2D-3D cascade network for glioma segmentation in multisequence MRI images using multiscale information.
Computer methods and programs in biomedicine(2022)
摘要
BACKGROUND AND OBJECTIVE:Glioma segmentation is an important procedure for the treatment plan and follow-up evaluation of patients with glioma. UNet-based networks are widely used in medical image segmentation tasks and have achieved state-of-the-art performance. However, context information along the third dimension is ignored in 2D convolutions, whereas difference between z-axis and in-plane resolutions is large in 3D convolutions. Moreover, an original UNet structure cannot capture fine details because of the reduced resolution of feature maps near bottleneck layers.
METHODS:To address these issues, a novel 2D-3D cascade network with multiscale information module is proposed for the multiclass segmentation of gliomas in multisequence MRI images. First, a 2D network is applied to fully exploit potential intra-slice features. A variational autoencoder module is incorporated into 2D DenseUNet to regularize a shared encoder, extract useful information, and represent glioma heterogeneity. Second, we integrated 3D DenseUNet with the 2D network in cascade mode to extract useful inter-slice features and alleviate the influence of large difference between z-axis and in-plane resolutions. Moreover, a multiscale information module is used in the 2D and 3D networks to further capture the fine details of gliomas. Finally, the whole 2D-3D cascade network is trained in an end-to-end manner, where the intra-slice and inter-slice features are fused and optimized jointly to take full advantage of 3D image information.
RESULTS:Our method is evaluated on publicly available and clinical datasets and achieves competitive performance in these two datasets.
CONCLUSIONS:These results indicate that the proposed method may be a useful tool for glioma segmentation.
更多查看译文
AI 理解论文
溯源树
样例
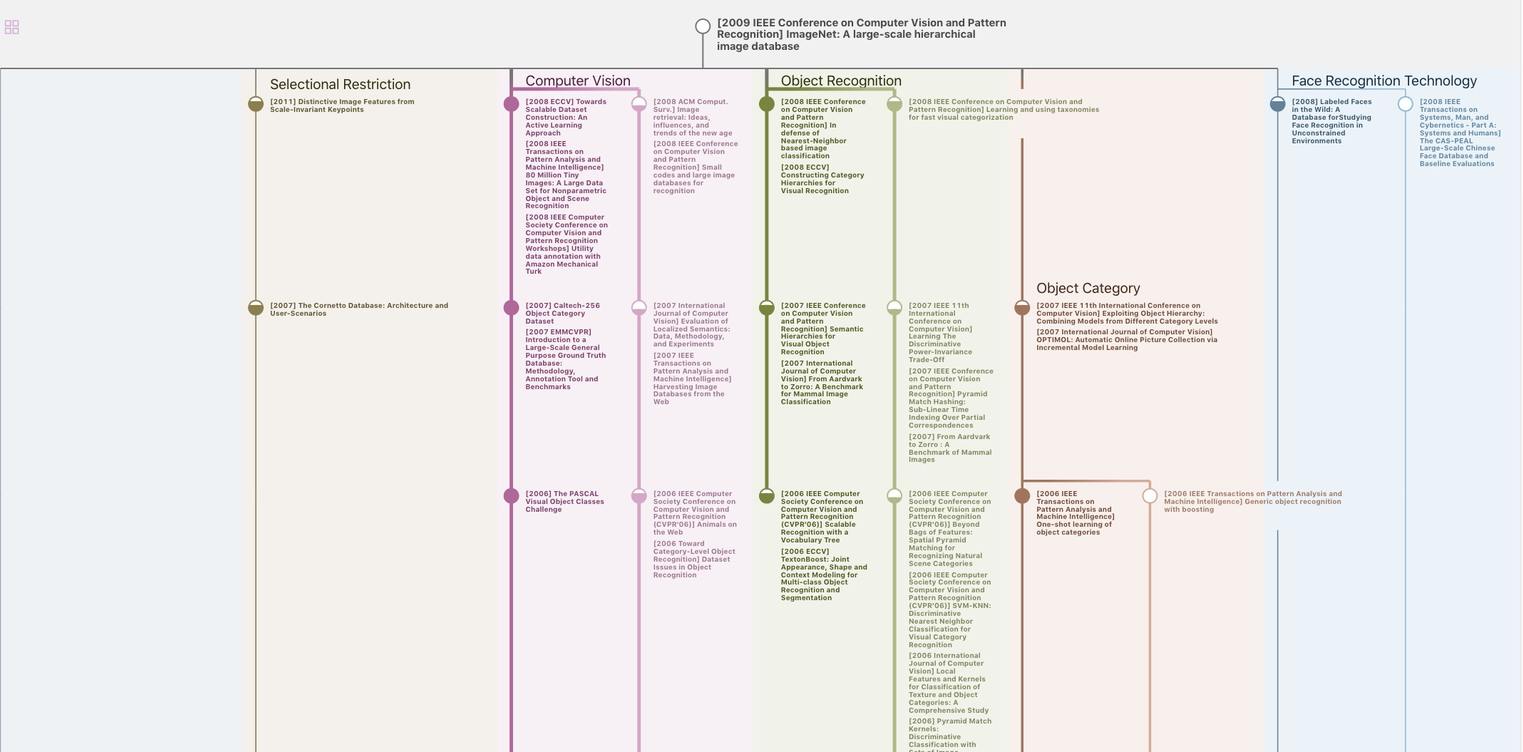
生成溯源树,研究论文发展脉络
Chat Paper
正在生成论文摘要