EvoRator: Prediction of Residue-level Evolutionary Rates from Protein Structures Using Machine Learning
Journal of Molecular Biology(2022)
摘要
•Predicting site-specific evolutionary rates using tools such as ConSurf, is an important bioinformatics application in molecular evolution, structural biology, drug discovery and biochemistry research. Accurate inference requires analyzing a large set of homologous sequences. A gap exists when only few or no homologous proteins can be found, e.g., for orphan proteins.•EvoRator implements a machine-learning based regression to predict site-specific evolutionary rates. In addition to structural features, such as packing density and accessible surface area, it uses a graph-based structural approach to extract informative features.•We evaluated the performance of EvoRator on a previously published benchmark dataset of 213 monomeric enzymes of diverse size, functional, and structural classes. We show that EvoRator is substantially and significantly more accurate than predictions based on single features.•By contrasting site specific conservation scores between EvoRator, which predicts structural constraints, and ConSurf, which predicts both functional and structural constraints, we are able to pinpoint sites that are conserved due to functional rather than structural constraints.
更多查看译文
关键词
protein evolution,machine learning,protein structure,protein function,ConSurf,orphan genes,gapped alignment
AI 理解论文
溯源树
样例
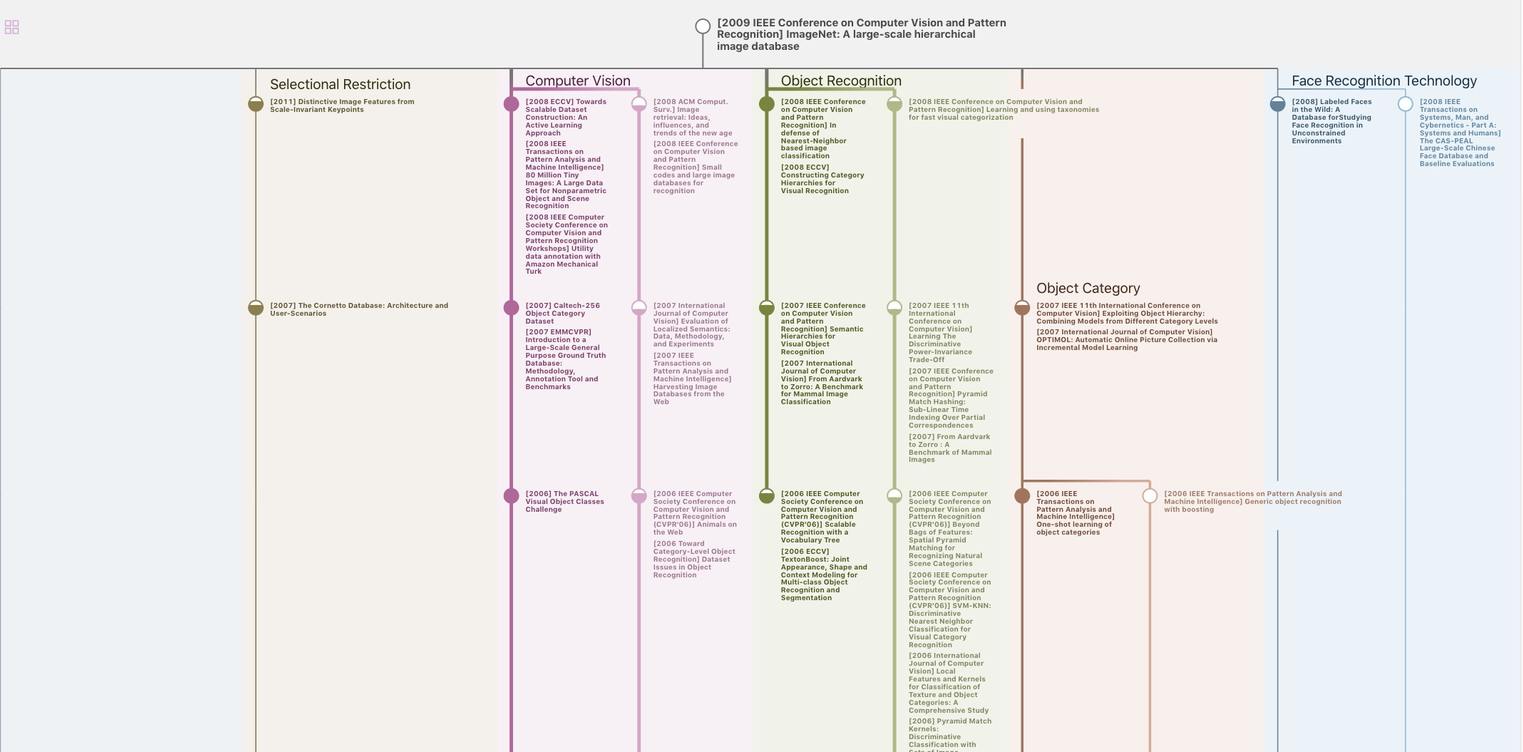
生成溯源树,研究论文发展脉络
Chat Paper
正在生成论文摘要