Contrastive Graph Multimodal Model for Text Classification in Videos
arxiv(2022)
摘要
The extraction of text information in videos serves as a critical step towards semantic understanding of videos. It usually involved in two steps: (1) text recognition and (2) text classification. To localize texts in videos, we can resort to large numbers of text recognition methods based on OCR technology. However, to our knowledge, there is no existing work focused on the second step of video text classification, which will limit the guidance to downstream tasks such as video indexing and browsing. In this paper, we are the first to address this new task of video text classification by fusing multimodal information to deal with the challenging scenario where different types of video texts may be confused with various colors, unknown fonts and complex layouts. In addition, we tailor a specific module called CorrelationNet to reinforce feature representation by explicitly extracting layout information. Furthermore, contrastive learning is utilized to explore inherent connections between samples using plentiful unlabeled videos. Finally, we construct a new well-defined industrial dataset from the news domain, called TI-News, which is dedicated to building and evaluating video text recognition and classification applications. Extensive experiments on TI-News demonstrate the effectiveness of our method.
更多查看译文
关键词
contrastive graph multimodal model,text classification,videos
AI 理解论文
溯源树
样例
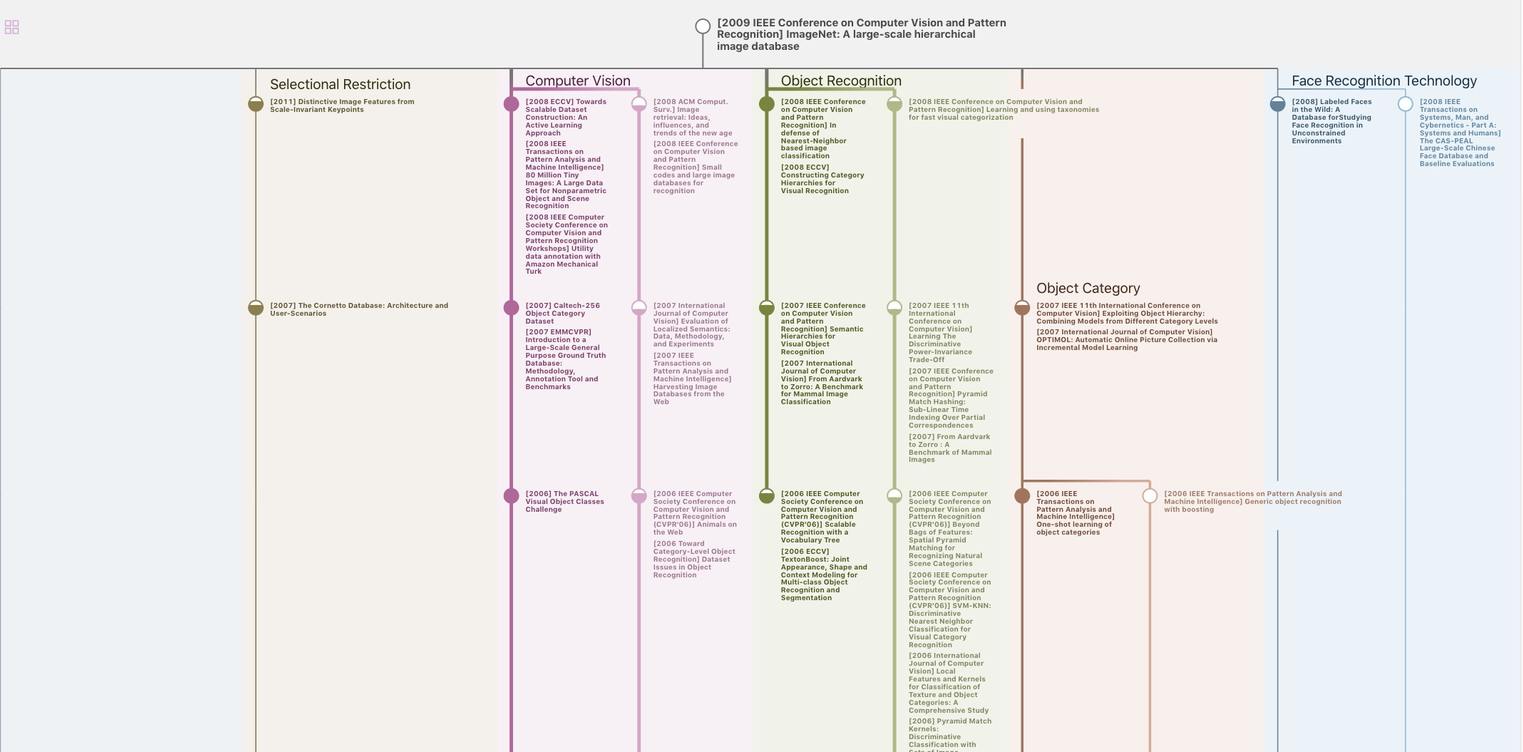
生成溯源树,研究论文发展脉络
Chat Paper
正在生成论文摘要