Incomplete Multiview Clustering With Cross-View Feature Transformation
IEEE Transactions on Artificial Intelligence(2022)
摘要
Incomplete multiview clustering (IMC) has attracted considerable attention as it can flexibly fuse the multiview information when part of the view samples are unobserved. Considering that the main challenge of IMC is the unobserved samples causing the information loss, in this article, we propose a novel IMC model to complete the unobserved samples, named cross-view feature transformation-based incomplete multiview clustering (CFTIMC). Unlike the previous completion methods that simply complete the unobserved samples with the average value or involve a third subspace, CFTIMC directly models the alignment relation between views by the cross-view feature transformation for completion, where only the overlapping views and its overlapping parts are selected for avoiding the affection of the nonoverlapping parts. Simultaneously, this completion process is integrated into multiview graph learning, where they can boost each other. To solve the proposed model, we develop an alternate optimization scheme-based iterative algorithm, together with its computational cost analysis and convergence analysis. Finally, the clustering experiments on several benchmark datasets verify that our method can outperform the related state-of-the-art methods.
更多查看译文
关键词
Completion,cross-view feature transformation,incomplete multiview clustering (IMC)
AI 理解论文
溯源树
样例
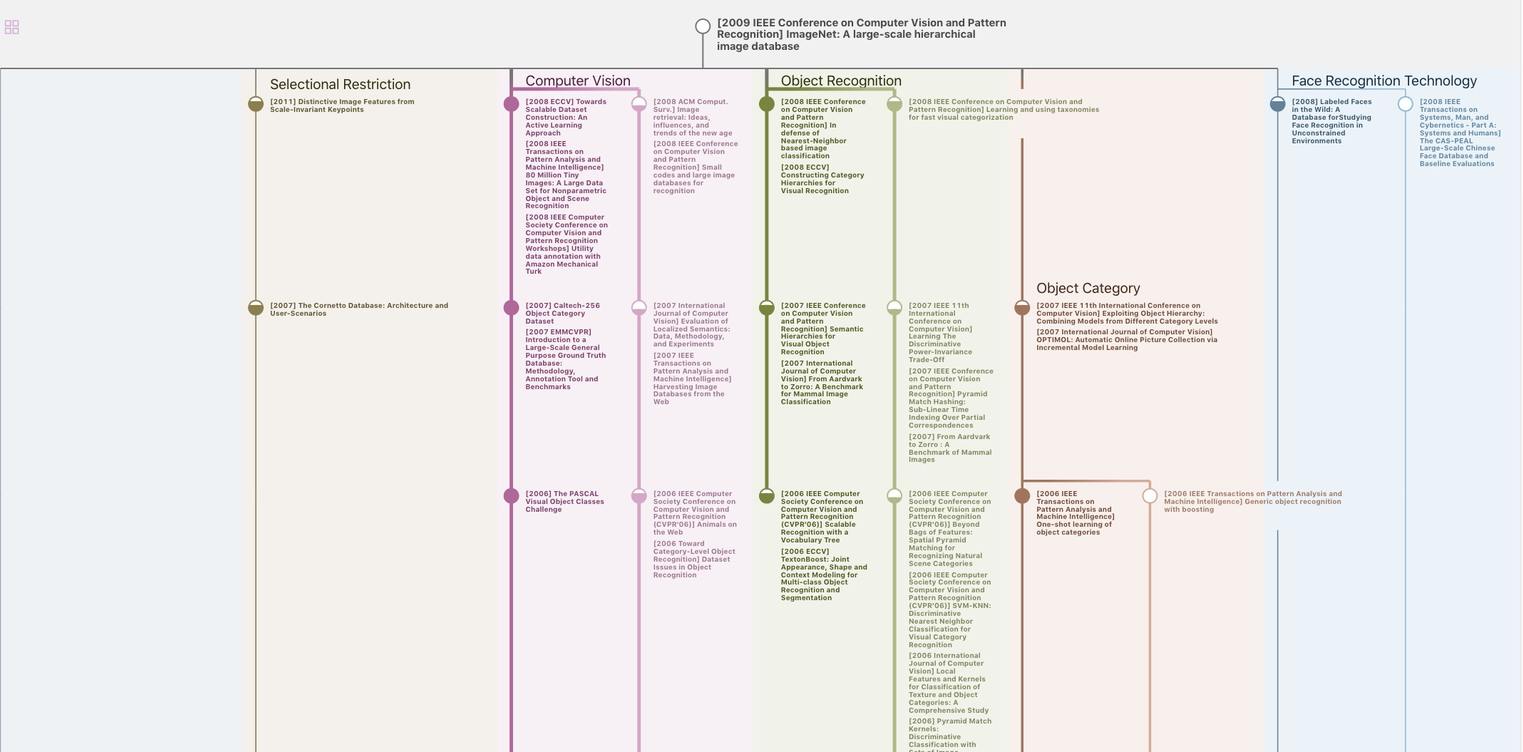
生成溯源树,研究论文发展脉络
Chat Paper
正在生成论文摘要