Privacy-Preserving Federated Learning based on Differential Privacy and Momentum Gradient Descent
IEEE International Joint Conference on Neural Network (IJCNN)(2022)
摘要
To preserve participants' privacy, Federated Learning (FL) has been proposed to let participants collaboratively train a global model by sharing their training gradients instead of their raw data. However, several studies have shown that conventional FL is insufficient to protect privacy from adversaries, as even from gradients, useful information can still be recovered. To obtain stronger privacy protection, Differential Privacy (DP) has been proposed on the server's side and the clients' side. Although adding artificial noise to the raw data can enhance users' privacy, the accuracy performance of the FL is inevitably degraded. In addition, although the communication overhead caused by the FL is much smaller than that of centralized learning, it still becomes a bottleneck of the learning performance and utilization efficiency due to its frequent parameters exchange. To tackle these problems, we propose a new FL framework via applying DP both locally and centrally in order to strengthen the protection of participants' privacy. To improve the accuracy performance of the model, we also apply sparse gradients and Momentum Gradient Descent on the server's side and the clients' side. Moreover, using sparse gradients can reduce the total communication costs. We provide the experiments to evaluate our proposed framework and the results show that our framework not only outperforms other DP-based FL frameworks in terms of the model accuracy but also provides a more powerful privacy guarantee. Besides, our framework can save up to 90% of communication costs while achieving the best accuracy performance.
更多查看译文
关键词
Privacy-preserving federated learning,differential privacy,momentum gradient descent,gradients sparsification
AI 理解论文
溯源树
样例
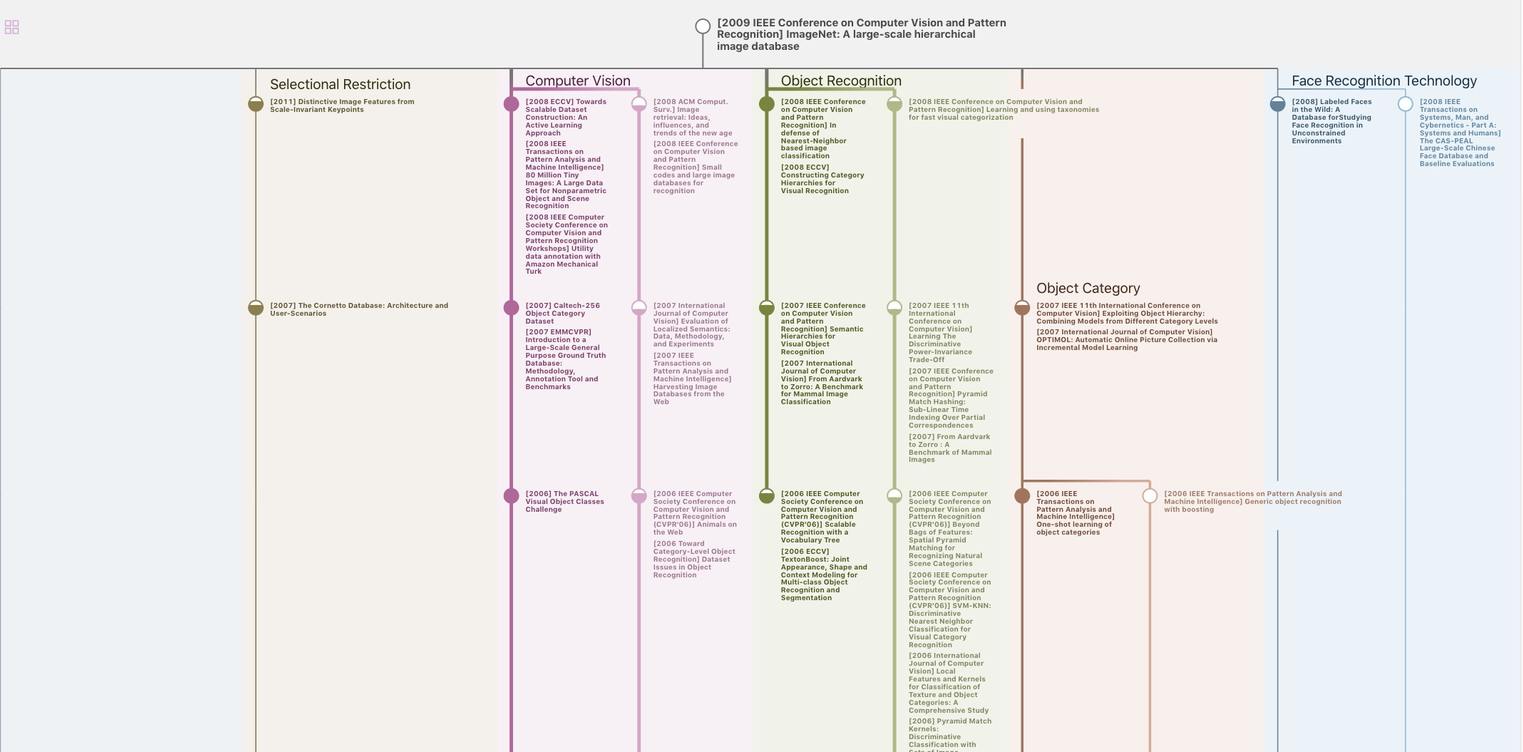
生成溯源树,研究论文发展脉络
Chat Paper
正在生成论文摘要