Persistent homology of coarse-grained state-space networks
arxiv(2023)
摘要
This work is dedicated to the topological analysis of complex transitional networks for dynamic state detection. Transitional networks are formed from time series data and they leverage graph theory tools to reveal information about the underlying dynamic system. However, traditional tools can fail to summarize the complex topology present in such graphs. In this work, we leverage persistent homology from topological data analysis to study the structure of these networks. We contrast dynamic state detection from time series using a coarse-grained state-space network (CGSSN) and topological data analysis (TDA) to two state of the art approaches: ordinal partition networks (OPNs) combined with TDA and the standard application of persistent homology to the time-delay embedding of the signal. We show that the CGSSN captures rich information about the dynamic state of the underlying dynamical system as evidenced by a significant improvement in dynamic state detection and noise robustness in comparison to OPNs. We also show that because the computational time of CGSSN is not linearly dependent on the signal's length, it is more computationally efficient than applying TDA to the time-delay embedding of the time series.
更多查看译文
关键词
persistent homology,networks,coarse-grained,state-space
AI 理解论文
溯源树
样例
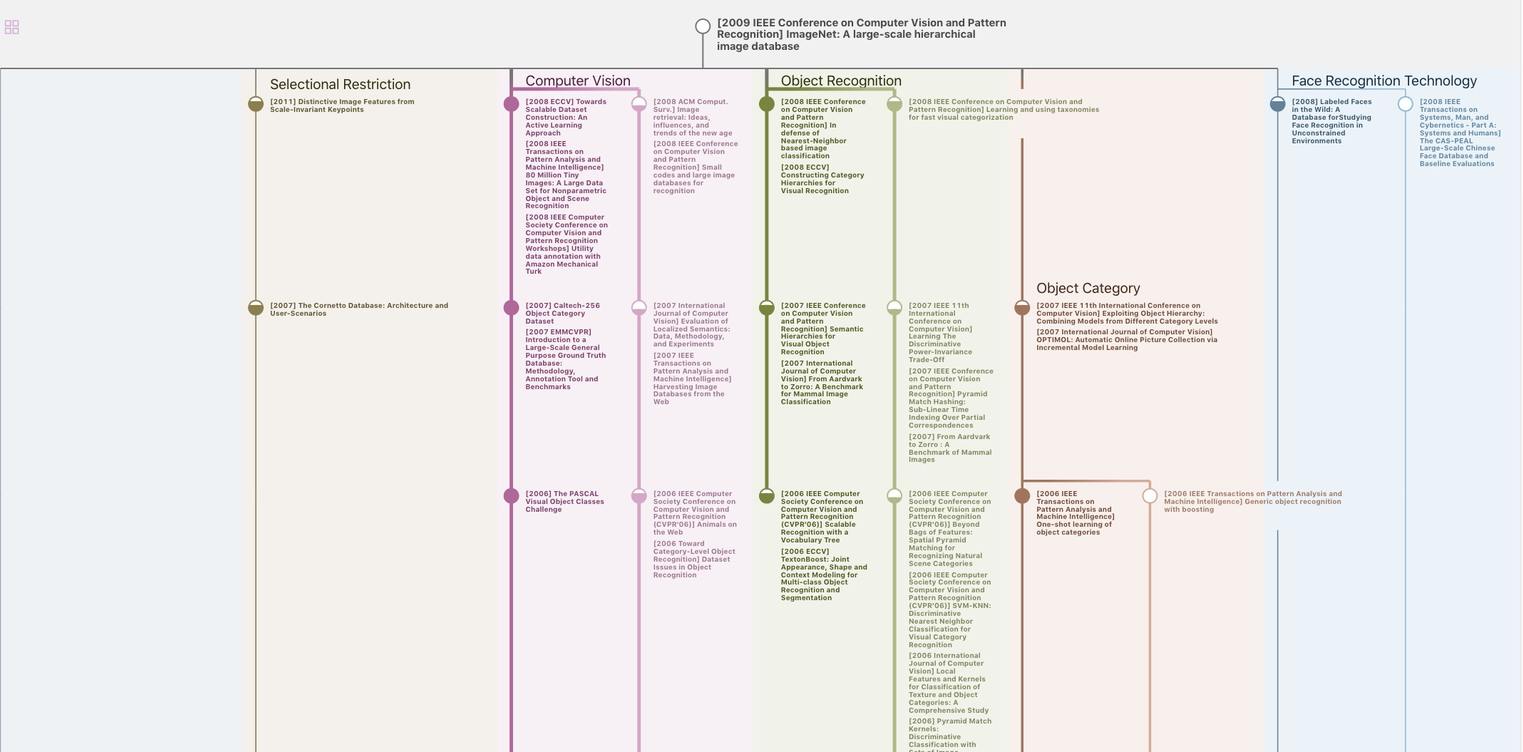
生成溯源树,研究论文发展脉络
Chat Paper
正在生成论文摘要