Robust Adversarial Attacks Detection based on Explainable Deep Reinforcement Learning For UAV Guidance and Planning
arxiv(2023)
摘要
The dangers of adversarial attacks on Uncrewed Aerial Vehicle (UAV) agents operating in public are increasing. Adopting AI-based techniques and, more specifically, Deep Learning (DL) approaches to control and guide these UAVs can be beneficial in terms of performance but can add concerns regarding the safety of those techniques and their vulnerability against adversarial attacks. Confusion in the agent's decision-making process caused by these attacks can seriously affect the safety of the UAV. This paper proposes an innovative approach based on the explainability of DL methods to build an efficient detector that will protect these DL schemes and the UAVs adopting them from attacks. The agent adopts a Deep Reinforcement Learning (DRL) scheme for guidance and planning. The agent is trained with a Deep Deterministic Policy Gradient (DDPG) with Prioritised Experience Replay (PER) DRL scheme that utilises Artificial Potential Field (APF) to improve training times and obstacle avoidance performance. A simulated environment for UAV explainable DRL-based planning and guidance, including obstacles and adversarial attacks, is built. The adversarial attacks are generated by the Basic Iterative Method (BIM) algorithm and reduced obstacle course completion rates from 97\% to 35\%. Two adversarial attack detectors are proposed to counter this reduction. The first one is a Convolutional Neural Network Adversarial Detector (CNN-AD), which achieves accuracy in the detection of 80\%. The second detector utilises a Long Short Term Memory (LSTM) network. It achieves an accuracy of 91\% with faster computing times compared to the CNN-AD, allowing for real-time adversarial detection.
更多查看译文
关键词
Adversarial attacks,adversarial attack detection,AI,autonomous vehicles,DRL,explainability,shapley values,UAV guidance
AI 理解论文
溯源树
样例
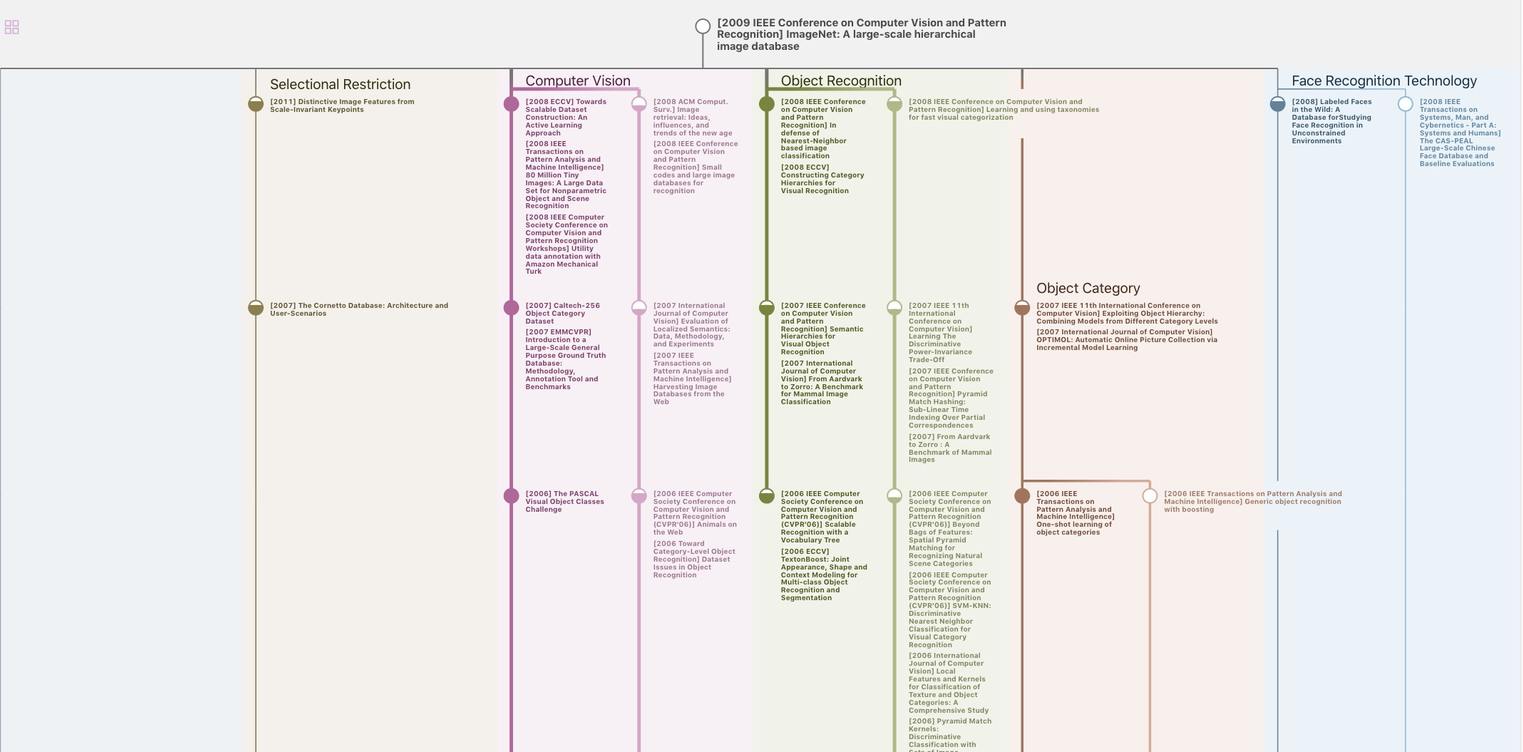
生成溯源树,研究论文发展脉络
Chat Paper
正在生成论文摘要