Multi-view manifold learning of human brain-state trajectories
Nature Computational Science(2023)
摘要
The complexity of the human brain gives the illusion that brain activity is intrinsically high-dimensional. Nonlinear dimensionality-reduction methods such as uniform manifold approximation and t-distributed stochastic neighbor embedding have been used for high-throughput biomedical data. However, they have not been used extensively for brain activity data such as those from functional magnetic resonance imaging (fMRI), primarily due to their inability to maintain dynamic structure. Here we introduce a nonlinear manifold learning method for time-series data—including those from fMRI—called temporal potential of heat-diffusion for affinity-based transition embedding (T-PHATE). In addition to recovering a low-dimensional intrinsic manifold geometry from time-series data, T-PHATE exploits the data’s autocorrelative structure to faithfully denoise and unveil dynamic trajectories. We empirically validate T-PHATE on three fMRI datasets, showing that it greatly improves data visualization, classification, and segmentation of the data relative to several other state-of-the-art dimensionality-reduction benchmarks. These improvements suggest many potential applications of T-PHATE to other high-dimensional datasets of temporally diffuse processes. A manifold learning method called T-PHATE is developed for high-dimensional time-series data. T-PHATE is applied to brain data (functional magnetic resonance imaging) where it faithfully denoises signals and unveils latent brain-state trajectories which correspond with cognitive processing.
更多查看译文
关键词
Cognitive neuroscience,Computational models,Computational neuroscience,Data processing,Computer Science,general
AI 理解论文
溯源树
样例
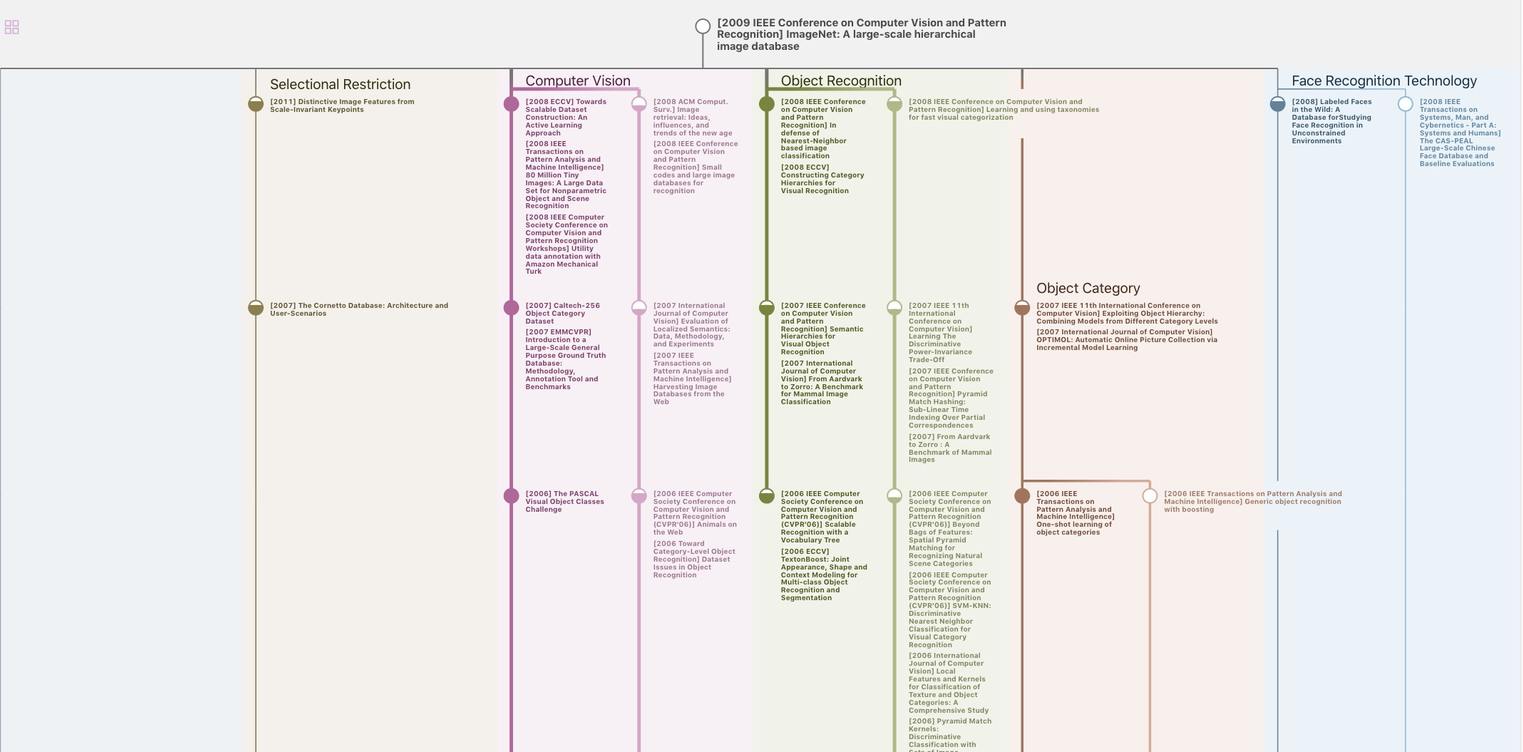
生成溯源树,研究论文发展脉络
Chat Paper
正在生成论文摘要