Differentially Private Shapley Values for Data Evaluation
arxiv(2022)
摘要
The Shapley value has been proposed as a solution to many applications in machine learning, including for equitable valuation of data. Shapley values are computationally expensive and involve the entire dataset. The query for a point's Shapley value can also compromise the statistical privacy of other data points. We observe that in machine learning problems such as empirical risk minimization, and in many learning algorithms (such as those with uniform stability), a diminishing returns property holds, where marginal benefit per data point decreases rapidly with data sample size. Based on this property, we propose a new stratified approximation method called the Layered Shapley Algorithm. We prove that this method operates on small (O(\polylog(n))) random samples of data and small sized ($O(\log n)$) coalitions to achieve the results with guaranteed probabilistic accuracy, and can be modified to incorporate differential privacy. Experimental results show that the algorithm correctly identifies high-value data points that improve validation accuracy, and that the differentially private evaluations preserve approximate ranking of data.
更多查看译文
关键词
private shapley values,data evaluation
AI 理解论文
溯源树
样例
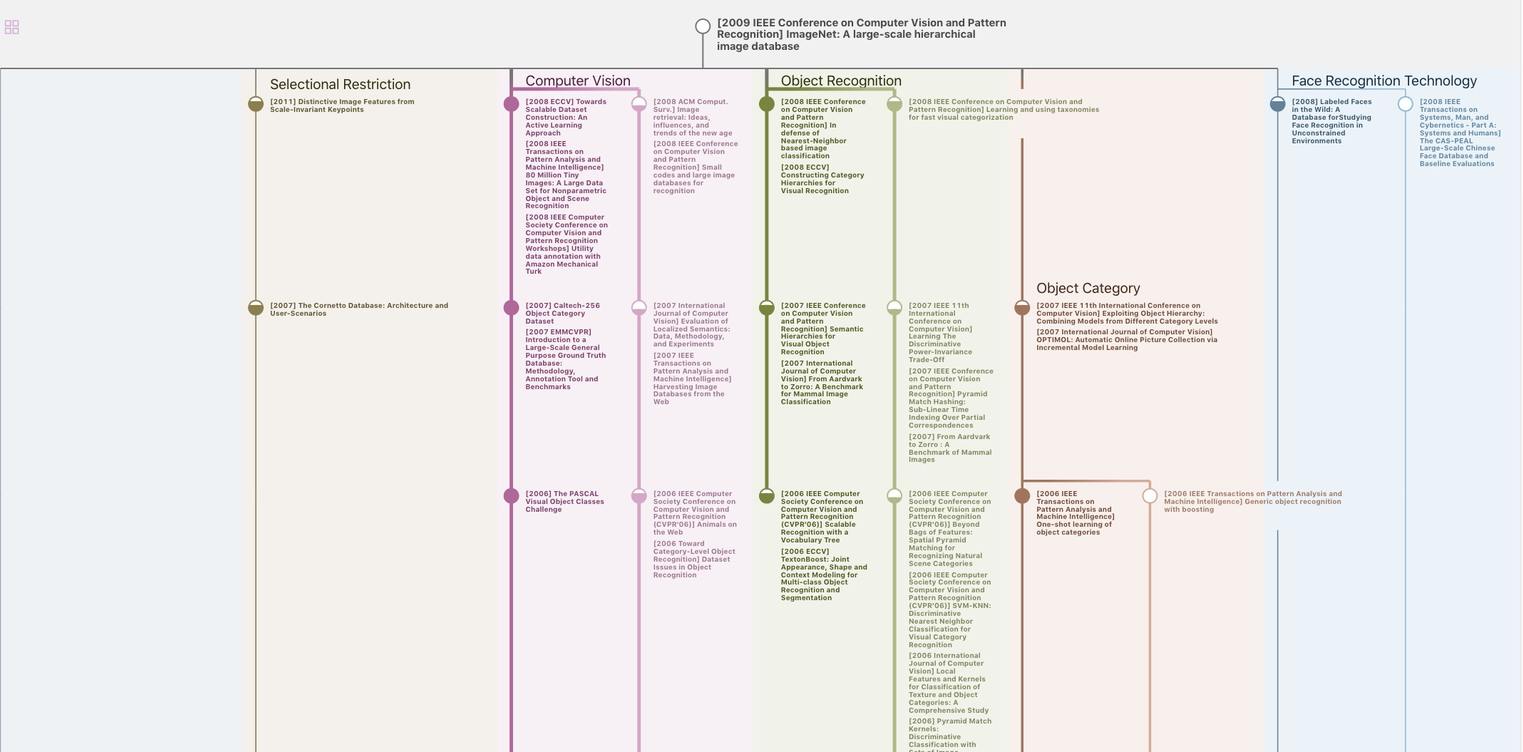
生成溯源树,研究论文发展脉络
Chat Paper
正在生成论文摘要