Principal Component Analysis based frameworks for efficient missing data imputation algorithms
arxiv(2023)
摘要
Missing data is a commonly occurring problem in practice. Many imputation methods have been developed to fill in the missing entries. However, not all of them can scale to high-dimensional data, especially the multiple imputation techniques. Meanwhile, the data nowadays tends toward high-dimensional. Therefore, in this work, we propose Principal Component Analysis Imputation (PCAI), a simple but versatile framework based on Principal Component Analysis (PCA) to speed up the imputation process and alleviate memory issues of many available imputation techniques, without sacrificing the imputation quality in term of MSE. In addition, the frameworks can be used even when some or all of the missing features are categorical, or when the number of missing features is large. Next, we introduce PCA Imputation - Classification (PIC), an application of PCAI for classification problems with some adjustments. We validate our approach by experiments on various scenarios, which shows that PCAI and PIC can work with various imputation algorithms, including the state-of-the-art ones and improve the imputation speed significantly, while achieving competitive mean square error/classification accuracy compared to direct imputation (i.e., impute directly on the missing data).
更多查看译文
关键词
efficient missing data
AI 理解论文
溯源树
样例
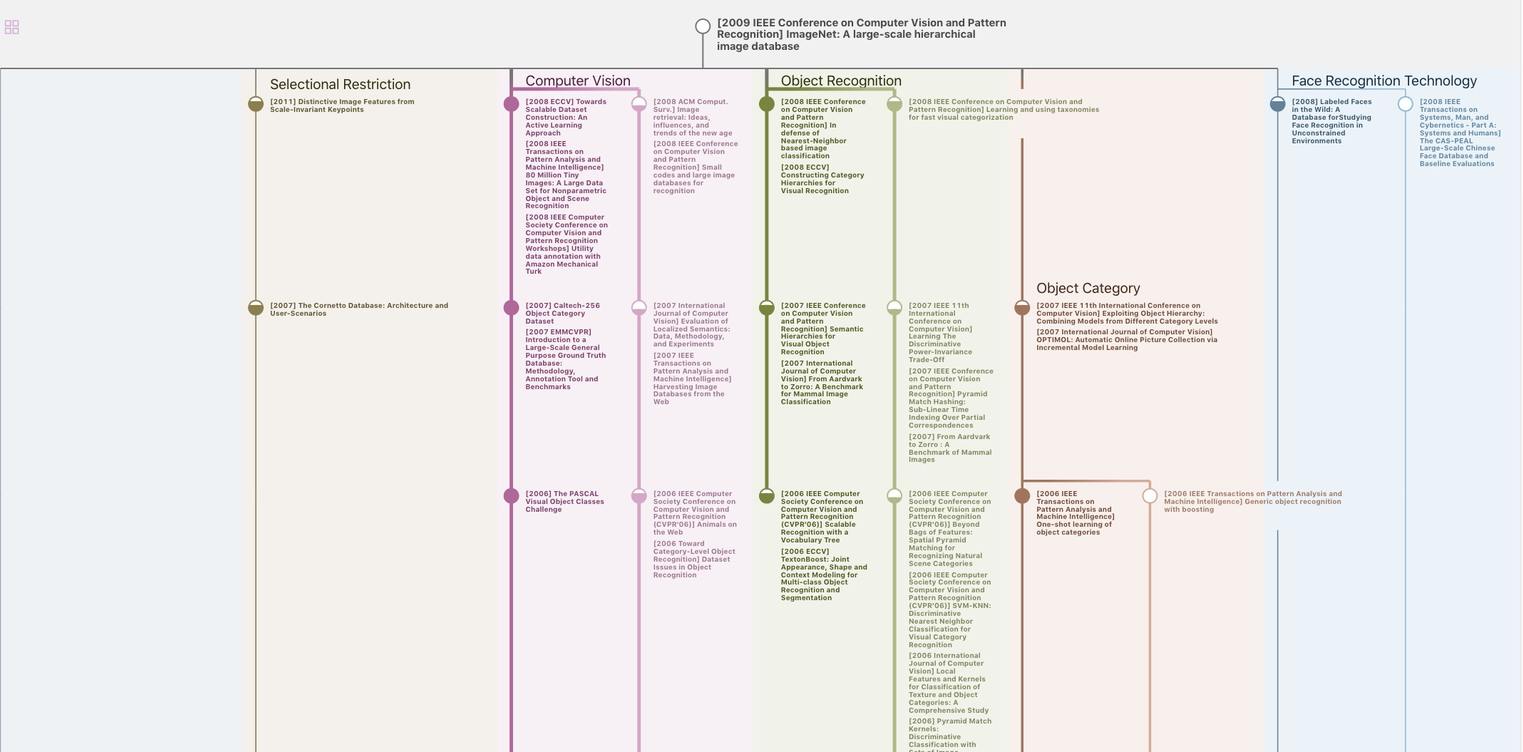
生成溯源树,研究论文发展脉络
Chat Paper
正在生成论文摘要