Completion Time Minimization of Fog-RAN-Assisted Federated Learning With Rate-Splitting Transmission
IEEE Transactions on Vehicular Technology(2022)
摘要
This work studies federated learning (FL) over a fog radio access network, in which multiple internet-of-things (IoT) devices cooperatively learn a shared machine learning model by communicating with a cloud server (CS) through distributed access points (APs). Under the assumption that the fronthaul links connecting APs to CS have finite capacity, a rate-splitting transmission at IoT devices (IDs) is proposed which enables hybrid edge and cloud decoding of split uplink messages. The problem of completion time minimization for FL is tackled by optimizing the rate-splitting transmission and fronthaul quantization strategies along with training hyperparameters such as precision and iteration numbers. Numerical results show that the proposed rate-splitting transmission achieves notable gains over benchmark schemes which rely solely on edge or cloud decoding.
更多查看译文
关键词
Federated learning,fog-RAN,rate splitting,hybrid decoding,completion time minimization
AI 理解论文
溯源树
样例
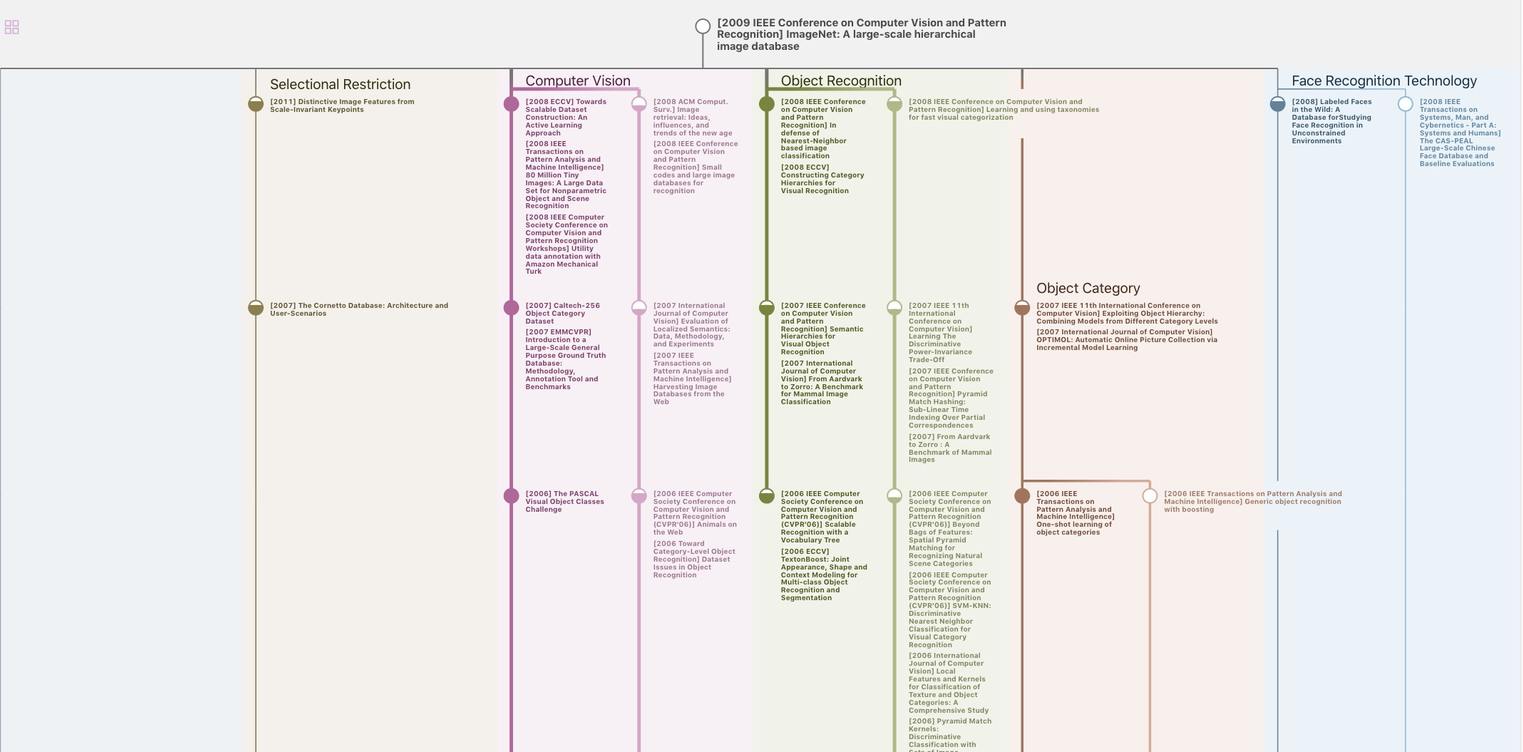
生成溯源树,研究论文发展脉络
Chat Paper
正在生成论文摘要