Differentiable Point-Based Radiance Fields for Efficient View Synthesis.
SIGGRAPH Asia(2022)
摘要
We propose a differentiable rendering algorithm for efficient novel view synthesis. By departing from volume-based representations in favor of a learned point representation, we improve on existing methods more than an order of magnitude in memory and runtime, both in training and inference. The method begins with a uniformly-sampled random point cloud and learns per-point position and view-dependent appearance, using a differentiable splat-based renderer to evolve the model to match a set of input images. Our method is up to 300x faster than NeRF in both training and inference, with only a marginal sacrifice in quality, while using less than 10~MB of memory for a static scene. For dynamic scenes, our method trains two orders of magnitude faster than STNeRF and renders at near interactive rate, while maintaining high image quality and temporal coherence even without imposing any temporal-coherency regularizers.
更多查看译文
关键词
radiance fields,view,point-based
AI 理解论文
溯源树
样例
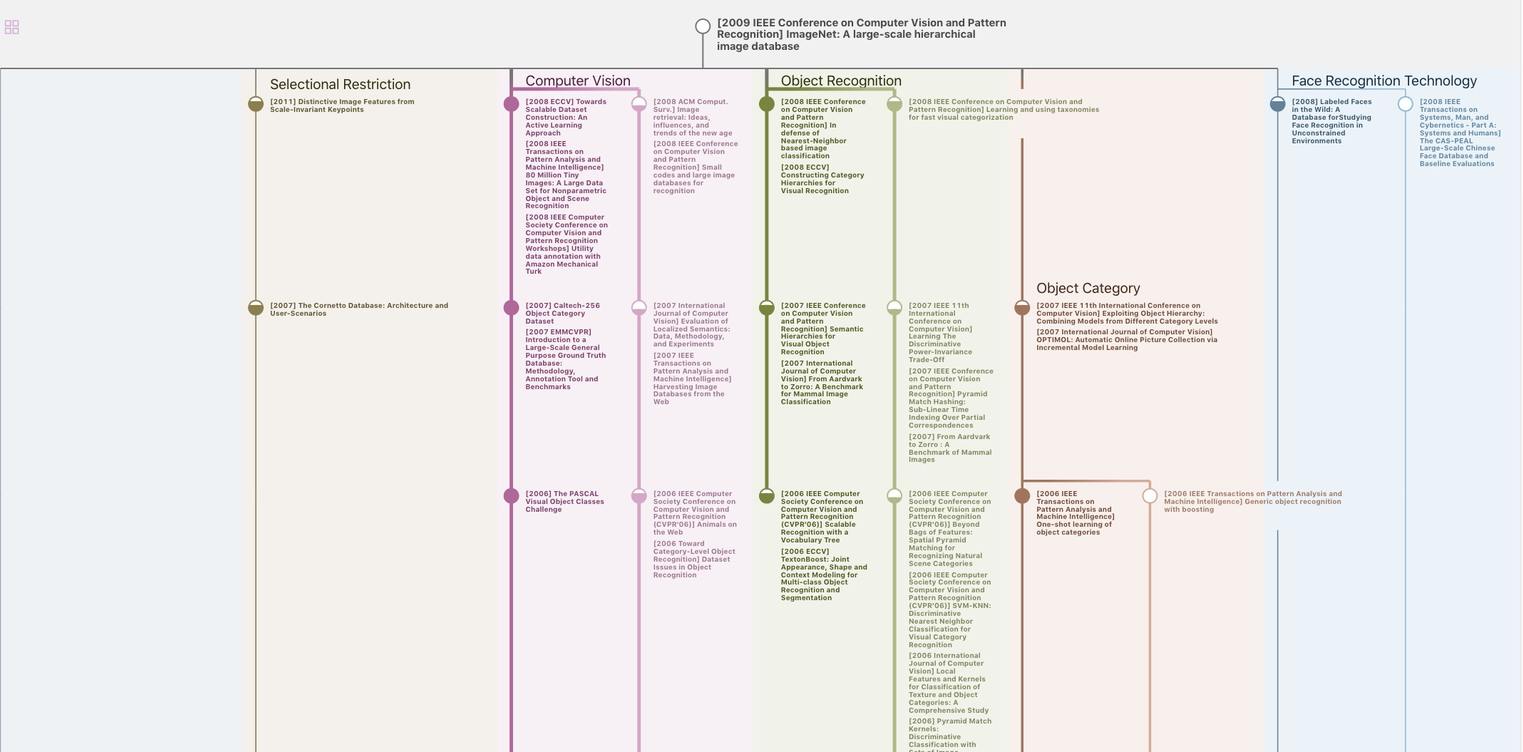
生成溯源树,研究论文发展脉络
Chat Paper
正在生成论文摘要