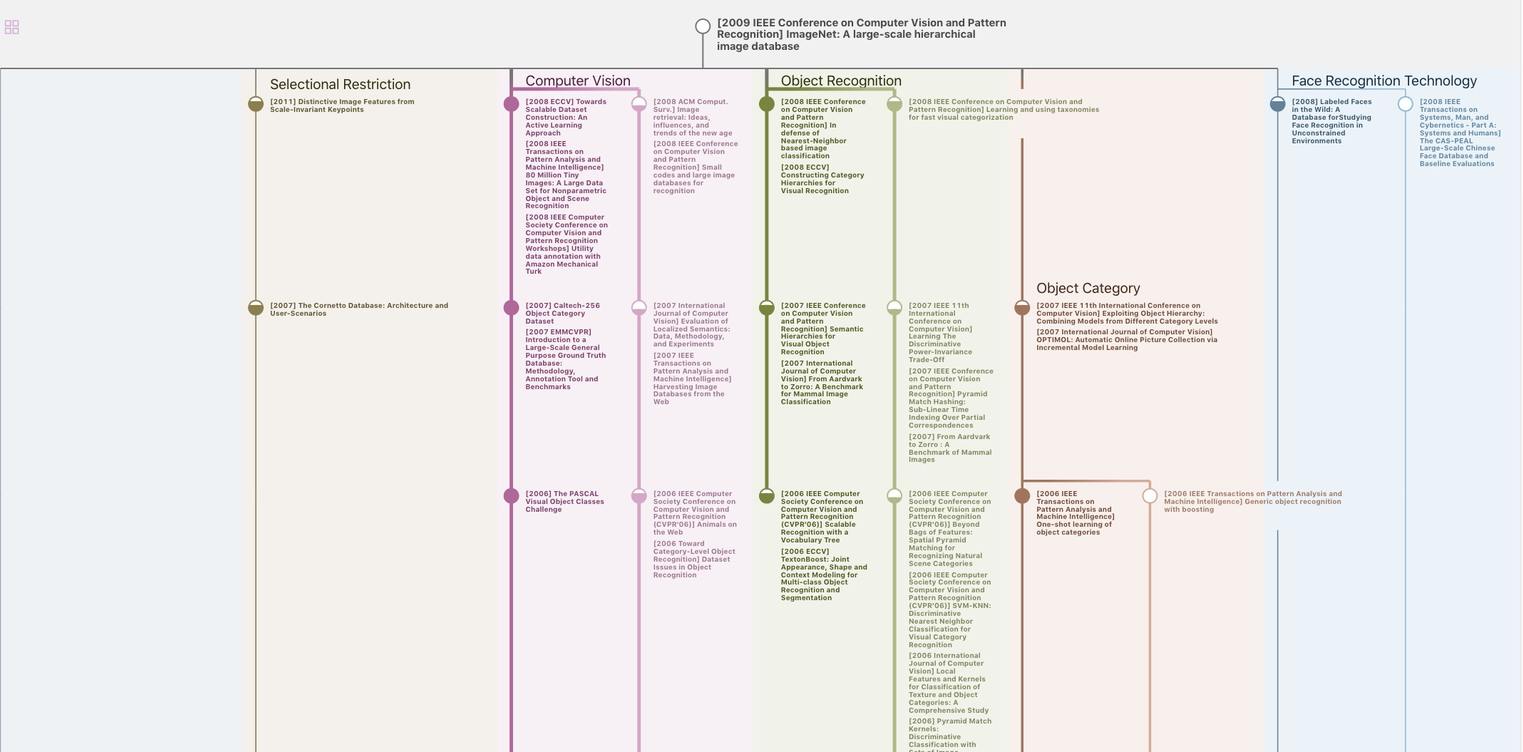
生成溯源树,研究论文发展脉络
Chat Paper
正在生成论文摘要