A machine learning approach for automatic sleep staging using heart rate variability
biorxiv(2022)
摘要
Background There have been recent developments of various machine learning based models to utilize Heart Rate Variability (HRV) indices for sleep staging. Estimation of HRV indices requires the removal of outliers and ectopics from ECG data. HRV indices are essentially determined from overlapping windows on epoched data, giving it a non-temporal architecture. To address this issue we propose a Random Forest Classifier along with sleep epoch index, as a temporal feature, for classification into 5 sleep stages.
Methods Polysomnography data of 30 subjects from the Haaglanden Medisch Centrum sleep staging database were selected. ECG data were corrected for outliers and ectopic beats using linear interrpolation. Time domain, frequency domain and nonlinear domain HRV indices were determined and were fed into the Classifier. Feature selection was performed using the Select From Model algorithm based on the gini importance of the features.
Results The Random Forest Classifier model using HRV indices and epoch index has a validation/test classification accuracy of 79.6±1.6% and 75.9% with Cohen’s kappa of 0.663 and macro F1 score of 0.76.
Conclusion We highlight the importance of correcting outliers and ectopic beats from RR interval data for the accurate estimation of HRV indices. Incorporating the sleep epoch index as a temporal feature improves the performance of the Classifier. Thus our model for classifying sleep stages using HRV data and sleep epoch index may serve as a suitable screening tool for studying sleep architecture.
### Competing Interest Statement
The authors have declared no competing interest.
更多查看译文
关键词
automatic sleep staging,heart rate variability,heart rate,machine learning approach
AI 理解论文
溯源树
样例
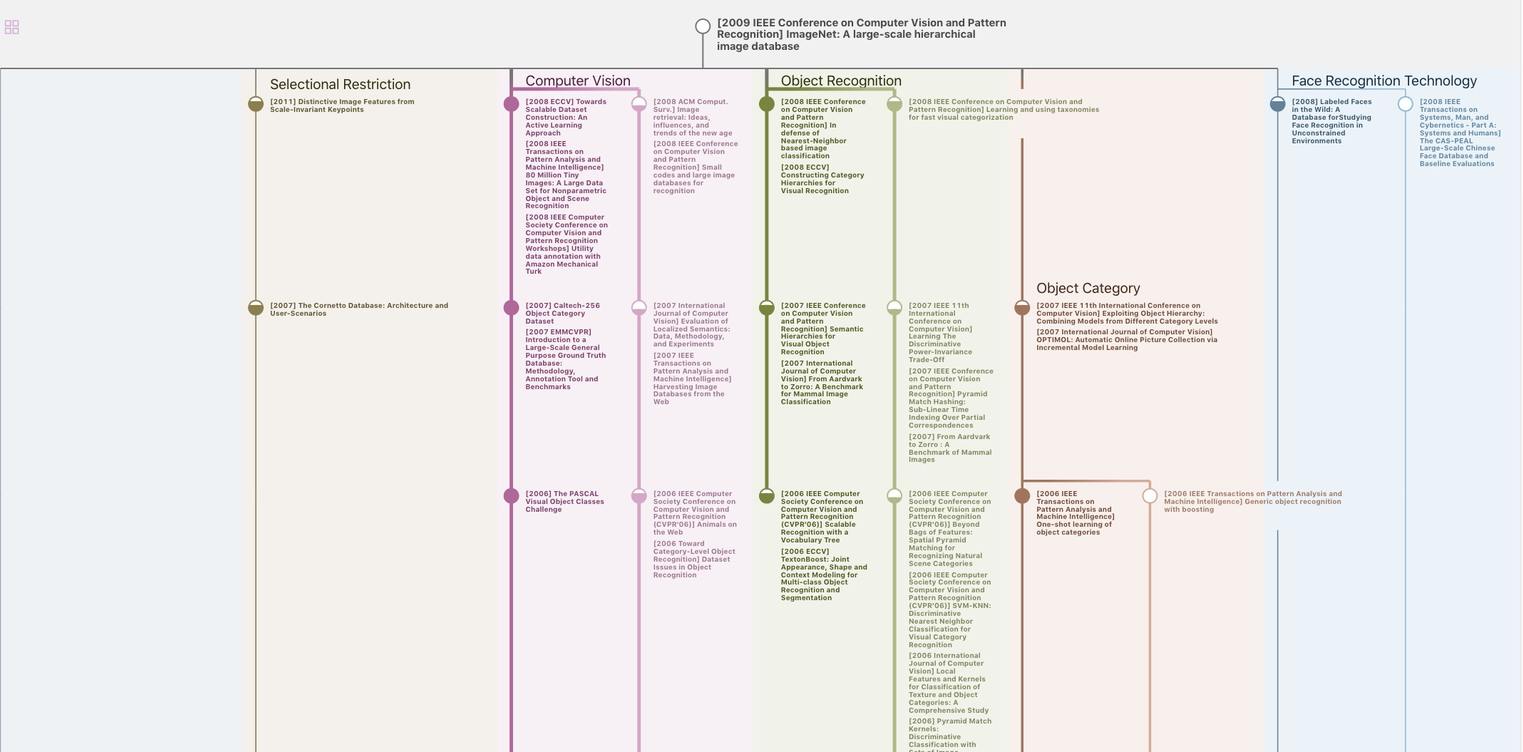
生成溯源树,研究论文发展脉络
Chat Paper
正在生成论文摘要