A multi-feature fusion transfer learning method for displacement prediction of rainfall reservoir-induced landslide with step-like deformation characteristics
Engineering Geology(2022)
摘要
Rainfall reservoir-induced landslides in the Zigui Basin, China Three Gorges Reservoir (CTGR) area, exhibit typical step-like deformation characteristics with mutation and creep states. Previous landslide displacement forecasting models yielded low prediction accuracy especially for mutational displacements. Coupled with the lack of monitoring sites and data limitations, it is extremely difficult to obtain accurate and reliable early warnings for landslides. The multi-feature fusion transfer learning (MFTL) method proposed in this paper applies the knowledge and skills obtained from the Baijiabao landslide scenario and sufficient monitoring data to improve the prediction capacity for other landslides, such as the Bazimen and Baishuihe landslides. The model barely relies on the long-time continuous monitoring process, and it can not only fill gaps in data when monitoring is interrupted, but also provide real-time displacement predictions based on accurate weather forecasting and periodic reservoir scheduling. In addition, the non-uniform weight error (NWE) evaluation method is proposed in this paper to focus more on the mutation state prediction accuracy because landslide instability is most likely to occur in this stage. Compared with other intelligent algorithms, the results indicate that the MFTL method owns low prediction error and high reliability, as well as the positive generalization ability in landslide prediction. This study paves the potential way for realizing the real-time, whole-process and accurate landslide forecasting.
更多查看译文
关键词
Landslide evolution state,Landslide deformation state,Multi-feature fusion transfer learning (MFTL) method,Displacement prediction,Step-like deformation characteristics
AI 理解论文
溯源树
样例
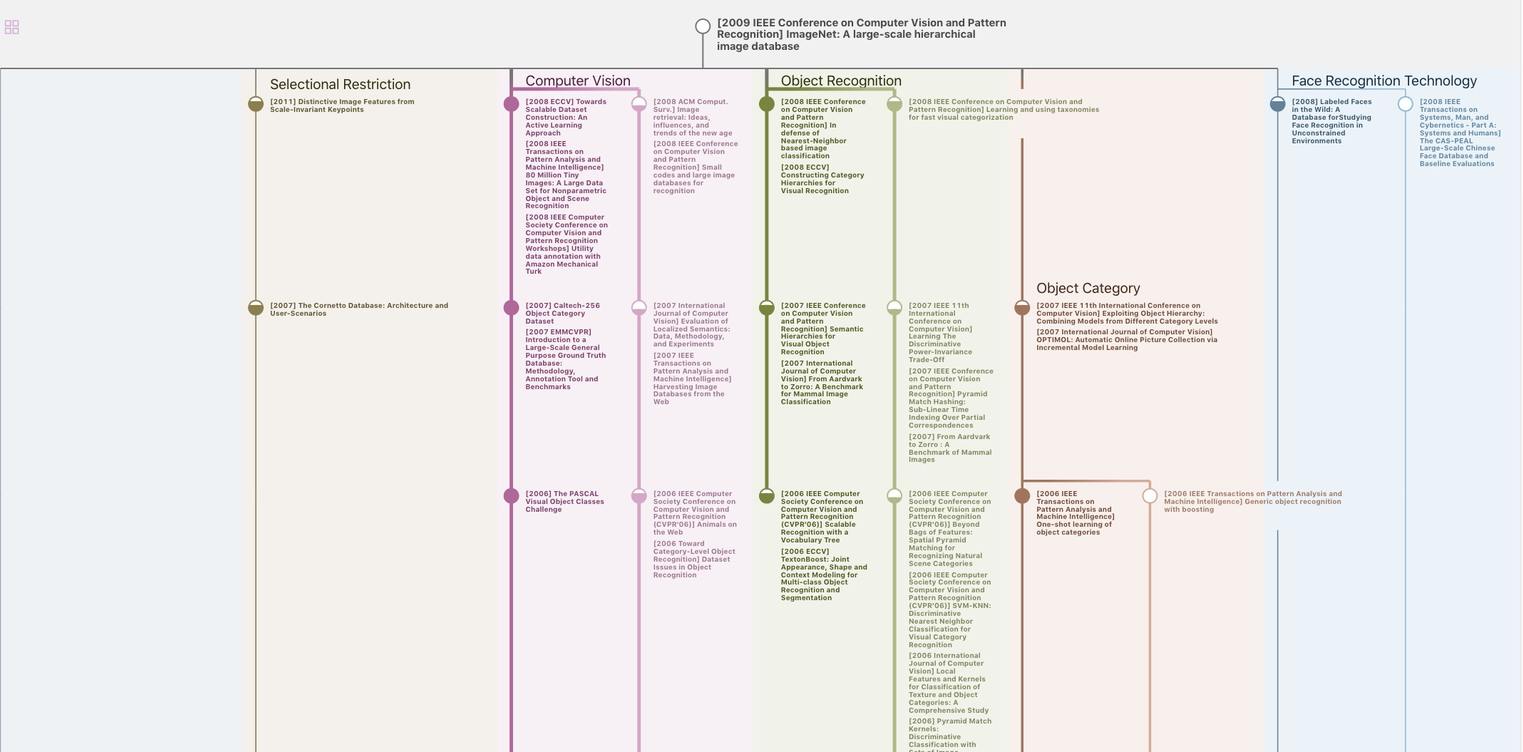
生成溯源树,研究论文发展脉络
Chat Paper
正在生成论文摘要