Interpretable Deep Learning Classifier by Detection of Prototypical Parts on Kidney Stones Images
arxiv(2022)
摘要
Identifying the type of kidney stones can allow urologists to determine their formation cause, improving the early prescription of appropriate treatments to diminish future relapses. However, currently, the associated ex-vivo diagnosis (known as morpho-constitutional analysis, MCA) is time-consuming, expensive, and requires a great deal of experience, as it requires a visual analysis component that is highly operator dependant. Recently, machine learning methods have been developed for in-vivo endoscopic stone recognition. Shallow methods have been demonstrated to be reliable and interpretable but exhibit low accuracy, while deep learning-based methods yield high accuracy but are not explainable. However, high stake decisions require understandable computer-aided diagnosis (CAD) to suggest a course of action based on reasonable evidence, rather than merely prescribe one. Herein, we investigate means for learning part-prototypes (PPs) that enable interpretable models. Our proposal suggests a classification for a kidney stone patch image and provides explanations in a similar way as those used on the MCA method.
更多查看译文
关键词
kidney stones images,deep learning,detection
AI 理解论文
溯源树
样例
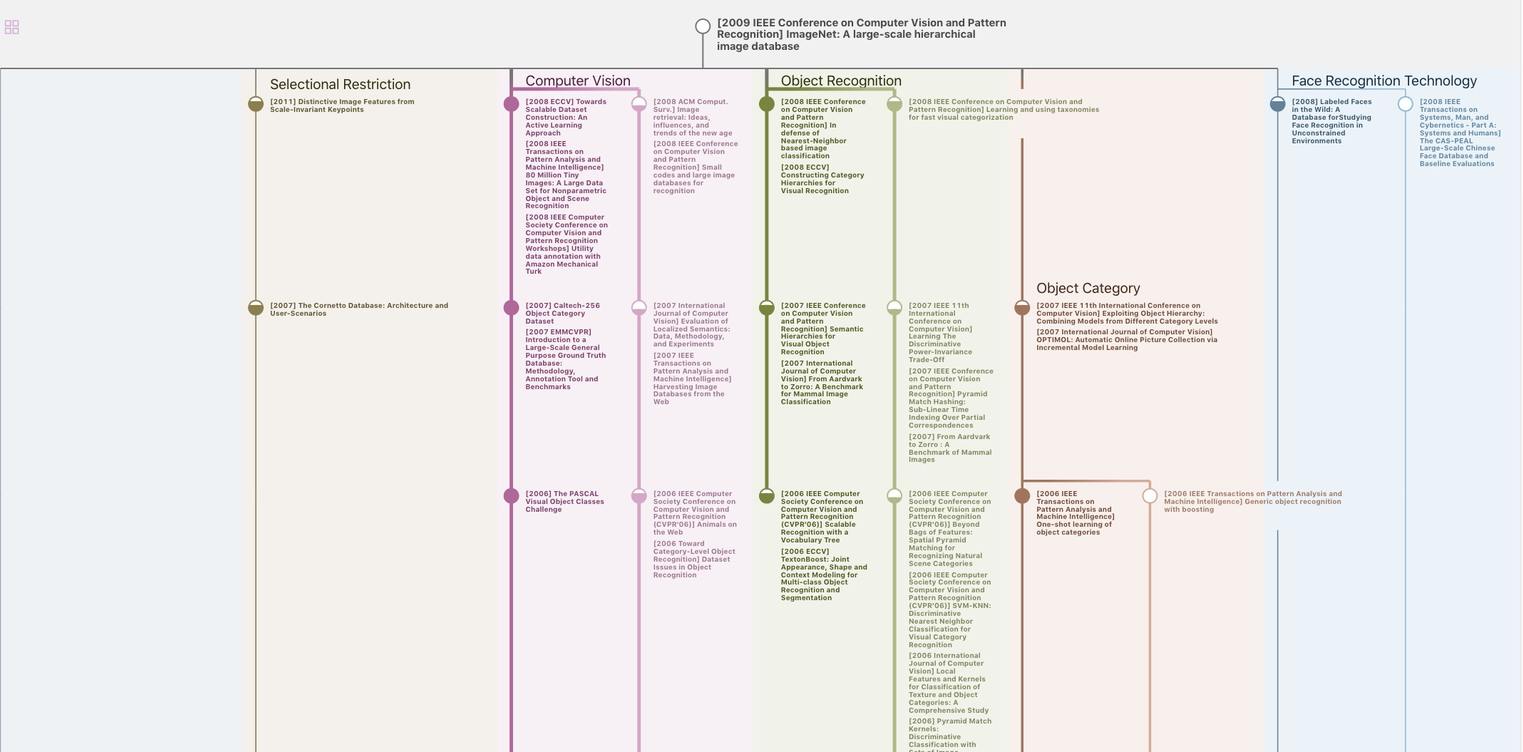
生成溯源树,研究论文发展脉络
Chat Paper
正在生成论文摘要