Data-driven Transient Stability Assessment Using Sparse PMU Sampling and Online Self-check Function
user-61447a76e55422cecdaf7d19(2023)
摘要
Artificial intelligence technologies provide a new approach for the real-time transient stability assessment (TSA) of large-scale power systems. In this paper, we propose a data-driven transient stability assessment model (DTSA) that combines different AI algorithms. A pre-AI based on the time-delay neural network is designed to locate the dominant buses for installing the phase measurement units (PMUs) and reducing the data dimension. A post-AI is designed based on the bidirectional long-short-term memory network to generate an accurate TSA with sparse PUM sampling. An online self-check function of the online TSA's validity when the power system changes is further added by comparing the results of the pre-AI and the post-AI. The IEEE 39-bus system and the 300-bus AC/DC hybrid system established by referring to China's existing power system are adopted to verify the proposed method. Results indicate that the proposed method can effectively reduce the computation costs with ensured TSA accuracy as well as provide feedback for its applicability. The DTSA provides new insights for properly integrating varied AI algorithms to solve practical problems in modern power systems.
更多查看译文
关键词
Power system stability,Phasor measurement units,Transient analysis,Training,Stability criteria,Satellite broadcasting,Feature extraction,Artificial intelligence,phasor measurement units,recurrent neural networks,transient stability assessment
AI 理解论文
溯源树
样例
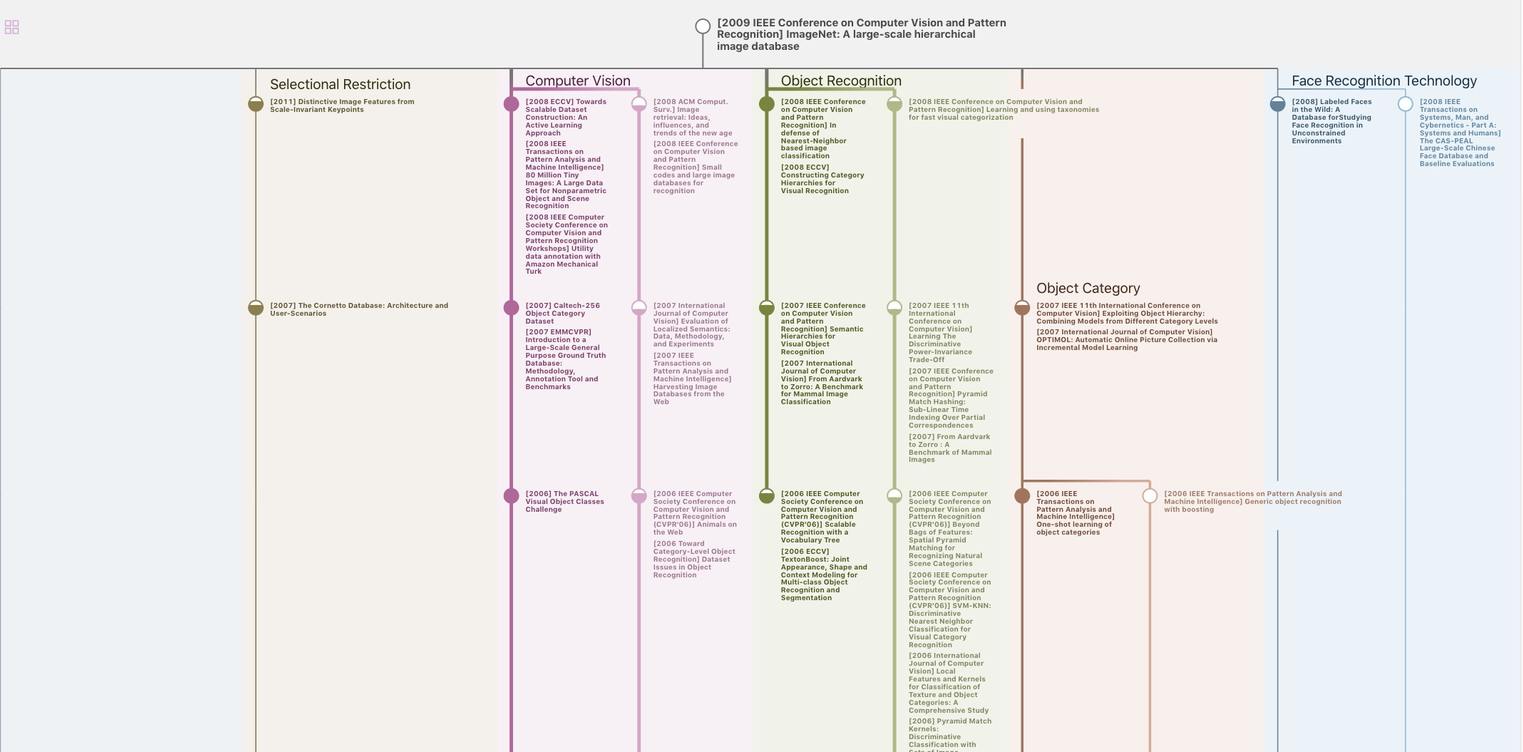
生成溯源树,研究论文发展脉络
Chat Paper
正在生成论文摘要