Flowification: Everything is a Normalizing Flow
NeurIPS 2022(2022)
摘要
We develop a method that can be used to calculate the likelihood contribution of linear and convolutional layers allowing multi-layer perceptrons and convolutional networks to be converted into normalizing flows. We term this process flowification. In some cases flowification requires the addition of uncorrelated noise to the model but in the simplest case no additional parameters. The technique we develop can be applied to a broad range of architectures, allowing them to be used for a wide range of tasks. Our models also allow existing density estimation techniques to be combined with high performance feature extractors. In contrast to standard density estimation techniques that require specific architectures and specialized knowledge, our approach can leverage design knowledge from different domains and is a step closer to the realization of general purpose architectures. We investigate the efficacy of linear and convolutional layers for the task of density estimation on standard datasets.
更多查看译文
关键词
Normalizing flows
AI 理解论文
溯源树
样例
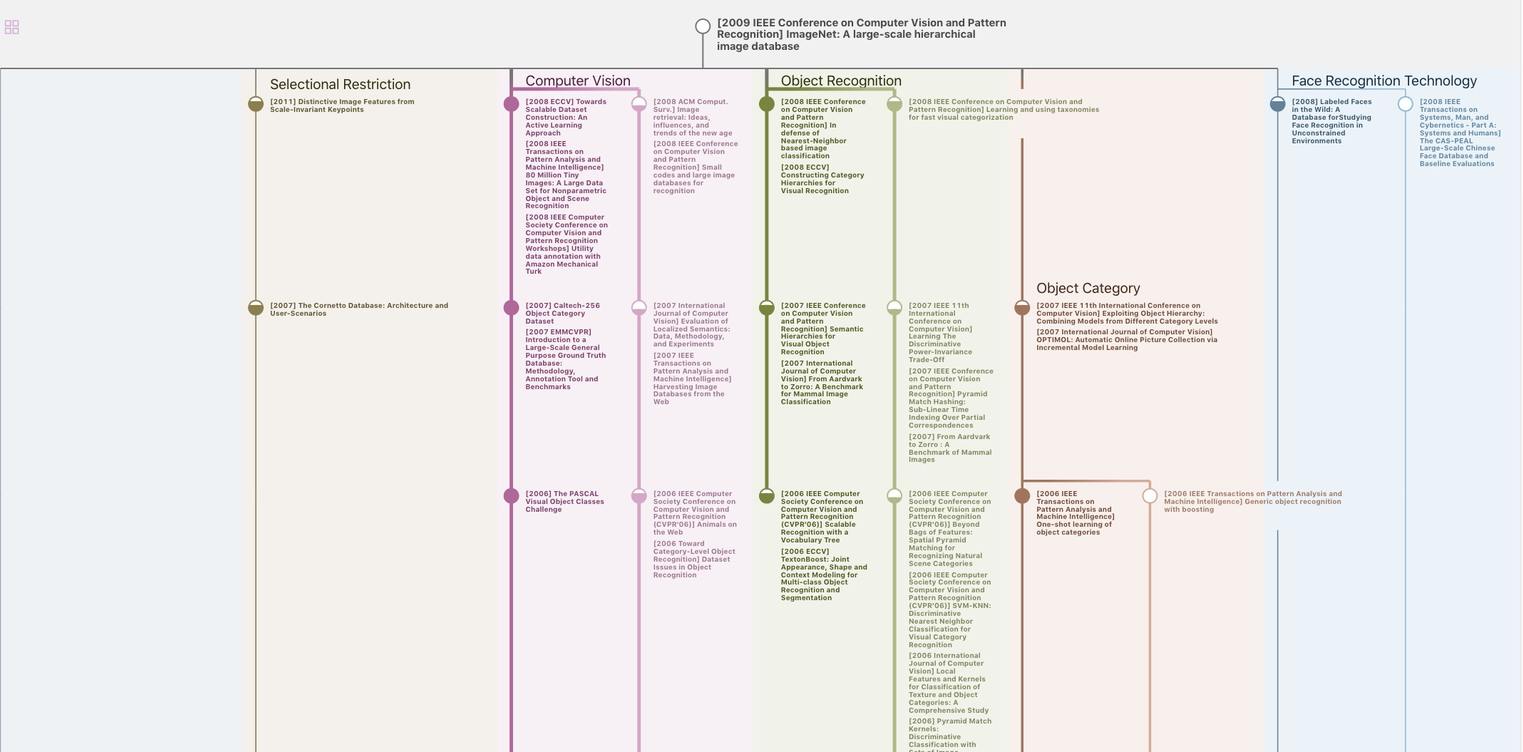
生成溯源树,研究论文发展脉络
Chat Paper
正在生成论文摘要