Robotic grasp detection based on Transformer
arxiv(2022)
摘要
Grasp detection in a cluttered environment is still a great challenge for robots. Currently, the Transformer mechanism has been successfully applied to visual tasks, and its excellent ability of global context information extraction provides a feasible way to improve the performance of robotic grasp detection in cluttered scenes. However, the insufficient inductive bias ability of the original Transformer model requires large-scale datasets training, which is difficult to obtain for grasp detection. In this paper, we propose a grasp detection model based on encoder-decoder structure. The encoder uses a Transformer network to extract global context information. The decoder uses a fully convolutional neural network to improve the inductive bias capability of the model and combine features extracted by the encoder to predict the final grasp configuration. Experiments on the VMRD dataset demonstrate that our model performs much better in overlapping object scenes. Meanwhile, on the Cornell Grasp dataset, our approach achieves an accuracy of 98.1%, which is comparable with state-of-the-art algorithms.
更多查看译文
关键词
Grasp detection, Cluttered environment, Transformer
AI 理解论文
溯源树
样例
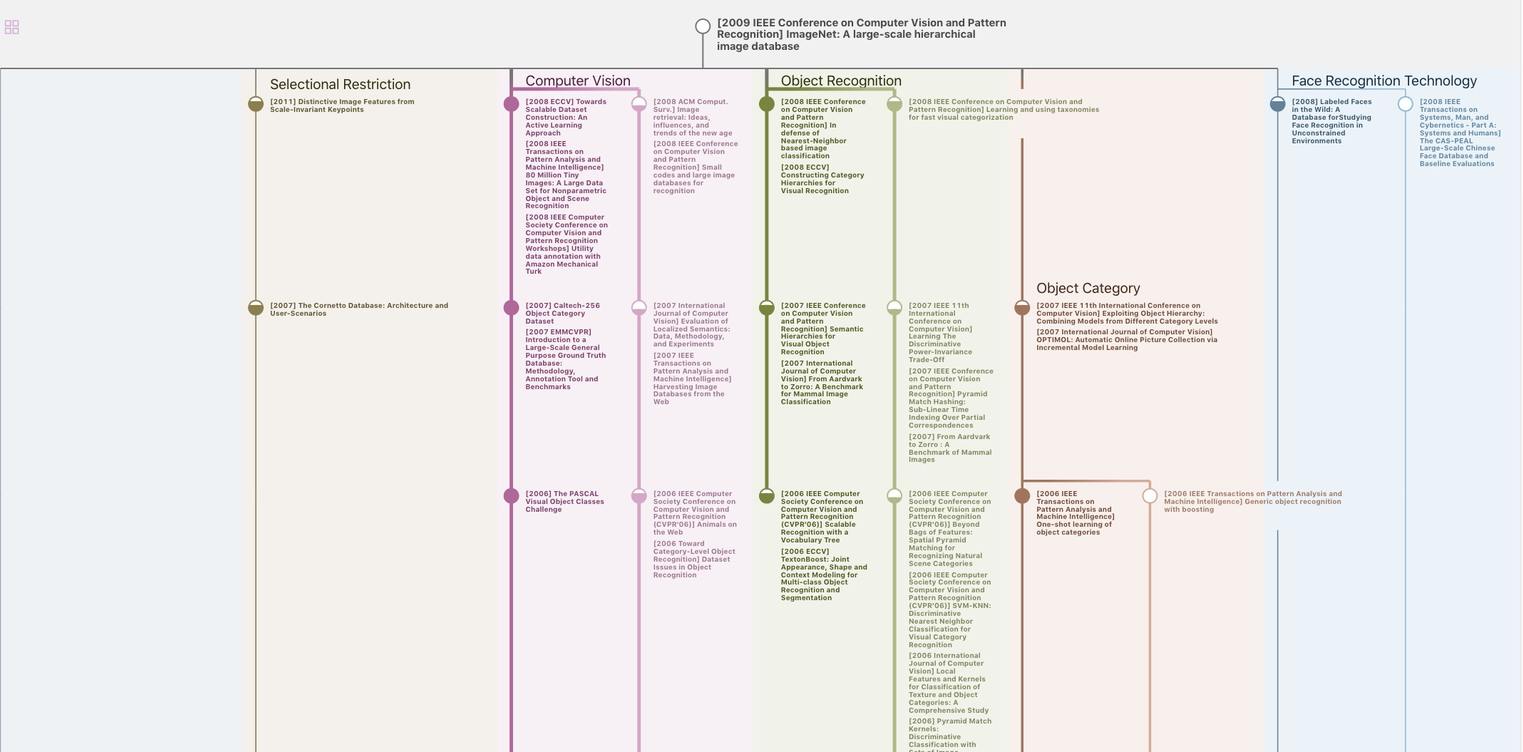
生成溯源树,研究论文发展脉络
Chat Paper
正在生成论文摘要