NIR Instruments and Prediction Methods for Rapid Access to Grain Protein Content in Multiple Cereals
SENSORS(2022)
摘要
Achieving global goals for sustainable nutrition, health, and wellbeing will depend on delivering enhanced diets to humankind. This will require instantaneous access to information on food-source quality at key points of agri-food systems. Although laboratory analysis and benchtop NIR spectrometers are regularly used to quantify grain quality, these do not suit all end users, for example, stakeholders in decentralized agri-food chains that are typical in emerging economies. Therefore, we explored benchtop and portable NIR instruments, and the methods that might aid these particular end uses. For this purpose, we generated NIR spectra for 328 grain samples from multiple cereals (finger millet, foxtail millet, maize, pearl millet, and sorghum) with a standard benchtop NIR spectrometer (DS2500, FOSS) and a novel portable NIR-based instrument (HL-EVT5, Hone). We explored classical deterministic methods (via winISI, FOSS), novel machine learning (ML)-driven methods (via Hone Create, Hone), and a convolutional neural network (CNN)-based method for building the calibrations to predict grain protein out of the NIR spectra. All of the tested methods enabled us to build relevant calibrations out of both types of spectra (i.e., R-2 >= 0.90, RMSE <= 0.91, RPD >= 3.08). Generally, the calibration methods integrating the ML techniques tended to enhance the prediction capacity of the model. We also documented that the prediction of grain protein content based on the NIR spectra generated using the novel portable instrument (HL-EVT5, Hone) was highly relevant for quantitative protein predictions (R-2 = 0.91, RMSE = 0.97, RPD = 3.48). Thus, the presented findings lay the foundations for the expanded use of NIR spectroscopy in agricultural research, development, and trade.
更多查看译文
关键词
cereals, protein, near-infrared spectroscopy (NIRS), prediction methods, winISI, Hone Create, Convolution Neural Network (CNN)
AI 理解论文
溯源树
样例
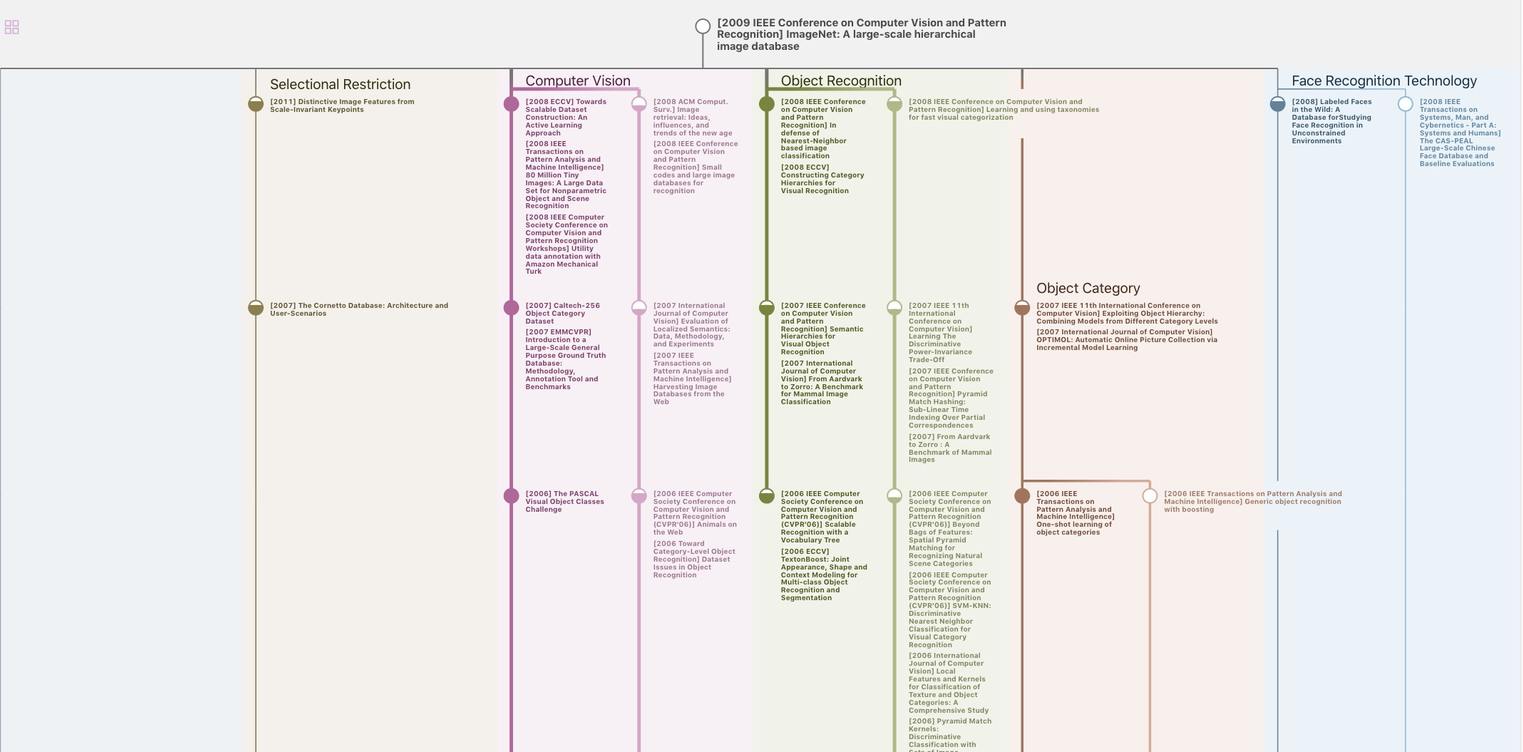
生成溯源树,研究论文发展脉络
Chat Paper
正在生成论文摘要