Critic Sequential Monte Carlo
ICLR 2023(2022)
摘要
We introduce CriticSMC, a new algorithm for planning as inference built from a novel composition of sequential Monte Carlo with learned soft-Q function heuristic factors. This algorithm is structured so as to allow using large numbers of putative particles leading to efficient utilization of computational resource and effective discovery of high reward trajectories even in environments with difficult reward surfaces such as those arising from hard constraints. Relative to prior art our approach is notably still compatible with model-free reinforcement learning in the sense that the implicit policy we produce can be used at test time in the absence of a world model. Our experiments on self-driving car collision avoidance in simulation demonstrate improvements against baselines in terms of infraction minimization relative to computational effort while maintaining diversity and realism of found trajectories.
更多查看译文
关键词
sequential monte carlo,reinforcement learning as inference,soft Q-learning,heuristic factors,driving behavior models
AI 理解论文
溯源树
样例
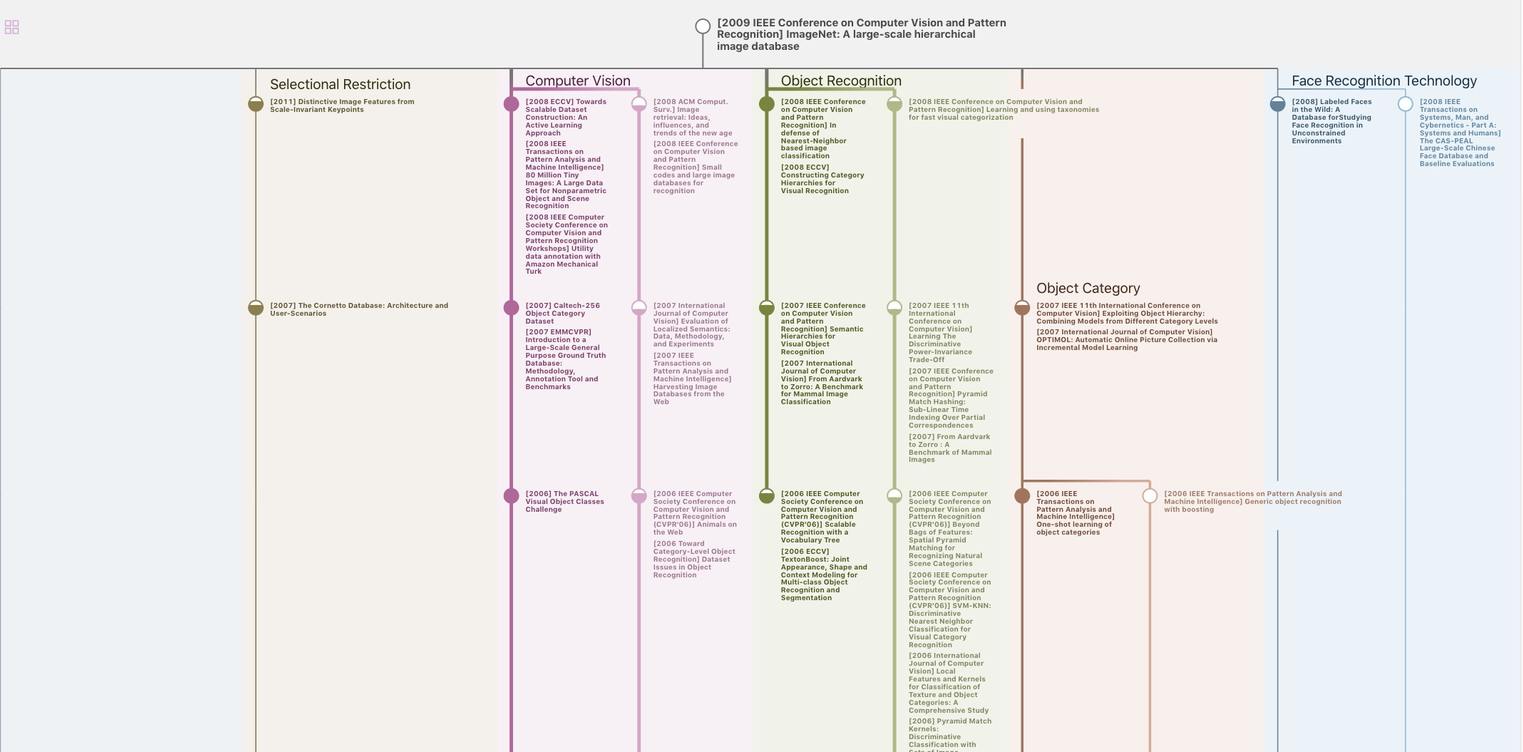
生成溯源树,研究论文发展脉络
Chat Paper
正在生成论文摘要