Social Bias Meets Data Bias: The Impacts of Labeling and Measurement Errors on Fairness Criteria
arxiv(2023)
摘要
Although many fairness criteria have been proposed to ensure that machine learning algorithms do not exhibit or amplify our existing social biases, these algorithms are trained on datasets that can themselves be statistically biased. In this paper, we investigate the robustness of a number of existing (demographic) fairness criteria when the algorithm is trained on biased data. We consider two forms of dataset bias: errors by prior decision makers in the labeling process, and errors in measurement of the features of disadvantaged individuals. We analytically show that some constraints (such as Demographic Parity) can remain robust when facing certain statistical biases, while others (such as Equalized Odds) are significantly violated if trained on biased data. We also analyze the sensitivity of these criteria and the decision maker's utility to biases. We provide numerical experiments based on three real-world datasets (the FICO, Adult, and German credit score datasets) supporting our analytical findings. Our findings present an additional guideline for choosing among existing fairness criteria, or for proposing new criteria, when available datasets may be biased.
更多查看译文
关键词
data bias,labeling,social
AI 理解论文
溯源树
样例
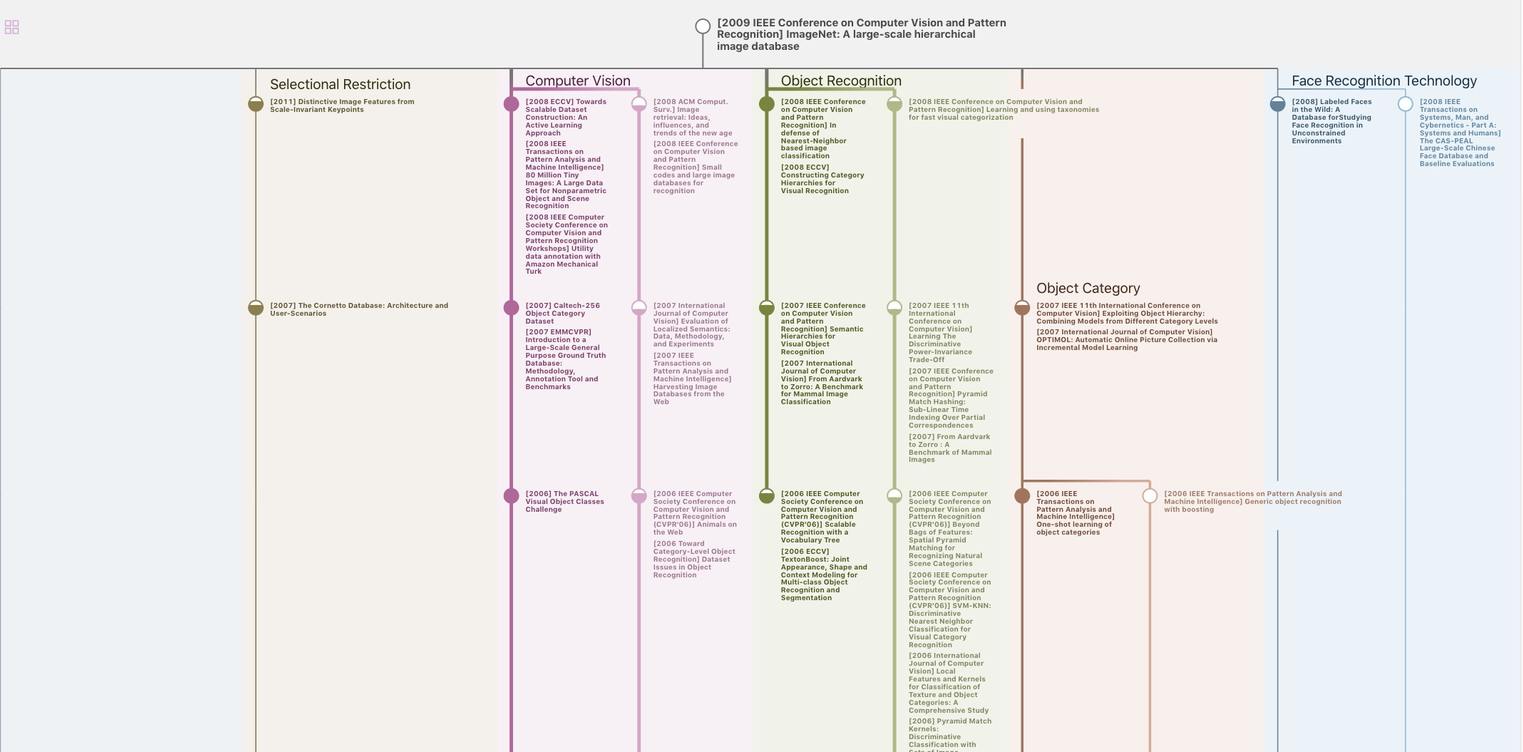
生成溯源树,研究论文发展脉络
Chat Paper
正在生成论文摘要