WT-MVSNet: Window-based Transformers for Multi-view Stereo
NeurIPS 2022(2022)
摘要
Recently, Transformers were shown to enhance the performance of multi-view stereo by enabling long-range feature interaction. In this work, we propose Window-based Transformers (WT) for local feature matching and global feature aggregation in multi-view stereo. We introduce a Window-based Epipolar Transformer (WET) which reduces matching redundancy by using epipolar constraints. Since point-to-line matching is sensitive to erroneous camera pose and calibration, we match windows near the epipolar lines. A second Shifted WT is employed for aggregating global information within cost volume. We present a novel Cost Transformer (CT) to replace 3D convolutions for cost volume regularization. In order to better constrain the estimated depth maps from multiple views, we further design a novel geometric consistency loss (Geo Loss) which punishes unreliable areas where multi-view consistency is not satisfied. Our WT multi-view stereo method (WT-MVSNet) achieves state-of-the-art performance across multiple datasets and ranks $1^{st}$ on Tanks and Temples benchmark.
更多查看译文
关键词
transformers,wt-mvsnet,window-based,multi-view
AI 理解论文
溯源树
样例
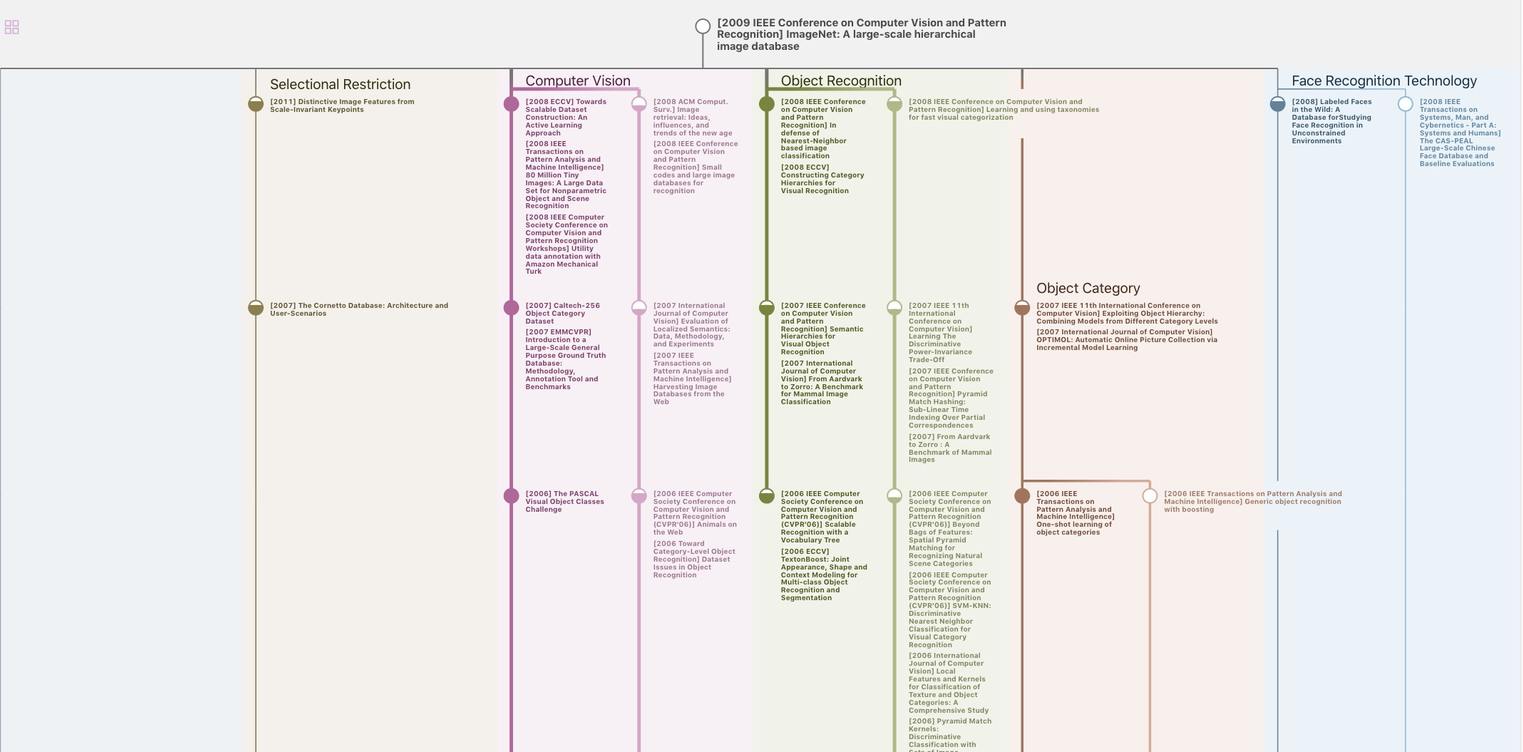
生成溯源树,研究论文发展脉络
Chat Paper
正在生成论文摘要