Class-Based Neural Network Language Model for Second-Pass Rescoring in ASR
INTERSPEECH 2021(2021)
摘要
Language model rescoring, especially neural network language model (NNLM) rescoring, is widely used to achieve improved performance in a second-pass automatic speech recognition (ASR) system. The rescoring NNLM is usually trained separately from the ASR system. Typically, the two's training corpora are different, leading to the vocabulary mismatch problem, consequently degrading ASR performance. Previous research focuses more on the language domain mismatch problem, while the vocabulary mismatch problem, which may also cause significant performance degradation, has not been well studied. This paper proposes a novel class-based NNLM framework to address the vocabulary mismatch problem for language model rescoring. Here, OOV words (unknown words to the rescoring NNLM are called OOV words for short) are assigned to well-trained classes of NNLM and inherit the class probability. Experiments show that class-based NNLM rescoring can significantly reduce performance degradation due to vocabulary mismatch.
更多查看译文
关键词
neural network language model,second-pass rescoring,class-based softmax,vocabulary mismatch,parameter estimation
AI 理解论文
溯源树
样例
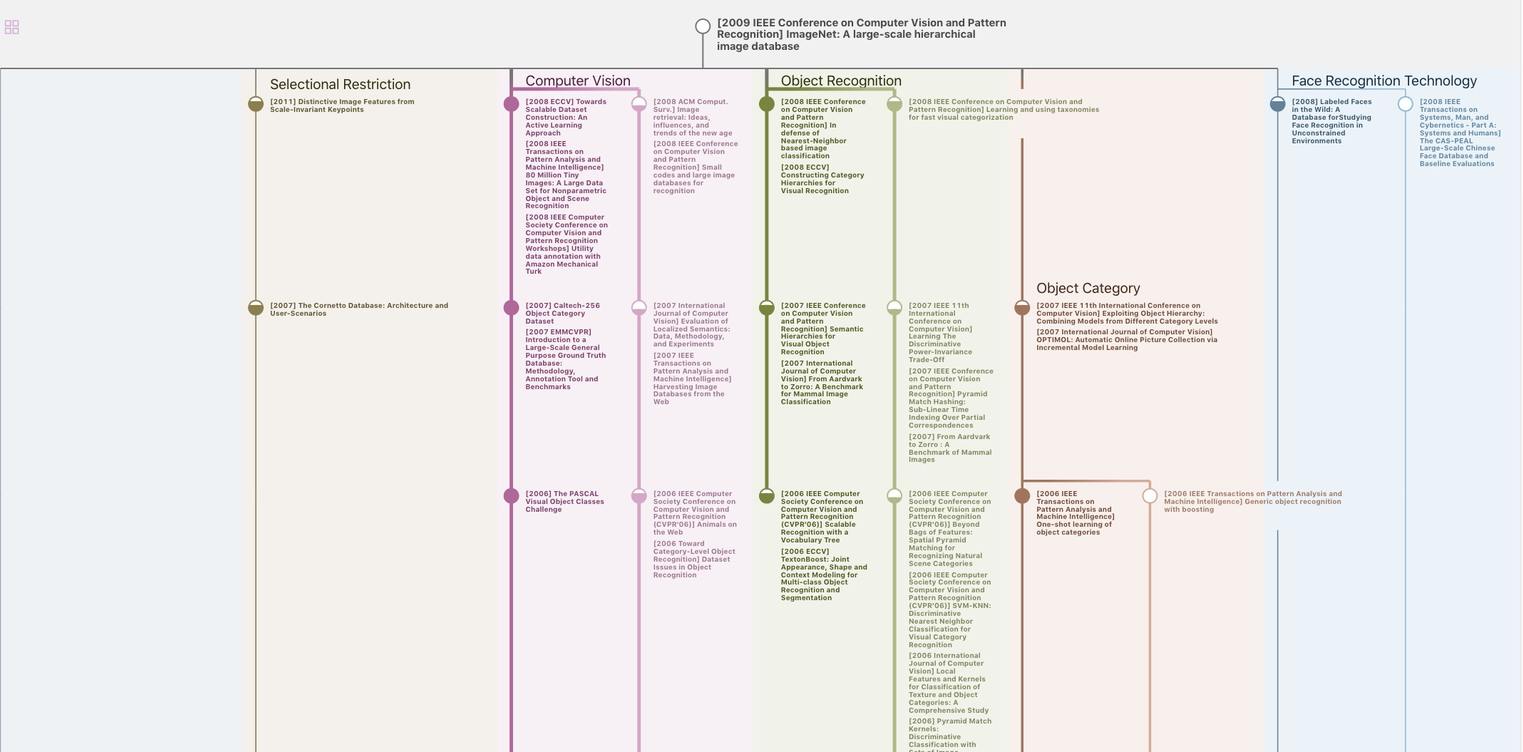
生成溯源树,研究论文发展脉络
Chat Paper
正在生成论文摘要