Graph Convolution Based Residual Connected Network for Morphological Reconstruction in Fluorescence Molecular Tomography
MEDICAL IMAGING 2022: BIOMEDICAL APPLICATIONS IN MOLECULAR, STRUCTURAL, AND FUNCTIONAL IMAGING(2022)
摘要
Fluorescence molecular tomography (FMT) is a promising multimodality-fused medical imaging technique, aiming at noninvasively and dynamically visualizing the interaction processes at the cellular and molecular level. However, the quality of FMT reconstruction is limited by the simplified linear model of photon propagation. In this work, we propose a novel GCN based Residual connected (GCN-RC) network to improve the quality of FMT morphological reconstruction. Instead of using a simplified linear model of photon propagation for FMT recon-struction, the method can directly construct a nonlinear mapping relationship between the photon density of an object surface and its internal fluorescent source. GCN-RC network consists of a fully connected(FC) sub-network and a GCN sub-network connected by means of residual connection. The FC sub-network provides a coarse reconstruction result and GCN sub-network fine-tunes the morphological quality of reconstructed result. In order to validate the reconstruction performance of GCN-RC, we performed numerical simulation experiments and in vivo experiments based on tumor-bearing mice. Comparisons were performed with the L2-based Tikhonov method (Tikhonov-L2), inverse problem simulation (IPS) method and GCN- RC method. Both numerical simulated and in vivo experimental results demonstrated that GCN-RC achieved improved reconstruction in terms of both source localization and morphology recovery.
更多查看译文
关键词
fluorescence molecular tomography, Graph convolution network, inverse problem
AI 理解论文
溯源树
样例
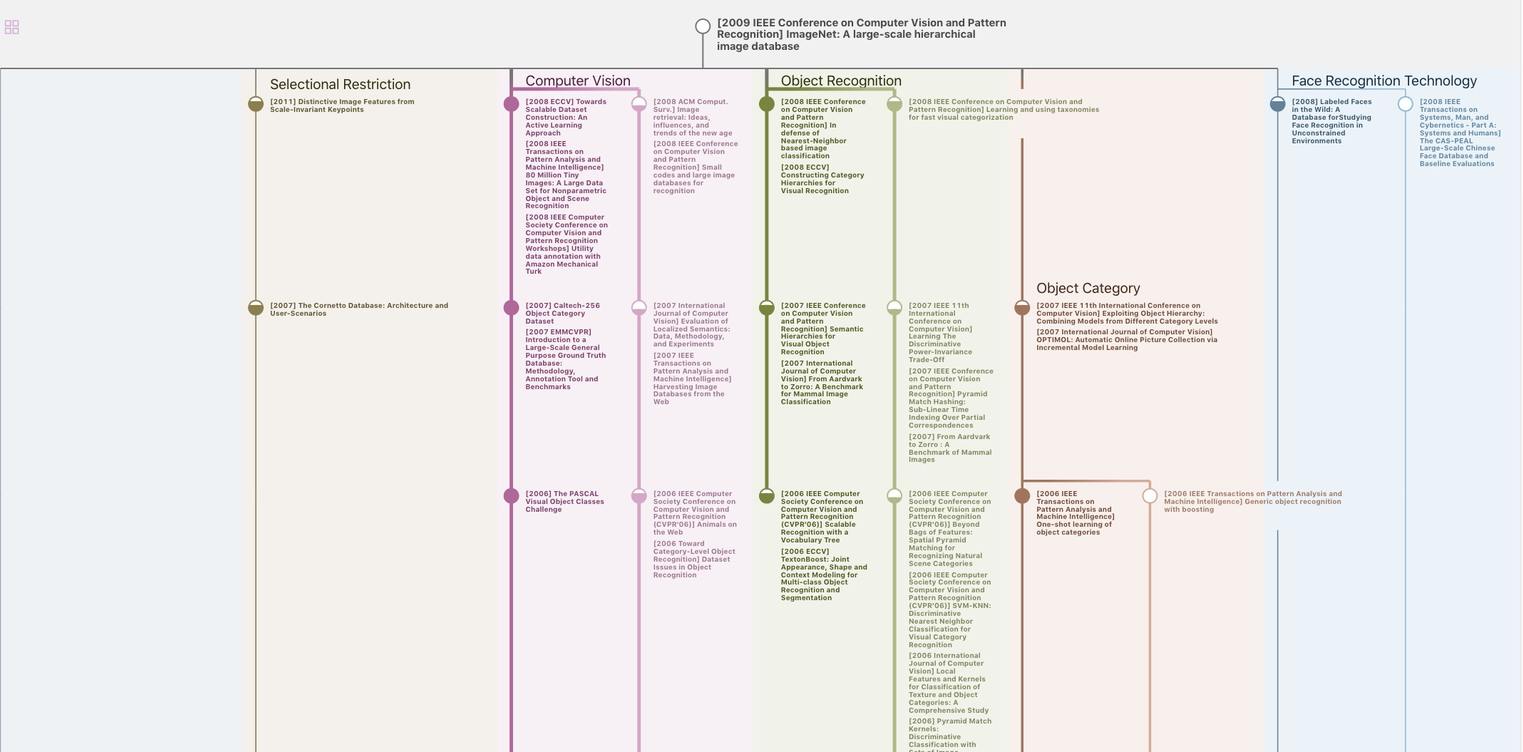
生成溯源树,研究论文发展脉络
Chat Paper
正在生成论文摘要