Quantum Multi-Armed Bandits and Stochastic Linear Bandits Enjoy Logarithmic Regrets
AAAI(2022)
摘要
Multi-arm bandit (MAB) and stochastic linear bandit (SLB) are important models in reinforcement learning, and it is well-known that classical algorithms for bandits with time horizon $T$ suffer $\Omega(\sqrt{T})$ regret. In this paper, we study MAB and SLB with quantum reward oracles and propose quantum algorithms for both models with $O(\mbox{poly}(\log T))$ regrets, exponentially improving the dependence in terms of $T$. To the best of our knowledge, this is the first provable quantum speedup for regrets of bandit problems and in general exploitation in reinforcement learning. Compared to previous literature on quantum exploration algorithms for MAB and reinforcement learning, our quantum input model is simpler and only assumes quantum oracles for each individual arm.
更多查看译文
关键词
stochastic linear bandits,quantum,multi-armed
AI 理解论文
溯源树
样例
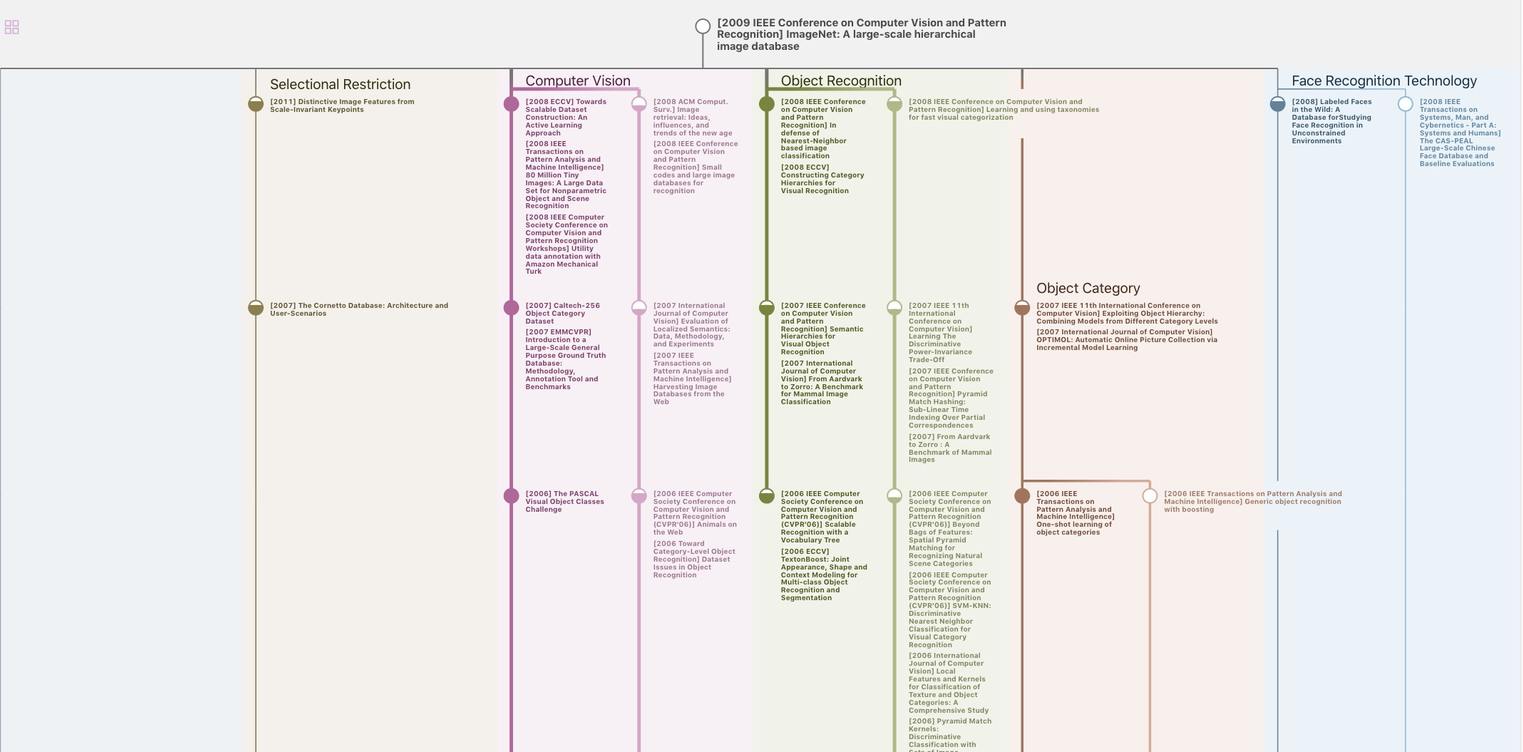
生成溯源树,研究论文发展脉络
Chat Paper
正在生成论文摘要