Compressed Hierarchical Representations for Multi-Task Learning and Task Clustering
IEEE International Joint Conference on Neural Network (IJCNN)(2022)
摘要
In this paper, we frame homogeneous-feature multi-task learning (MTL) as a hierarchical representation learning problem, with one task-agnostic and multiple task-specific latent representations. Drawing inspiration from the information bottleneck principle and assuming an additive independent noise model between the task-agnostic and task-specific latent representations, we limit the information contained in each task-specific representation. It is shown that our resulting representations yield competitive performance for several MTL benchmarks. Furthermore, for certain setups, we show that the trained parameters of the additive noise model are closely related to the similarity of different tasks. This indicates that our approach yields a task-agnostic representation that is disentangled in the sense that its individual dimensions may be interpretable from a task-specific perspective.
更多查看译文
关键词
Representation learning,multi-task learning,disentanglement,information bottleneck
AI 理解论文
溯源树
样例
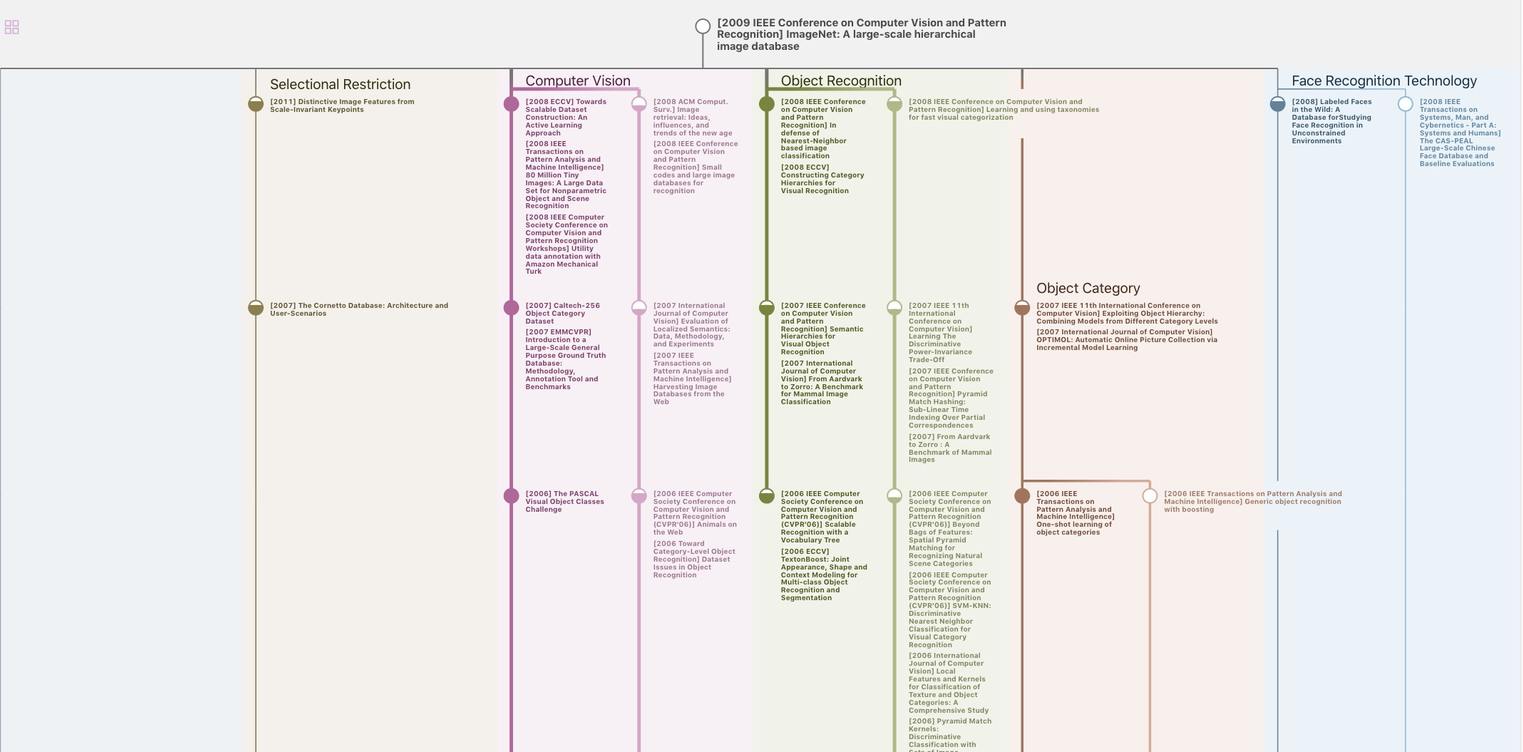
生成溯源树,研究论文发展脉络
Chat Paper
正在生成论文摘要