In-memory sparse matrix multiplication with a low-power self-rectifying memristor array
user-61447a76e55422cecdaf7d19(2022)
摘要
Abstract Memristor-enabled in-memory computing provides an unconventional computing paradigm to surpass the energy efficiency of von Neumann computers. However, owing to the physical limitation of the crossbar structure, although the memristor array is desirable for dense computation, it suffers from significant performance degradation in both energy and area efficiency when processing sparse linear algebra operations. In this work, we report a highly efficient in-memory sparse computing system based on the self-rectifying memristor, which originates from the joint effort of devices and algorithms and is used to solve computational modelling problems. This system is expected to have 74.9 – 19.6 TOPS / W energy efficiency for 2-bit to 8-bit sparse computation in computational modelling tasks. Compared to the previous in-memory computing hardware, our system provides over one order of magnitude improvement in energy efficiency with more than two orders of magnitude reduction in hardware overhead. This work could pave the road towards a highly efficient, unconventional computing solution for high-performance computing.
更多查看译文
关键词
memristor,in-memory,low-power,self-rectifying
AI 理解论文
溯源树
样例
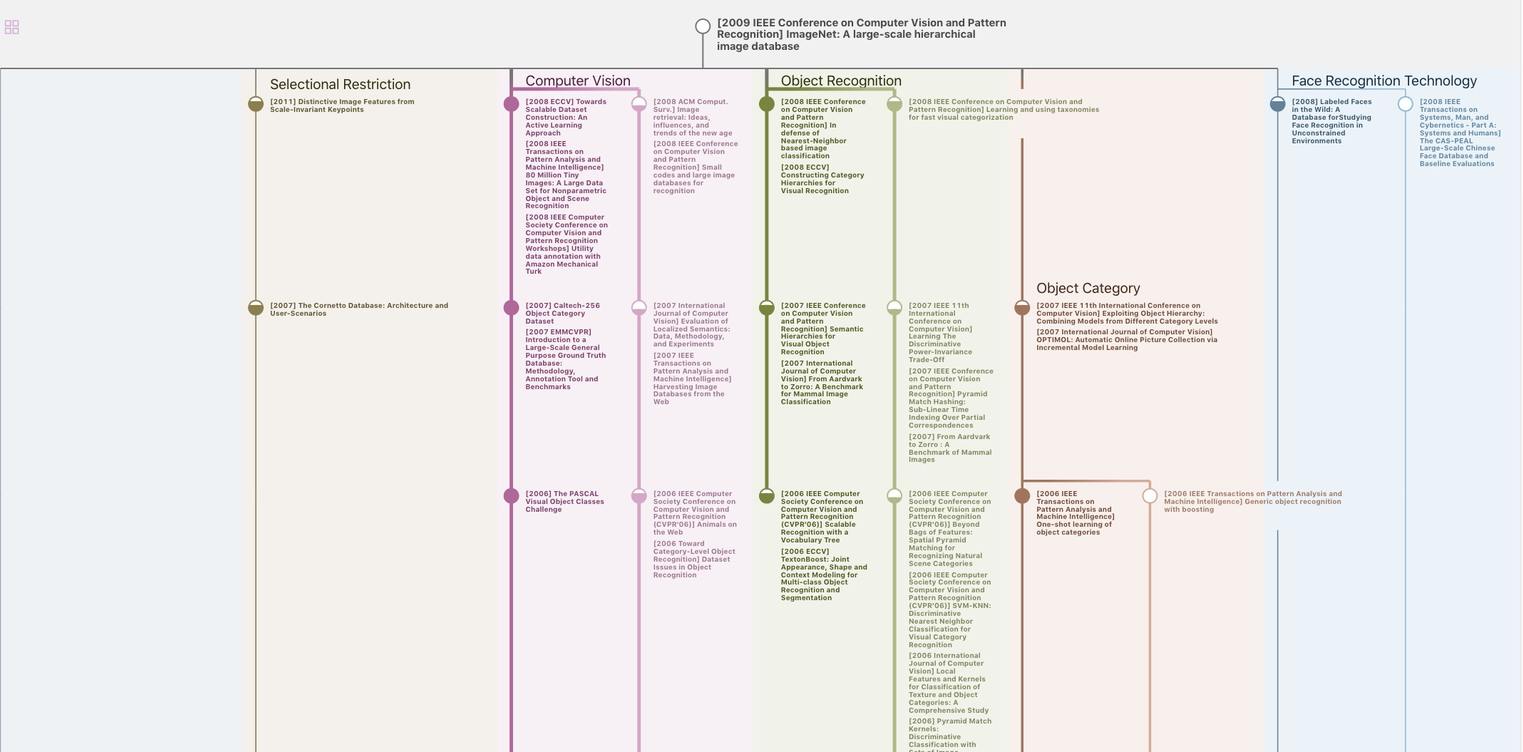
生成溯源树,研究论文发展脉络
Chat Paper
正在生成论文摘要