Constraining Gaussian processes for physics-informed acoustic emission mapping
Mechanical Systems and Signal Processing(2023)
摘要
The automated localisation of damage in structures is a challenging but critical ingredient in the path towards predictive or condition-based maintenance of high value structures. The use of acoustic emission time of arrival mapping is a promising approach to this challenge, but is severely hindered by the need to collect a dense set of artificial acoustic emission measurements across the structure, resulting in a lengthy and often impractical data acquisition process. In this paper, we consider the use of physics-informed Gaussian processes for learning these maps to alleviate this problem. In the approach, the Gaussian process is constrained to the physical domain such that information relating to the geometry and boundary conditions of the structure are embedded directly into the learning process, returning a model that guarantees that any predictions made satisfy physically-consistent behaviour at the boundary. A number of scenarios that arise when training measurement acquisition is limited, including where training data are sparse, and also of limited coverage over the structure of interest. Using a complex plate-like structure as an experimental case study, we show that our approach significantly reduces the burden of data collection, where it is seen that incorporation of boundary condition knowledge significantly improves predictive accuracy as training observations are reduced, particularly when training measurements are not available across all parts of the structure.
更多查看译文
关键词
Physics-informed learning,Damage localisation,Acoustic emission,Constrained Gaussian processes
AI 理解论文
溯源树
样例
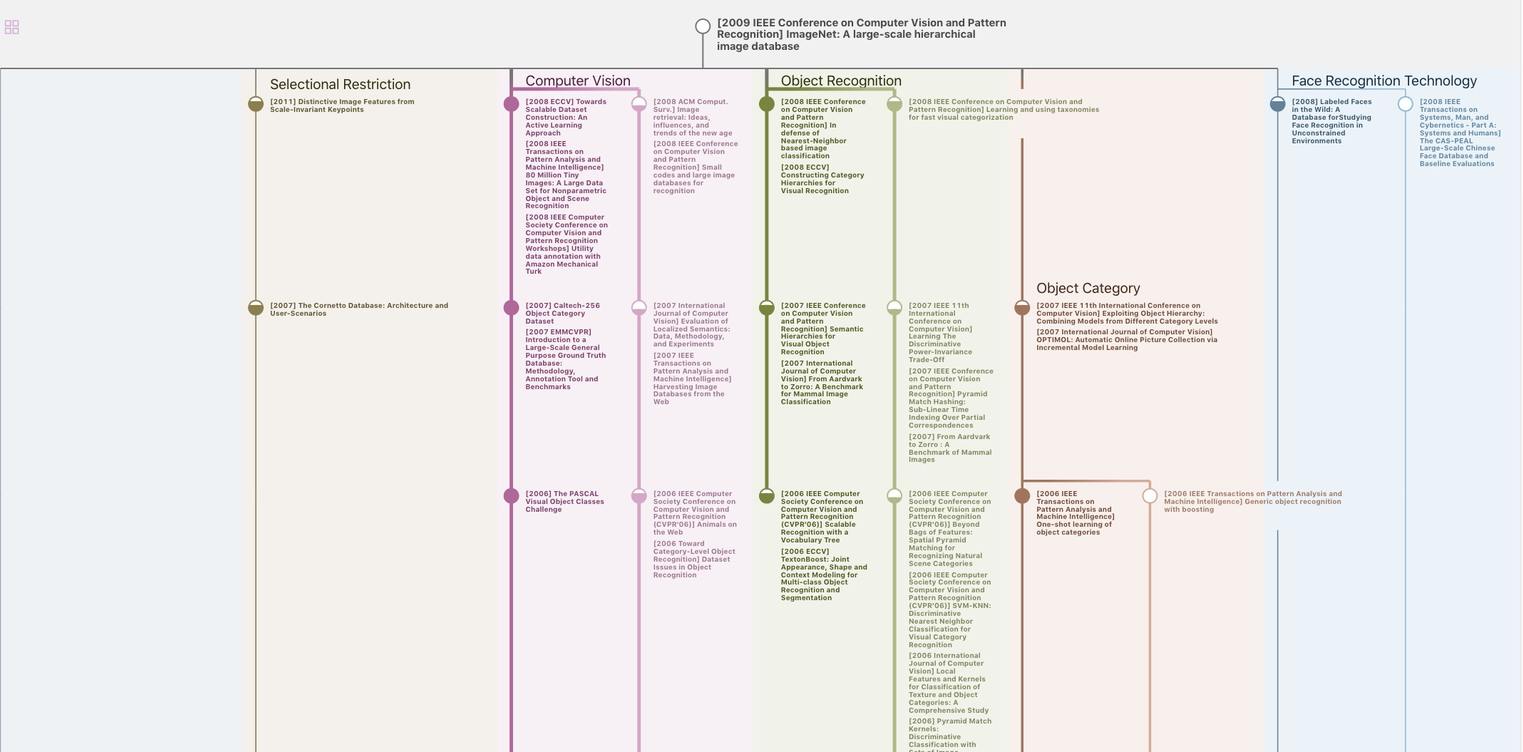
生成溯源树,研究论文发展脉络
Chat Paper
正在生成论文摘要