Cross-domain Detection Transformer based on Spatial-aware and Semantic-aware Token Alignment.
CoRR(2022)
摘要
Detection transformers such as DETR [1] have recently exhibited promising performance for many object detection tasks, but the generalization ability of those methods is still quite limited for cross-domain adaptation scenarios. To address the cross-domain issue, a straightforward method is to perform token alignment with adversarial training in transformers. However, its performance is often unsatisfactory because the tokens in detection transformers are quite diverse and represent different spatial and semantic information. In this paper, we propose a new method for cross-domain detection transformers called spatial-aware and semantic-aware token alignment (SSTA). Specifically, we take advantage of the characteristics of cross-attention as used in the detection transformer and propose spatial-aware token alignment (SpaTA) and semantic-aware token alignment (SemTA) strategies to guide the token alignment across domains. For spatial-aware token alignment, we extract the information from the cross-attention map (CAM) to align the distribution of tokens according to their attention to object queries. For semantic-aware token alignment, we inject the category information into the cross-attention map and construct domain embedding to guide the learning of a multi-class discriminator to model the category relationship and achieve category-level token alignment during the entire adaptation process. We conduct extensive experiments on several widely-used benchmarks, and the results clearly show the effectiveness of our proposed approach over existing state-of-the-art methods.
更多查看译文
关键词
Object Detection,Domain Adaptation,Detection Transformer
AI 理解论文
溯源树
样例
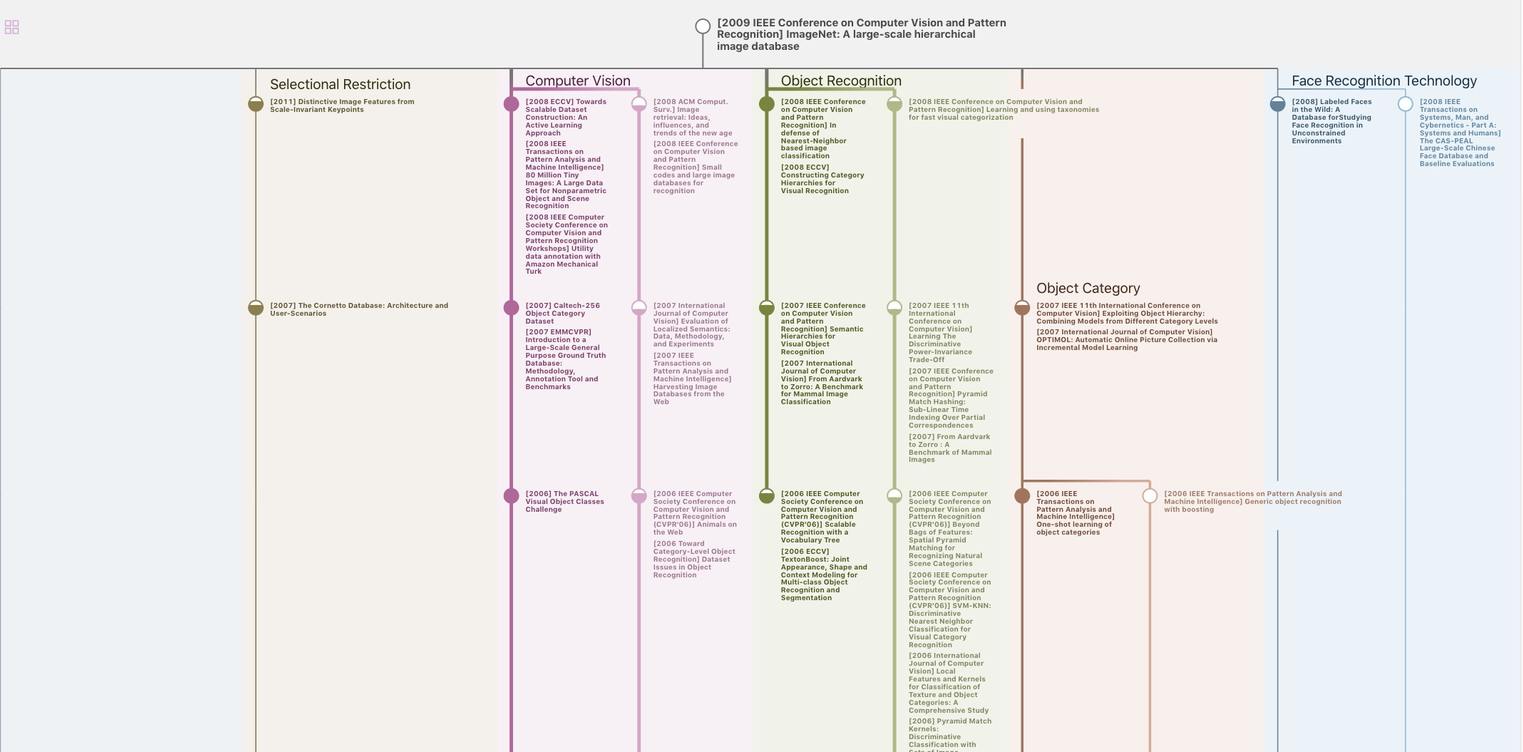
生成溯源树,研究论文发展脉络
Chat Paper
正在生成论文摘要