Effect of 320-row CT reconstruction technology on fractional flow reserve derived from coronary CT angiography based on machine learning: single- versus multiple-cardiac periodic images
QUANTITATIVE IMAGING IN MEDICINE AND SURGERY(2022)
摘要
Background: Fractional flow reserve derived from computed tomography (CT-FFR) can be used to noninvasively evaluate the functions of coronary arteries and has been widely welcomed in the field of cardiovascular research. However, whether different image reconstruction schemes have an effect on CT-FFR analysis through single- and multiple-cardiac periodic images in the same patient has not been investigated. Methods: This study retrospectively enrolled 122 patients who underwent 320-row computed tomography (CT) examination with both single- and multiple-cardiac periodic reconstruction schemes; a total of 366 coronary arteries were analyzed. The lowest CT-FFR values of each vessel and the poststenosis CTFFR values of the lesion-specific coronary artery were measured using the two reconstruction techniques. The Wilcoxon signed-rank test was used to compare differences in CT-FFR values between the two reconstruction techniques. Spearman correlation analysis was performed to determine the relationship between CT-FFR values derived using the two methods. Bland-Altman and intraclass correlation coefficient (ICC) analyses were performed to evaluate the consistency of CT-FFR values. Results: In all blood vessels, the lowest CT-FFR values showed no significant differences between the two reconstruction techniques in the left anterior descending artery (LAD; P=0.65), left circumflex artery (LCx; P=0.46), or right coronary artery (RCA; P=0.22). In blood vessels with atherosclerotic plaques, the poststenosis CT-FFR values (2 cm distal to the maximum stenosis) exhibited no significant differences between the two reconstruction techniques in the LAD (P=0.78), LCx (P=1.00), or RCA (P=1.00). The mean CT-FFR values of single- and multiple-cardiac periodic images showed excellent correlation and minimal bias in all groups. Conclusions: CT-FFR analysis based on an artificial intelligence deep learning neural network is stable and not affected by the type of 320-row CT reconstruction technology.
更多查看译文
关键词
Coronary artery disease (CAD), coronary computed tomography angiography (CCTA), fractional flow reserve (FFR), machine learning (ML)
AI 理解论文
溯源树
样例
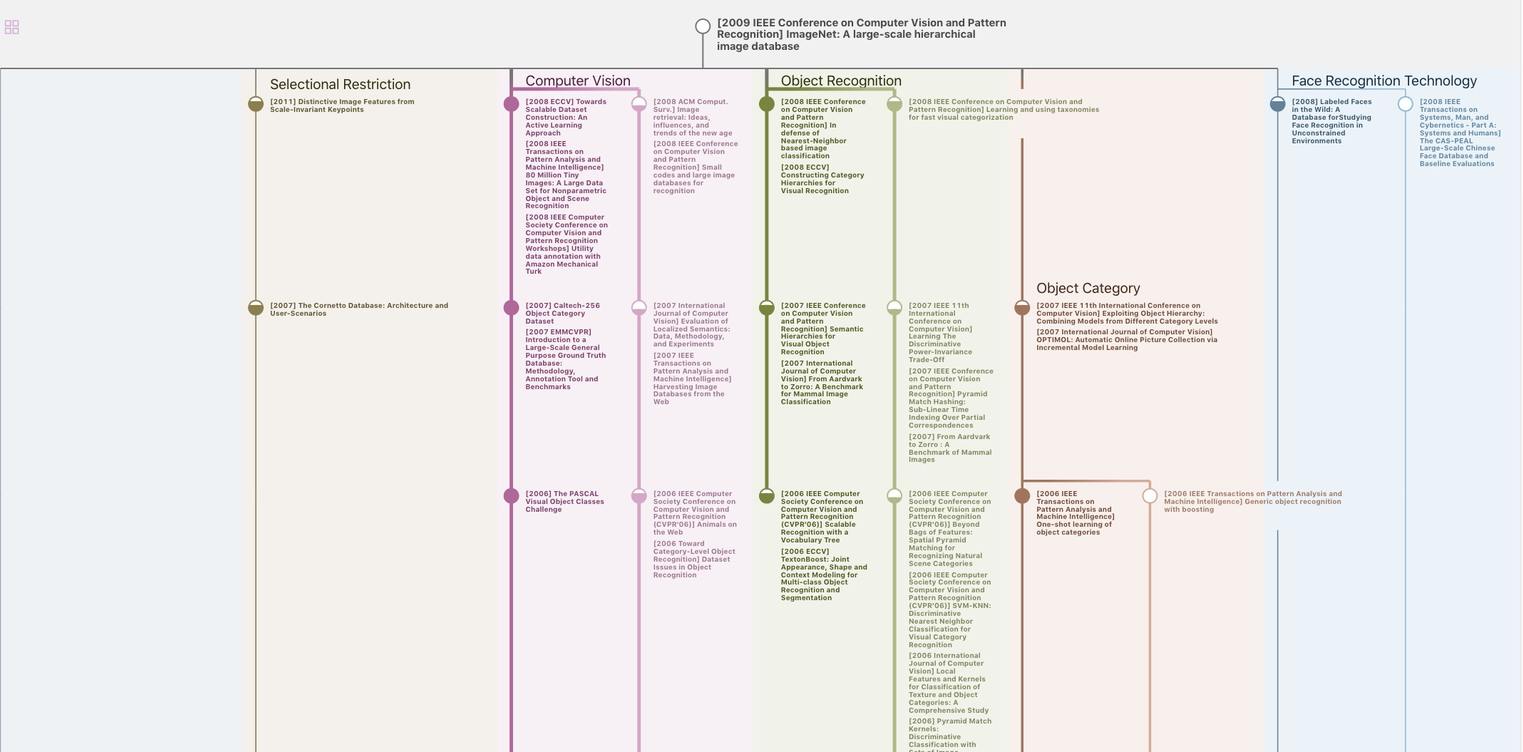
生成溯源树,研究论文发展脉络
Chat Paper
正在生成论文摘要